Hierarchically Structured Scheduling and Execution of Tasks in a Multi-agent Environment
PROGRESS IN ARTIFICIAL INTELLIGENCE, EPIA 2022(2022)
摘要
In a warehouse environment, tasks appear dynamically. Consequently, a task management system that matches them with the workforce too early (e.g., weeks in advance) is necessarily sub-optimal. Also, the rapidly increasing size of the action space of such a system consists of a significant problem for traditional schedulers. Reinforcement learning, however, is suited to deal with issues requiring making sequential decisions towards a long-term, often remote, goal. In this work, we set ourselves on a problem that presents itself with a hierarchical structure: the task-scheduling, by a centralised agent, in a dynamic warehouse multi-agent environment and the execution of one such schedule, by decentralised agents with only partial observability thereof. We propose to use deep reinforcement learning to solve both the high-level scheduling problem and the low-level multi-agent problem of schedule execution. The topic and contribution is relevant to both reinforcement learning and operations research scientific communities and is directed towards future real-world industrial applications.
更多查看译文
关键词
Hierarchical reinforcement learning, Multi-agent systems
AI 理解论文
溯源树
样例
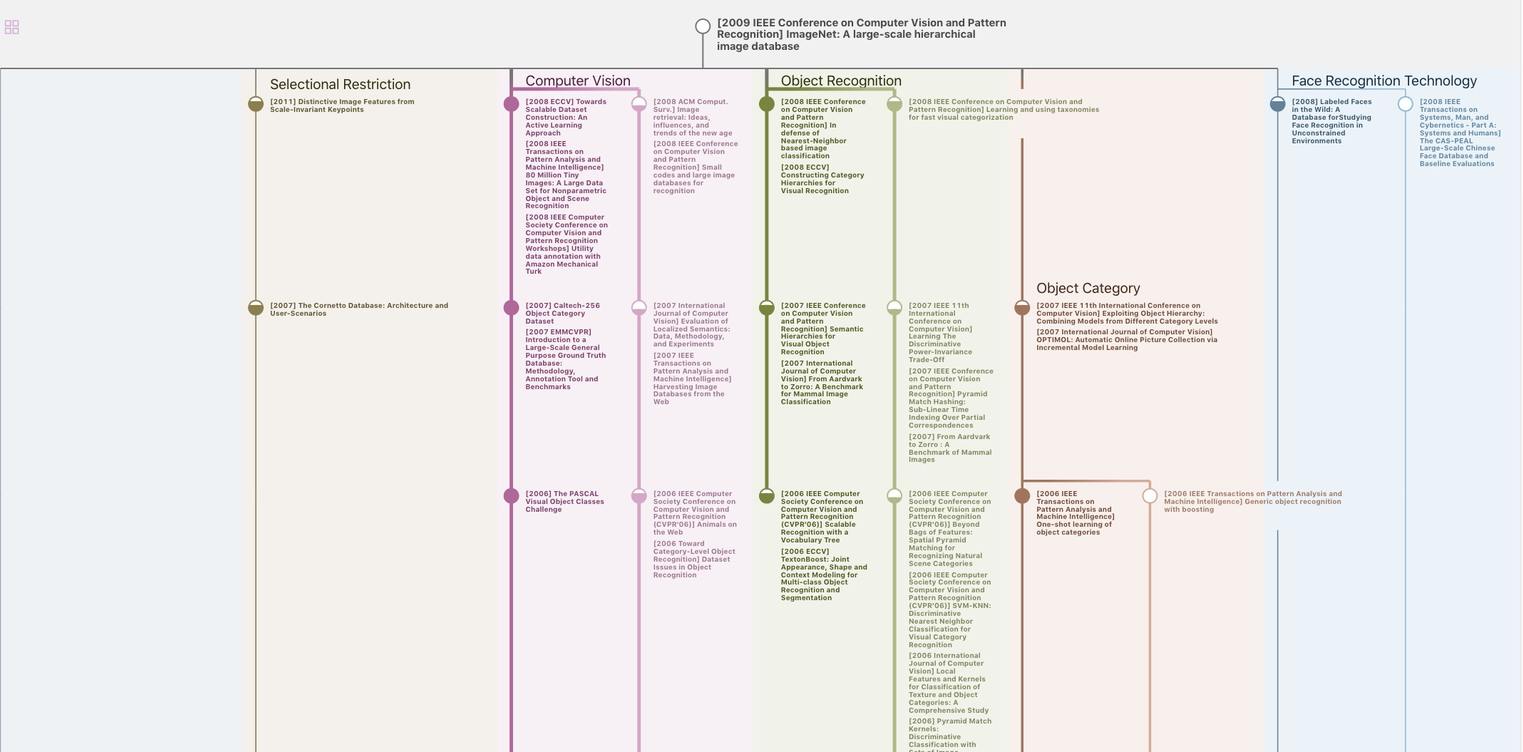
生成溯源树,研究论文发展脉络
Chat Paper
正在生成论文摘要