A Bayesian Alternative to Synthetic Control for Comparative Case Studies
Political Analysis(2022)
摘要
This paper proposes a Bayesian alternative to the synthetic control method for comparative case studies with a single or multiple treated units. We adopt a Bayesian posterior predictive approach to Rubin's causal model, which allows researchers to make inferences about both individual and average treatment effects on treated observations based on the empirical posterior distributions of their counterfactuals. The prediction model we develop is a dynamic multilevel model with a latent factor term to correct biases induced by unit-specific time trends. It also considers heterogeneous and dynamic relationships between covariates and the outcome, thus improving precision of the causal estimates. To reduce model dependency, we adopt a Bayesian shrinkage method for model searching and factor selection. Monte Carlo exercises demonstrate that our method produces more precise causal estimates than existing approaches and achieves correct frequentist coverage rates even when sample sizes are small and rich heterogeneities are present in data. We illustrate the method with two empirical examples from political economy.
更多查看译文
关键词
synthetic control,comparative case studies,panel data,TSCS data,causal inference,Bayesian statistics,stochastic model search,latent factor model
AI 理解论文
溯源树
样例
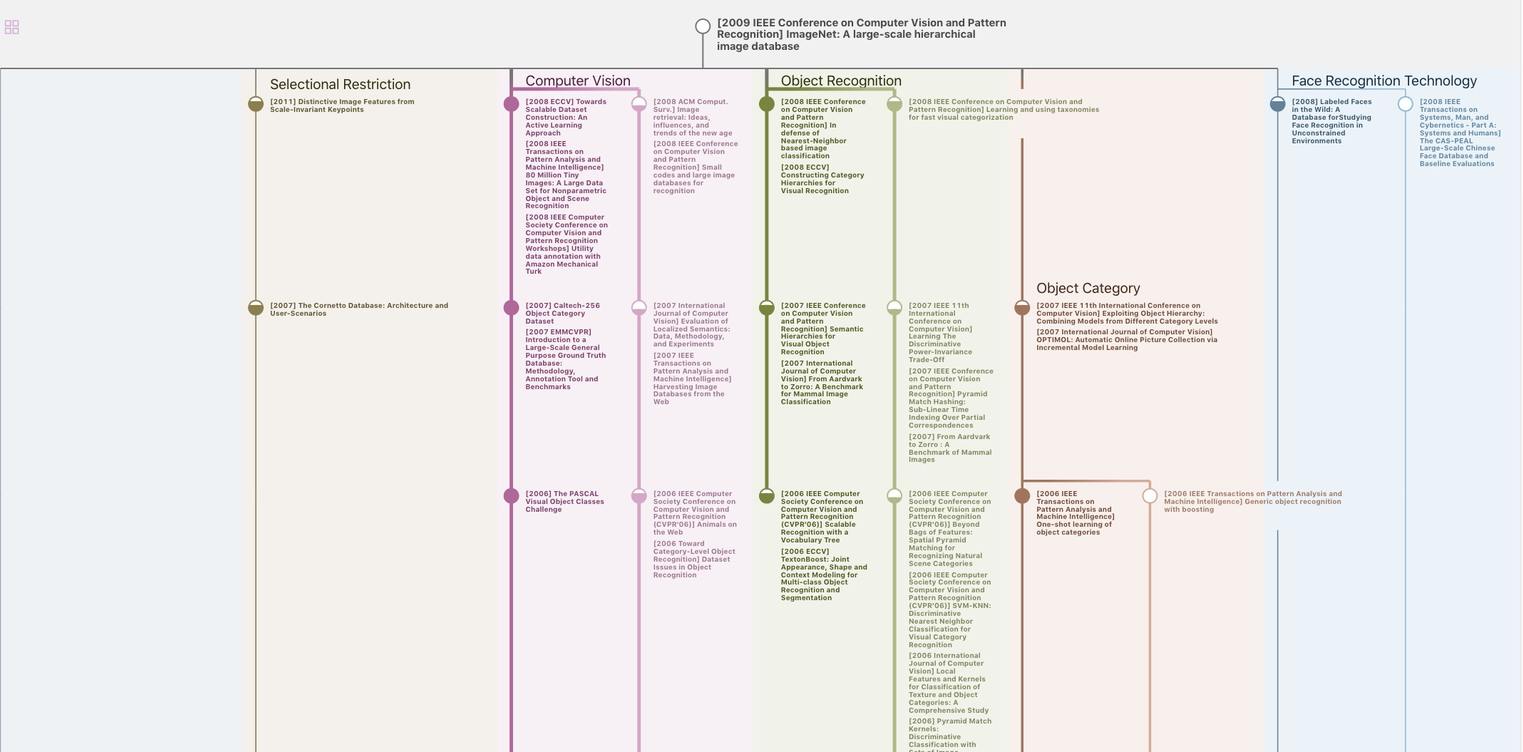
生成溯源树,研究论文发展脉络
Chat Paper
正在生成论文摘要