Robust Multi-View Clustering With Incomplete Information
IEEE Transactions on Pattern Analysis and Machine Intelligence(2023)
摘要
The success of existing multi-view clustering methods heavily relies on the assumption of view consistency and instance completeness, referred to as the complete information. However, these two assumptions would be inevitably violated in data collection and transmission, thus leading to the so-called Partially View-unaligned Problem (PVP) and Partially Sample-missing Problem (PSP). To overcome such incomplete information challenges, we propose a novel method, termed robuSt mUlti-view clusteRing with incomplEte information (SURE), which solves PVP and PSP under a unified framework. In brief, SURE is a novel contrastive learning paradigm which uses the available pairs as positives and randomly chooses some cross-view samples as negatives. To reduce the influence of the false negatives caused by random sampling, SURE is with a noise-robust contrastive loss that theoretically and empirically mitigates or even eliminates the influence of the false negatives. To the best of our knowledge, this could be the first successful attempt that simultaneously handles PVP and PSP using a unified solution. In addition, this could be one of the first studies on the noisy correspondence problem (i.e., the false negatives) which is a novel paradigm of noisy labels. Extensive experiments demonstrate the effectiveness and efficiency of SURE comparing with 10 state-of-the-art approaches on the multi-view clustering task.
更多查看译文
关键词
Unsupervised multi-view representation learning,multi-view clustering,partially view-unaligned problem,partially sample-missing problem,false negatives
AI 理解论文
溯源树
样例
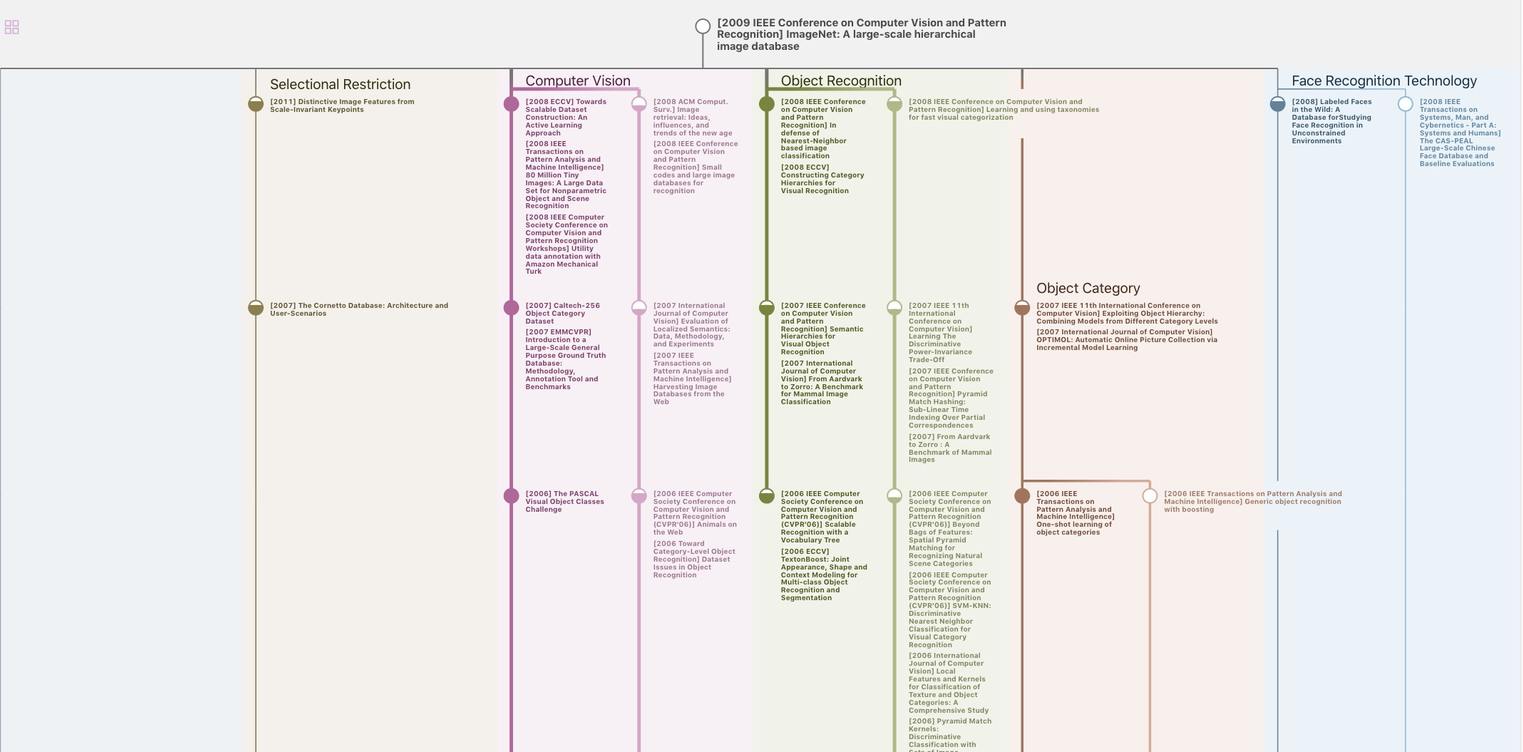
生成溯源树,研究论文发展脉络
Chat Paper
正在生成论文摘要