Neutral pion reconstruction using machine learning in the \minerva experiment at $\langle E_\nu \rangle \sim 6$ GeV
arXiv: High Energy Physics - Experiment(2021)
摘要
This paper presents a novel neutral-pion reconstruction that takes advantage of the machine learning technique of semantic segmentation using MINERvA data collected between 2013-2017, with an average neutrino energy of $6$ GeV. Semantic segmentation improves the purity of neutral pion reconstruction from two gammas from 71\% to 89\% and improves the efficiency of the reconstruction by approximately 40\%. We demonstrate our method in a charged current neutral pion production analysis where a single neutral pion is reconstructed. This technique is applicable to modern tracking calorimeters, such as the new generation of liquid-argon time projection chambers, exposed to neutrino beams with $\langle E_\nu \rangle$ between 1-10 GeV. In such experiments it can facilitate the identification of ionization hits which are associated with electromagnetic showers, thereby enabling improved reconstruction of charged-current $\nu_e$ events arising from $\nu_{\mu} \rightarrow \nu_{e}$ appearance.
更多查看译文
AI 理解论文
溯源树
样例
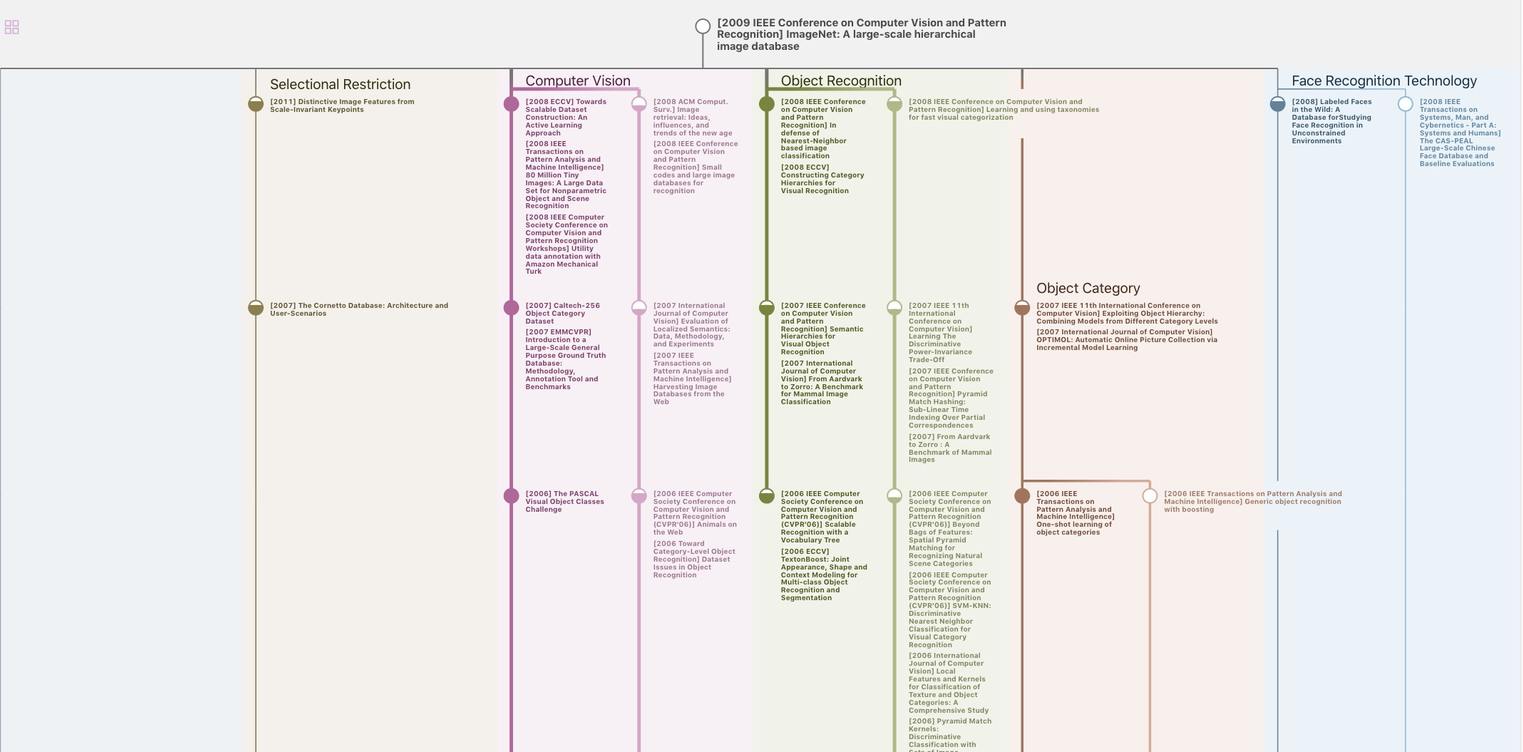
生成溯源树,研究论文发展脉络
Chat Paper
正在生成论文摘要