Graph Attention Transformer Network for Multi-label Image Classification.
arxiv(2023)
摘要
Multi-label classification aims to recognize multiple objects or attributes from images. The key to solving this issue relies on effectively characterizing the inter-label correlations or dependencies, which bring the prevailing graph neural network. However, current methods often use the co-occurrence probability of labels based on the training set as the adjacency matrix to model this correlation, which is greatly limited by the dataset and affects the model's generalization ability. This article proposes a Graph Attention Transformer Network, a general framework for multi-label image classification by mining rich and effective label correlation. First, we use the cosine similarity value of the pre-trained label word embedding as the initial correlation matrix, which can represent richer semantic information than the co-occurrence one. Subsequently, we propose the graph attention transformer layer to transfer this adjacency matrix to adapt to the current domain. Our extensive experiments have demonstrated that our proposed methods can achieve highly competitive performance on three datasets.
更多查看译文
关键词
Graph neural network,transformer,attention mechanism,multi-label classification
AI 理解论文
溯源树
样例
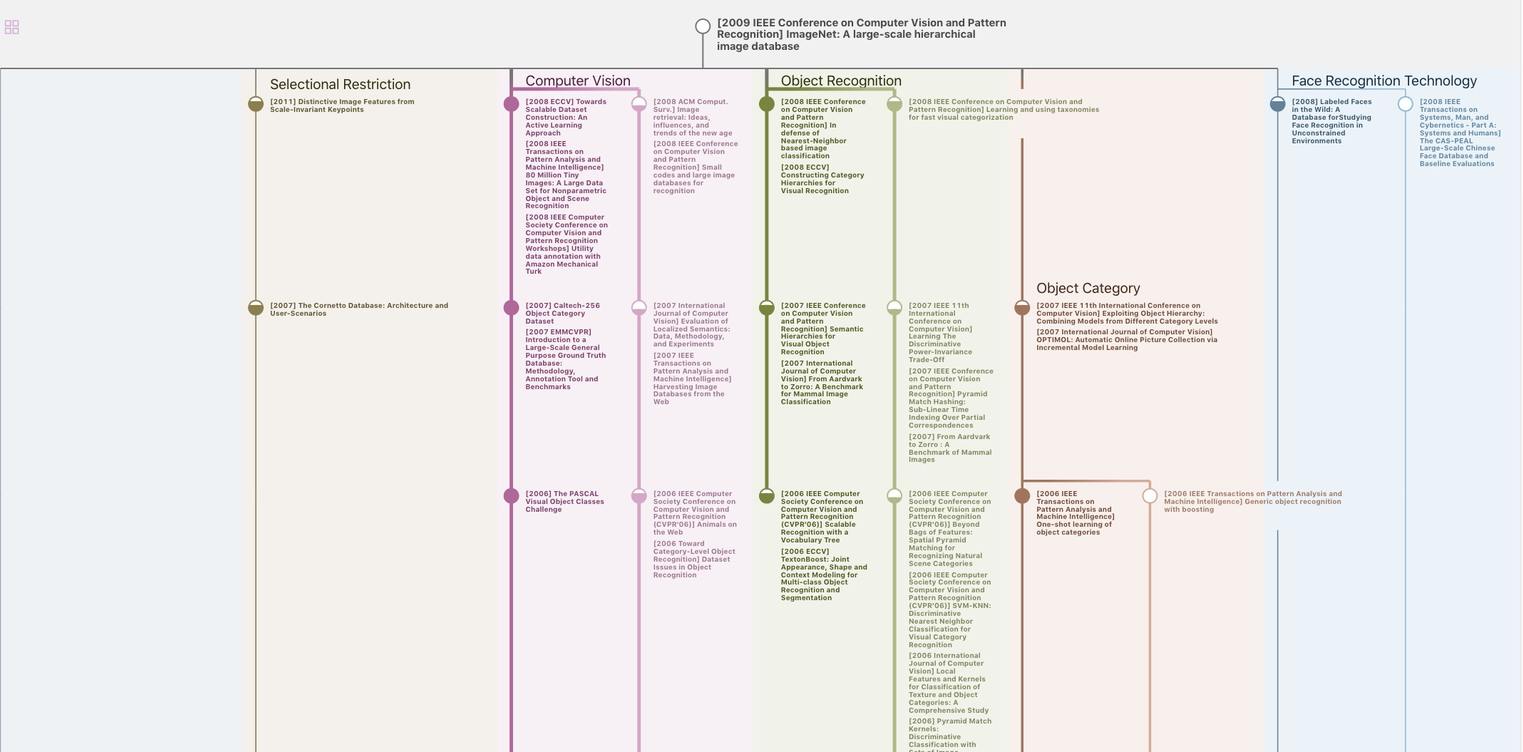
生成溯源树,研究论文发展脉络
Chat Paper
正在生成论文摘要