Machine Learning and Anomaly Detection Algorithms for Damage Characterization From Compliance Data in Three-Point Bending Fatigue
Journal of Nondestructive Evaluation, Diagnostics and Prognostics of Engineering Systems(2021)
摘要
Three-point bending fatigue compliance datasets of multi-layer fiberglass-weave/epoxy test specimens, including 5 and 10 mil interlayers, were analyzed using artificial intelligence (AI) methods along with statistical analysis, revealing the existence of three different compliance-based damage modes. Anomaly detection algorithms helped discover damage indicators observable in short intervals (of 50 cycles) in the compliance data, whose patterns vary with the material and the number of load cycles to which the material is subjected. Machine learning algorithms were applied using the compliance features to assess the likelihood that material failure may occur within a certain number of future loading cycles. High accuracy, precision, and recall rates were achieved in the classification task, for which we evaluated several algorithms, including various variations of neural networks and support vector machines. Thus, our work demonstrates the utility of AI algorithms for discovering a diversity of damage mechanisms and failures.
更多查看译文
关键词
damage classification,failure analysis,anomaly detection,failure prediction
AI 理解论文
溯源树
样例
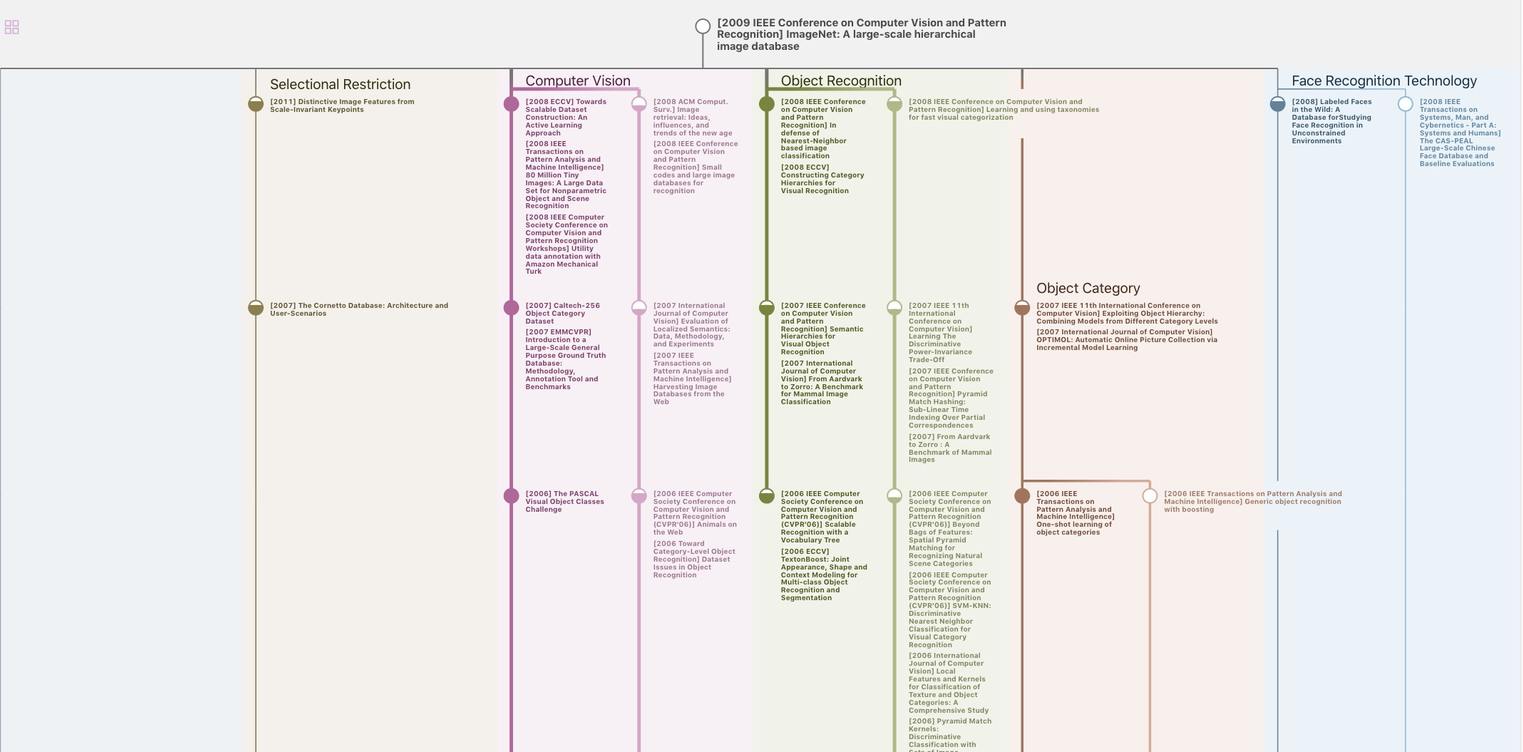
生成溯源树,研究论文发展脉络
Chat Paper
正在生成论文摘要