Reinforcement learning based energy-efficient internet-of-things video transmission
Intelligent and Converged Networks(2020)
摘要
The video transmission in the Internet-of-Things (IoT) system must guarantee the video quality and reduce the packet loss rate and the delay with limited resources to satisfy the requirement of multimedia services. In this paper, we propose a reinforcement learning based energy-efficient IoT video transmission scheme that protects against interference, in which the base station controls the transmission action of the IoT device including the encoding rate, the modulation and coding scheme, and the transmit power. A reinforcement learning algorithm state-action-reward-state-action is applied to choose the transmission action based on the observed state (the queue length of the buffer, the channel gain, the previous bit error rate, and the previous packet loss rate) without knowledge of the transmission channel model at the transmitter and the receiver. We also propose a deep reinforcement learning based energy-efficient IoT video transmission scheme that uses a deep neural network to approximate Q value to further accelerate the learning process involved in choosing the optimal transmission action and improve the video transmission performance. Moreover, both the performance bounds of the proposed schemes and the computational complexity are theoretically derived. Simulation results show that the proposed schemes can increase the peak signal-to-noise ratio and decrease the packet loss rate, the delay, and the energy consumption relative to the benchmark scheme.
更多查看译文
关键词
Internet-of-Things (IoT),video transmission,reinforcement learning,energy efficiency
AI 理解论文
溯源树
样例
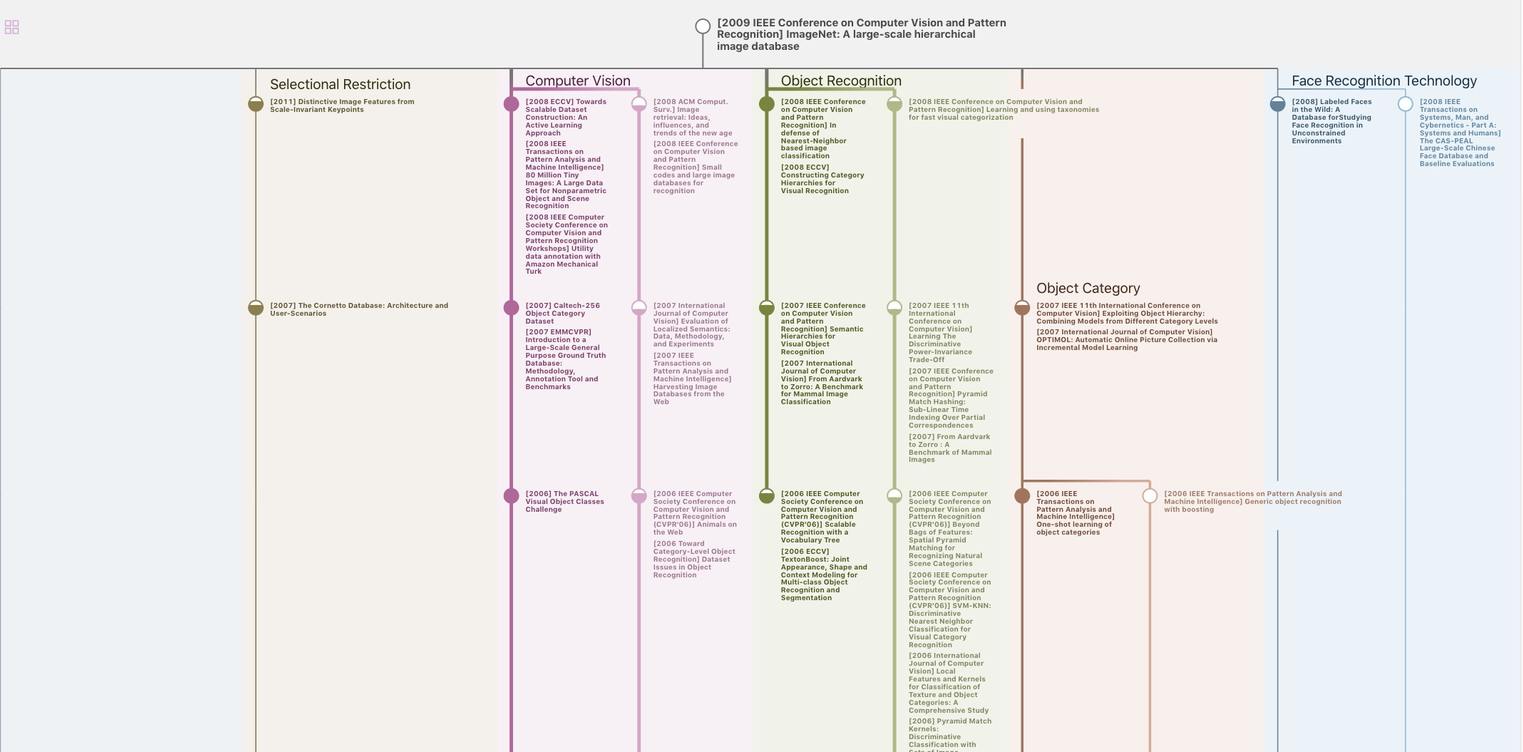
生成溯源树,研究论文发展脉络
Chat Paper
正在生成论文摘要