Probabilistic Reward-Based Reinforcement Learning for Multi-Agent Pursuit and Evasion
chinese control and decision conference(2021)
摘要
The reinforcement learning is studied to solve the problem of multi-agent pursuit and evasion games in this article. The main problem of current reinforcement learning for multi-agents is the low learning efficiency of agents. An important factor leading to this problem is that the delay of the Q function is related to the environment changing. To solve this problem, a probabilistic distribution reward value is used to replace the Q function in the multi-agent depth deterministic policy gradient framework (hereinafter referred to as MADDPG). The distribution Bellman equation is proved to be convergent, and can be brought into the framework of reinforcement learning algorithm. The probabilistic distribution reward value is updated in the algorithm, so that the reward value can be more adaptive to the complex environment. In the same time, eliminating the delay of rewards improves the efficiency of the strategy and obtains a better pursuit-evasion results. The final simulation and experiment show that the multi-agent algorithm with distribution rewards achieves better results under the setting environment.
更多查看译文
关键词
Reinforcement learning,Multi-agent,Pursuit-evasion,Probabilistic reward
AI 理解论文
溯源树
样例
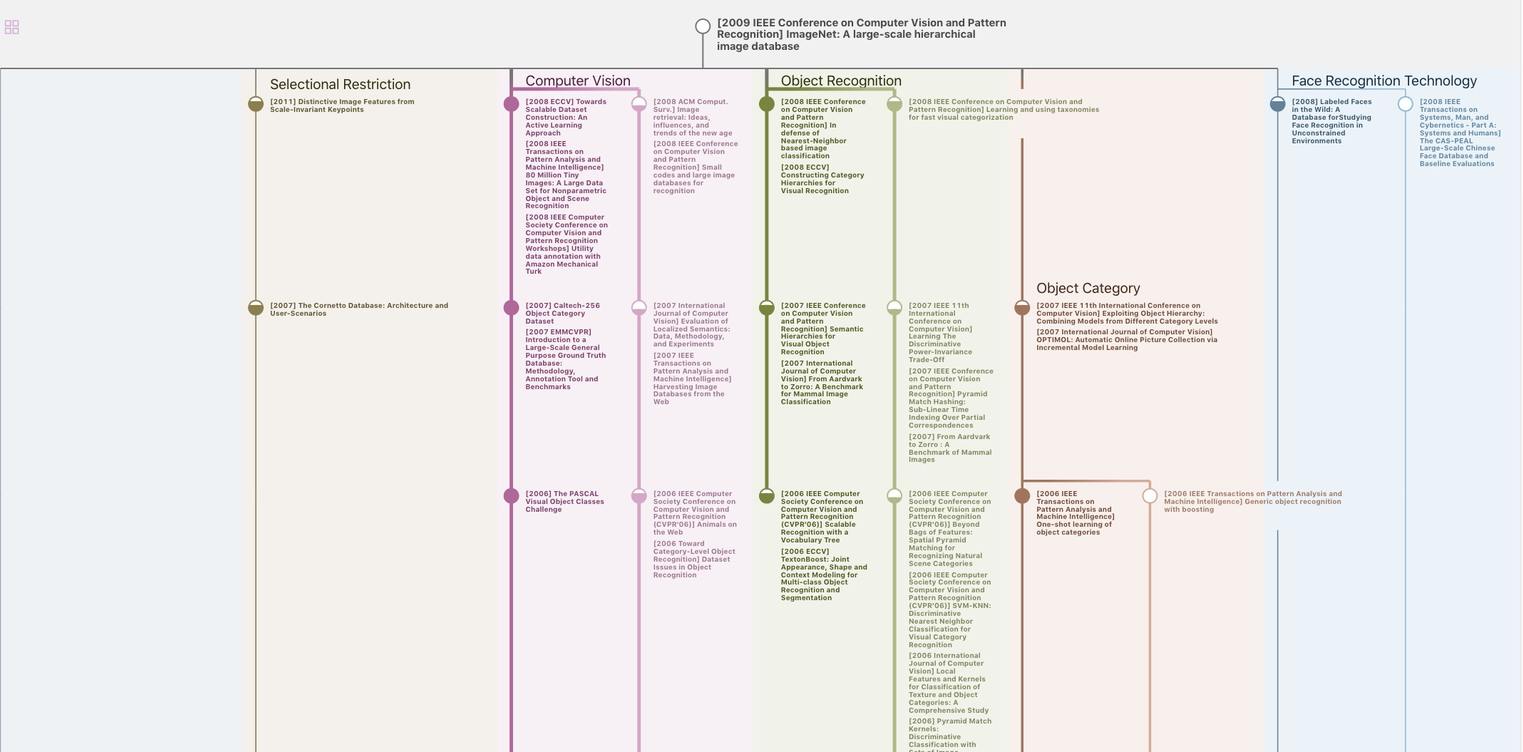
生成溯源树,研究论文发展脉络
Chat Paper
正在生成论文摘要