Color Enhanced Cross Correlation Net for Image Sentiment Analysis
IEEE TRANSACTIONS ON MULTIMEDIA(2024)
摘要
Automatic analysis of image sentiment has gained considerable attention with the increasing throughput of user-generated visual contents online. Recently, researchers generally tend to design different Convolutional Neural Networks (CNNs) to extract image content features for sentiment analysis. However, they underestimated the importance of image color, which has been proved very crucial for image sentiment expressing by psychology and art theory. Moreover, we further observe that the coordination of content and color is the main form of image sentiment expressing. Different combinations of content and color could express extremely different sentiments. To that end, in this paper, we propose a Color Enhanced Cross Correlation Net (CECCN), a novel architecture for image sentiment analysis that not only leverages contents and colors simultaneously, but also takes their correlations into consideration. Specifically, we first use a pre-trained CNN to extract content features and color moment to collect color features from multiple color spaces. Then, we propose a novel Cross Correlation (CC) method to model the correlations between content features and color features with attention mechanism and sequence convolution, in which sentiment expressing of content and color can be enhanced by each other. Finally, we integrate these two types of information for better image sentiment analysis. Extensive experiments on two popular and well-studied benchmark datasets demonstrate the superiority and rationality of our proposed CECCN.
更多查看译文
关键词
Image color analysis,Sentiment analysis,Feature extraction,Correlation,Visualization,Brain modeling,Analytical models,Image sentiment analysis,neural network,cross correlation,feature representation
AI 理解论文
溯源树
样例
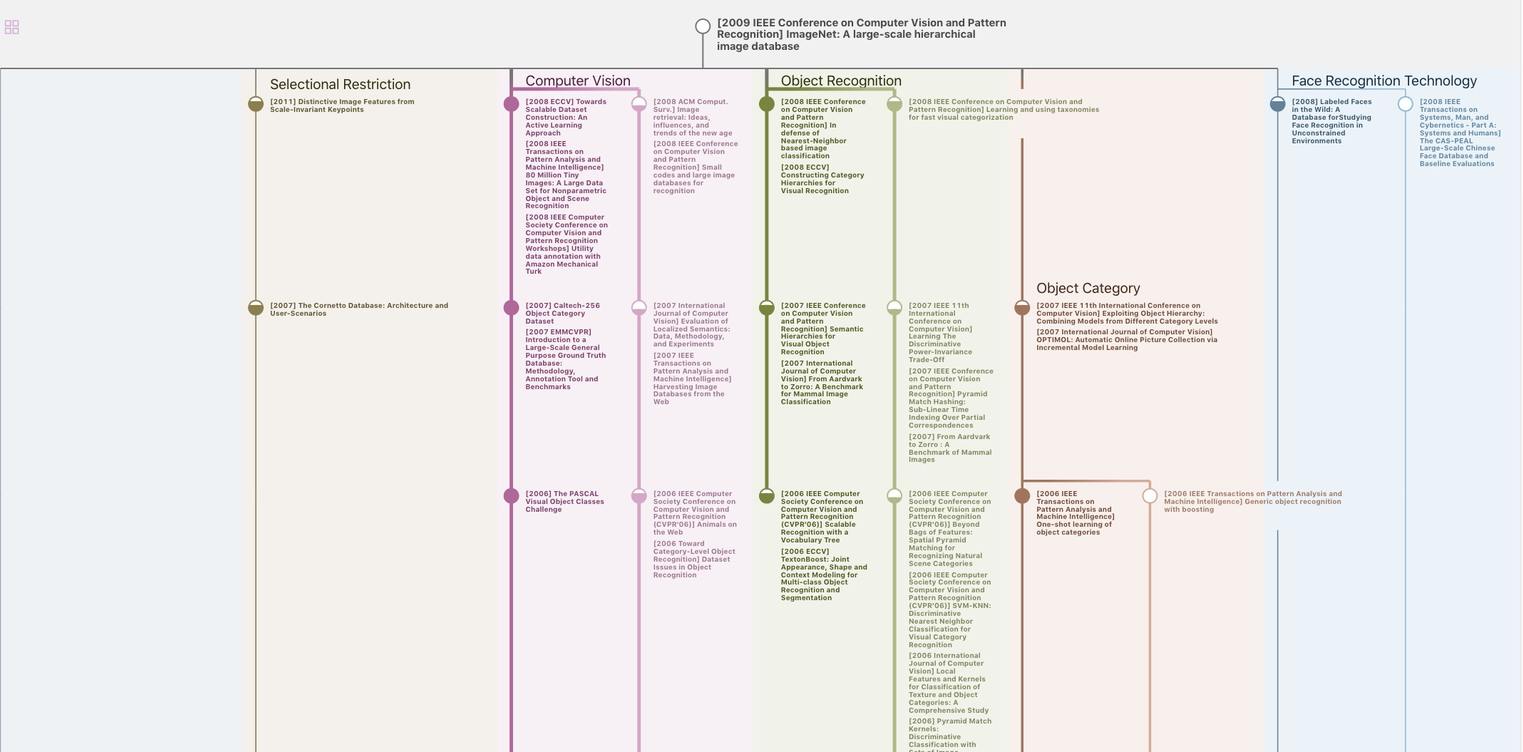
生成溯源树,研究论文发展脉络
Chat Paper
正在生成论文摘要