Effective Utilization of Whitening for Person Identification
Social Science Research Network(2019)
摘要
The growth of importance of Person Identification(PI) is so drastic these days, since it helps support the growth of many other fields like surveillance, robotics etc. Computer Vision Enthusiasts are working on making this robust to various factors such as noise, scale, pose, intensity, illumination, translation, expression and many more. Whitening is a pre-processing technique which makes an image robust to noise, intensity and illumination. There are many feature detectors such as FLANN matching algorithms, which use relatively lesser resources, but are not robust to one or more of the above mentioned problems. However, in general whitening is usually used with machine learning algorithms, which are already robust to these problems. This paper hence compares results obtained using SVD whitening and ZCA whitening to without pre-processing on four distinct pipelines. The first two pipelines use SIFT extracted keypoints and SURF extracted keypoints matched by KD-Tree FLANN matcher and classified using confidence probability matching. These pipelines as we know are not robust to the problems solved by whitening. They are implemented on ORL, grimace and faces 95 database. The third and fourth pipeline includes machine learning algorithms to classify the features extracted by Spatial Pyramid Matching and Earth Mover’s Distance on Dense SIFT keypoints. They were implemented on ORL, faces 95 and FEI database. Results show that SVD whitening and ZCA whitening increases the accuracy more when FLANN matcher is used than when machine learning algorithms are used to classify the images. In both cases, we encounter a scenario where the accuracy is slightly lesser than the accuracy obtained without whitening. However, we find that the decrease in accuracy is more when machine learning algorithms are used than when FLANN matchers are used.
更多查看译文
AI 理解论文
溯源树
样例
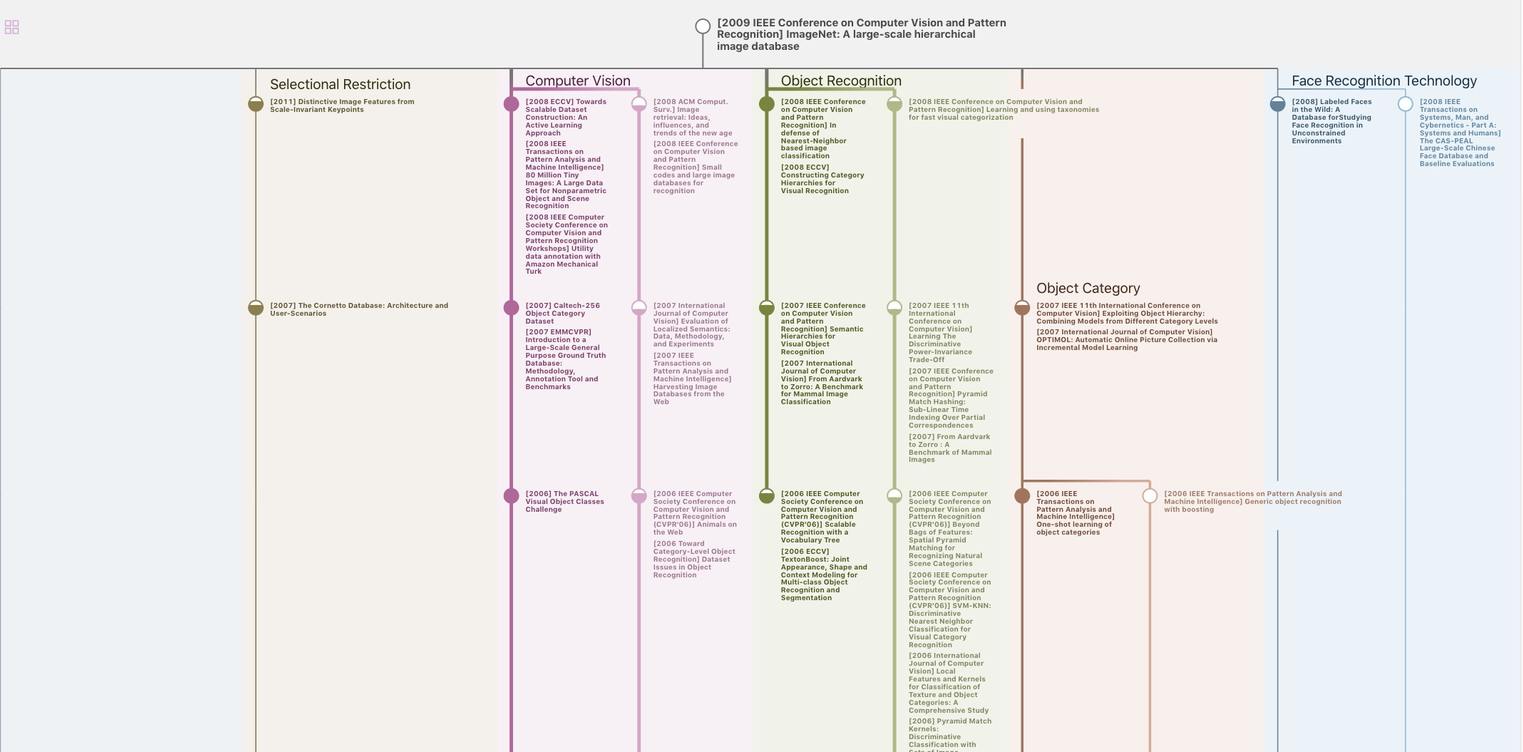
生成溯源树,研究论文发展脉络
Chat Paper
正在生成论文摘要