Quantum Circuit Learning with Parameterized Boson Sampling
IEEE Transactions on Knowledge and Data Engineering(2021)
摘要
A quantum circuit learning approach is studied to carry out the fast-fitting of Gaussian functions. First, a parameterized structure is designed for quantum circuits based on the boson sampling model. And then, the training procedure of exploiting gradient-based optimizations is presented to iteratively update the gradient of the loss function concerning circuit parameters. For efficiency, two kinds of circuit loss, the kernel maximum mean discrepancy and the mean absolute error, are used in the training procedure, which are both competent to achieve quantum circuit learning well. It is significant that the two circuit losses assist in reducing the variance to
$2.54 \times 10^{-6}$
and
$6.91 \times 10^{-6}$
, respectively. Finally, a kind of quantum circuit fixed structure is developed with the boson sampling model that can decrease the model complexity as the circuit depth
$d$
grows. Sets of experiments have been conducted to evaluate the proposed quantum circuit learning scheme, and demonstrate that our parameterized approach is efficient and promising, and it is worth looking forward to solving practical application problems with quantum computers since valid quantum circuits for Gaussian function fast-fitting can be designed indeed.
更多查看译文
关键词
Quantum computing,hybrid quantum-classical approach,quantum circuit learning,boson sampling,parameterized boson sampling,parameterized quantum circuit,gaussian function fitting
AI 理解论文
溯源树
样例
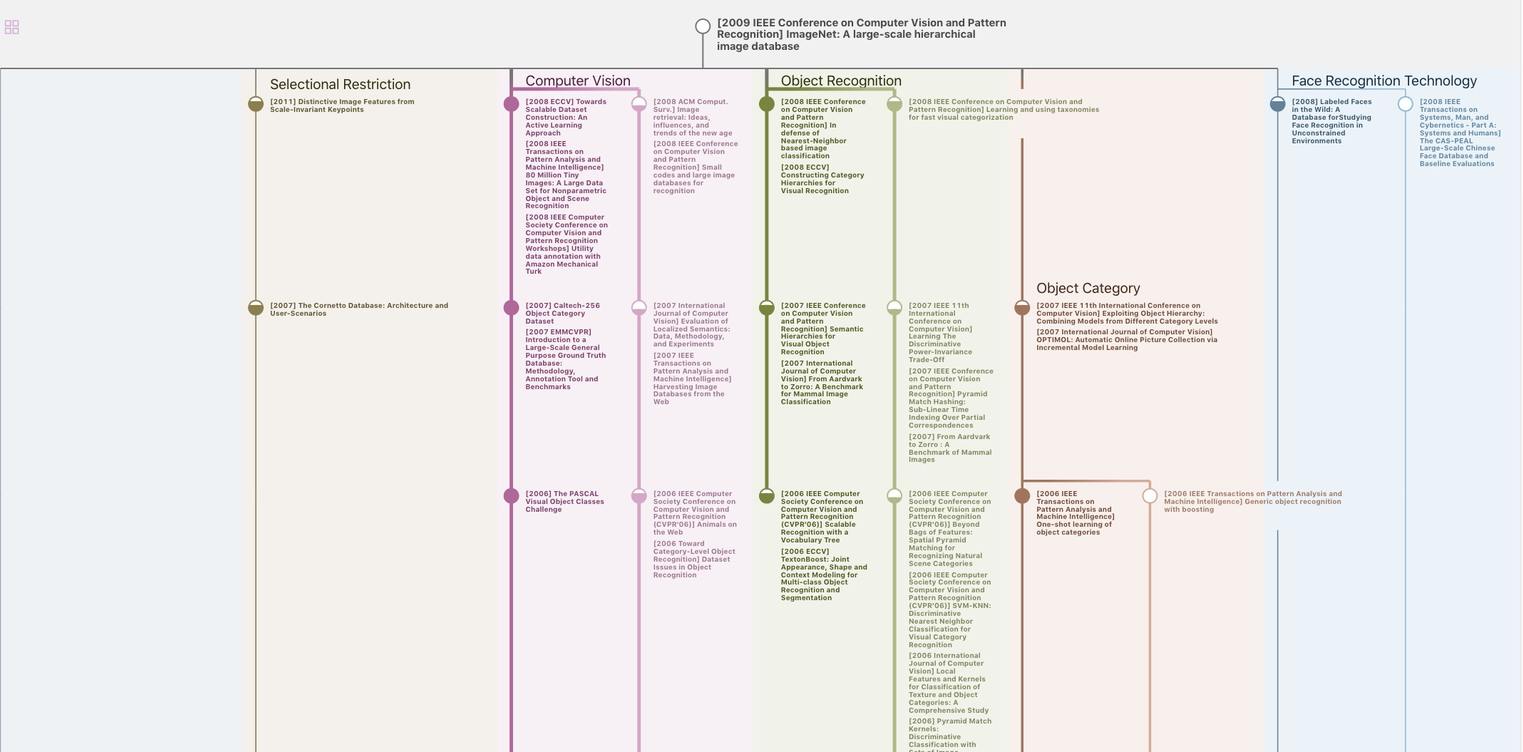
生成溯源树,研究论文发展脉络
Chat Paper
正在生成论文摘要