Modeling Multiple Temporal Scales of Full-Body Movements for Emotion Classification
IEEE Transactions on Affective Computing(2023)
摘要
This article investigates classification of emotions from full-body movements by using a novel Convolutional Neural Network-based architecture. The model is composed of two shallow networks processing in parallel when the 8-bit RGB images obtained from time intervals of 3D-positional data are the inputs. One network performs a coarse-grained modelling in the time domain while the other one applies a fine-grained modelling. We show that combining different temporal scales into a single architecture improves the classification results of a dataset composed of short excerpts of the performances of professional dancers who interpreted four affective states: anger, happiness, sadness, and insecurity. Additionally, we investigate the effect of data chunk duration, overlapping, the size of the input images and the contribution of several data augmentation strategies for our proposed method. Better recognition results were obtained when the duration of a data chunk was longer, and this was further improved by applying balanced data augmentation. Moreover, we test our method on other existing motion capture datasets and compare the results with prior art. In all experiments, our results surpassed the state-of-the-art approaches, showing that this method generalizes across diverse settings and contexts.
更多查看译文
关键词
Emotion recognition,Computer architecture,Hidden Markov models,Legged locomotion,Data models,Computational modeling,Videos,convolutional neural network,full-body movements,kinematics,multiple temporal scales,motion capture
AI 理解论文
溯源树
样例
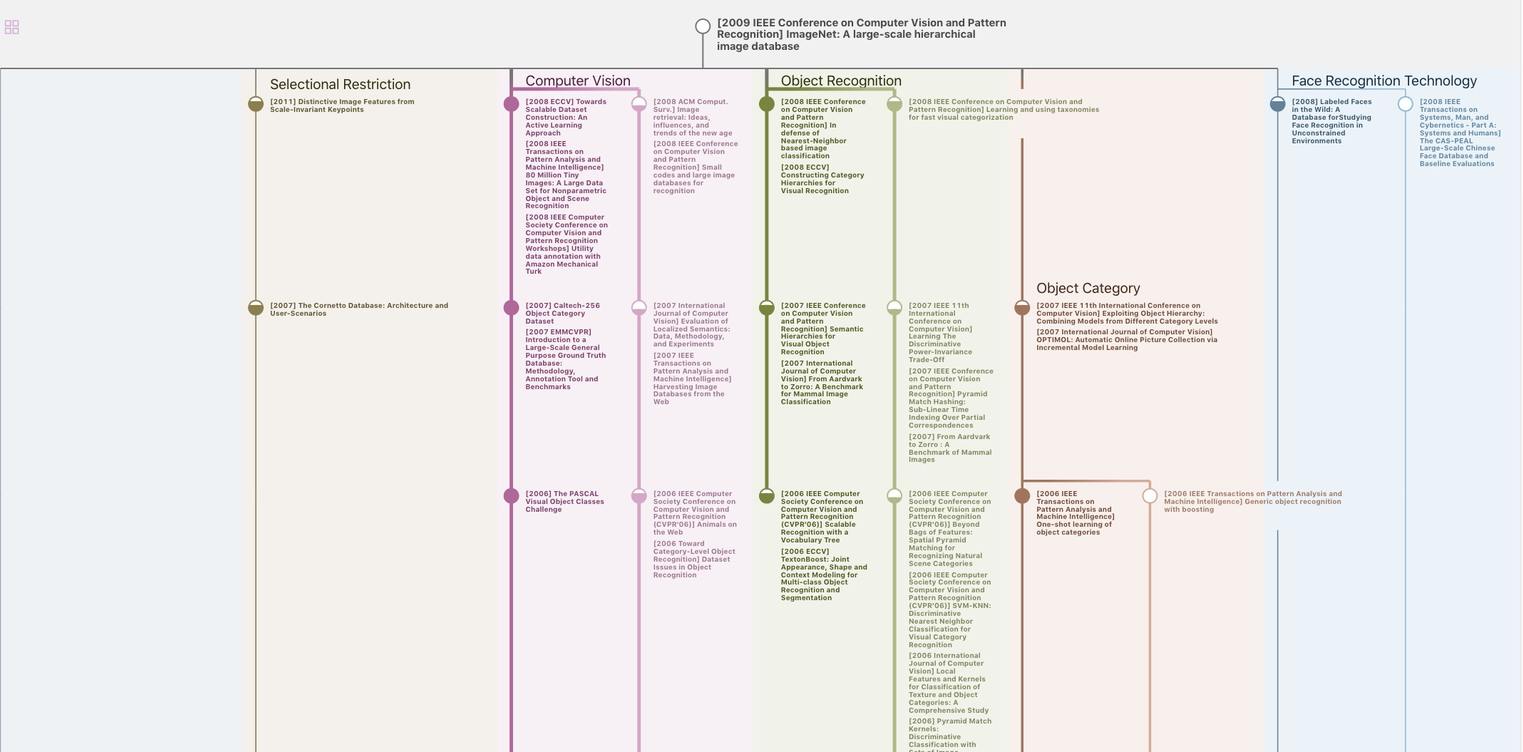
生成溯源树,研究论文发展脉络
Chat Paper
正在生成论文摘要