Improving phenology predictions for sparsely observed species through fusion of botanical collections and citizen-science
Climate Change Ecology(2021)
摘要
Describing patterns of plant phenology through models has been critical for quantifying species responses to climate change and forecasting future vegetation impacts. However, many species remain unincluded in large analyses because they are poorly represented in the large public or citizen science datasets that form the foundation of these efforts. Botanical living collections are often key resources that facilitate study of rare and sparsely observed species, but alone are insufficient to predict species phenology throughout their observed ranges. We investigate whether predictions for rare and data-poor species observed at a single site can be improved by leveraging observations of similar taxa observed at multiple locations. We combined observations of oak (Quercus) budburst and leaf out from one botanical garden with a subset of congeneric species observed in the USA-NPN citizen science dataset using Bayesian hierarchical modeling. We show that including USA-NPN observations into a simple thermal time model of budburst and leaf out did not reduce geographic bias in model predictions over models parameterized only with single-site observations. However, using USA-NPN data to add non-taxonomic spatial covariates to the thermal time model improved model performance for all species, including those only observed at a single site. Living collections at botanical gardens provide valuable opportunities to observe rare or understudied species, but are limited in geographic scope. National-scale citizen science observations that capture the spatial variability of related or ecologically similar taxa can be combined with living collections data to improve predictions of species of conservation concern across their native range.
更多查看译文
关键词
Ecological modeling,Spatial variability,Bayesian modeling,Climate change,Rare species,Vulnerability
AI 理解论文
溯源树
样例
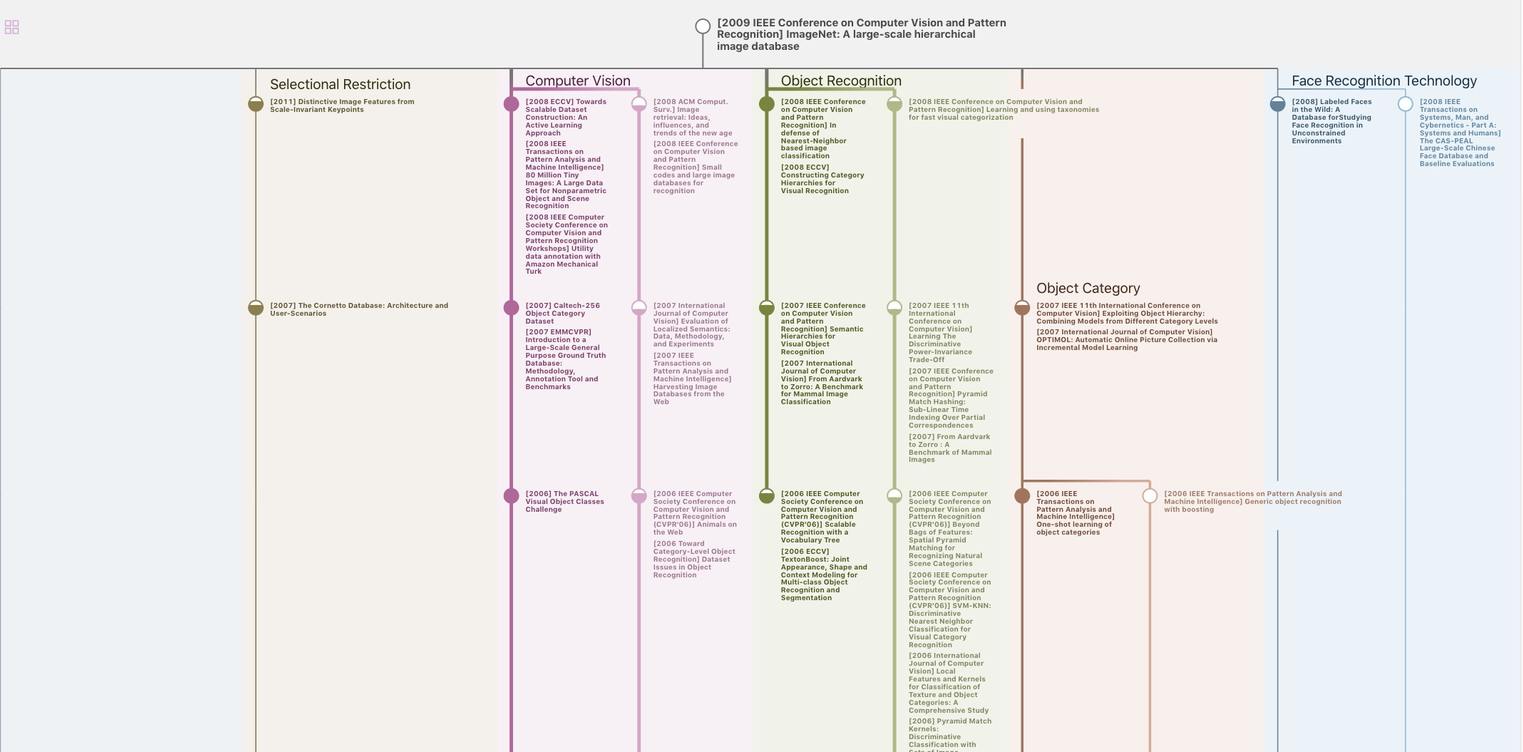
生成溯源树,研究论文发展脉络
Chat Paper
正在生成论文摘要