Spatial-Temporal Chebyshev Graph Neural Network for Traffic Flow Prediction in IoT-Based ITS
IEEE Internet of Things Journal(2022)
摘要
As one of the most widely used applications of the Internet of Things (IoT), intelligent transportation system (ITS) is of great significance for urban traffic planning, traffic control, and traffic guidance. However, widespread traffic congestion occurs with the increased number of vehicles. The traffic flow prediction is a good idea for traffic congestion. Therefore, many schemes have been proposed for accurate and real-time traffic flow prediction, but there still exist many issues, including low accuracy, weak adaptability and inferior real-time. Meanwhile, the complex spatial and temporal dependencies in traffic flow are still challenging. To address the above issues, we propose a novel spatial-temporal Chebyshev graph neural network model (ST-ChebNet) for traffic flow prediction to capture the spatial-temporal features, which can ensure accurate traffic flow prediction. Concretely, we first add a fully connected layer to fuse the features of traffic data into a new feature to generate a matrix, and then the long short-term memory (LSTM) model is adopted to learn traffic state changes for capturing the temporal dependencies. Then, we use the Chebyshev graph neural network (ChebNet) to learn the complex topological structures in the traffic network for capturing the spatial dependencies. Eventually, the spatial features and the temporal features are fused to guarantee the traffic flow prediction. The experiments show that ST-ChebNet can make accurate and real-time traffic flow prediction compared with other eight baseline methods on real-world traffic data sets PeMS.
更多查看译文
关键词
Graph neural network (GNN),intelligent transportation system (ITS),long short-term memory (LSTM),spatial-temporal graph data,traffic flow prediction
AI 理解论文
溯源树
样例
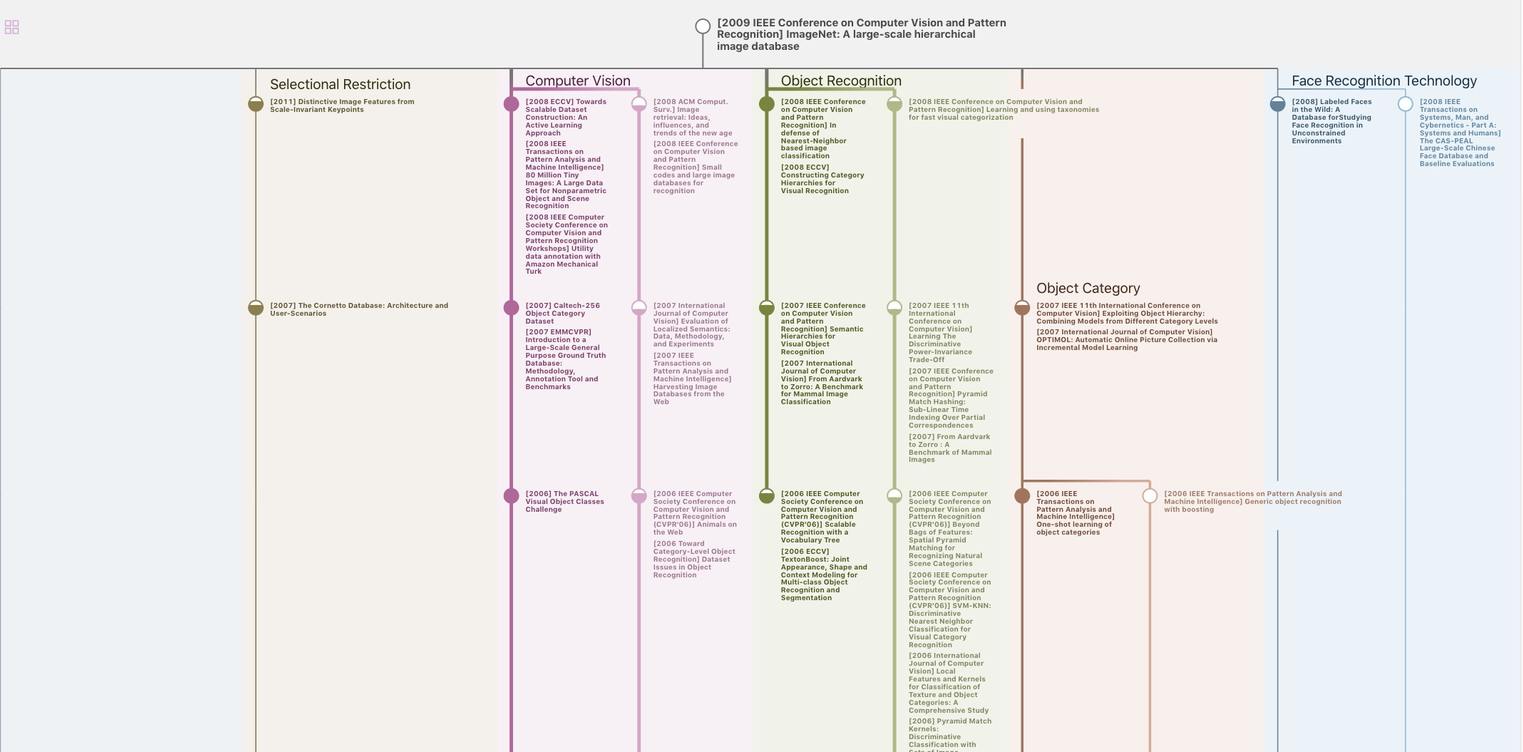
生成溯源树,研究论文发展脉络
Chat Paper
正在生成论文摘要