Reconstruction of univariate functions from directional persistence diagrams
arxiv(2023)
摘要
We describe a method for approximating a single-variable function $f$ using persistence diagrams of sublevel sets of $f$ from height functions in different directions. We provide algorithms for the piecewise linear case and for the smooth case. Three directions suffice to locate all local maxima and minima of a piecewise linear continuous function from its collection of directional persistence diagrams, while five directions are needed in the case of smooth functions with non-degenerate critical points. Our approximation of functions by means of persistence diagrams is motivated by a study of importance attribution in machine learning, where one seeks to reduce the number of critical points of signal functions without a significant loss of information for a neural network classifier.
更多查看译文
关键词
directional persistence
AI 理解论文
溯源树
样例
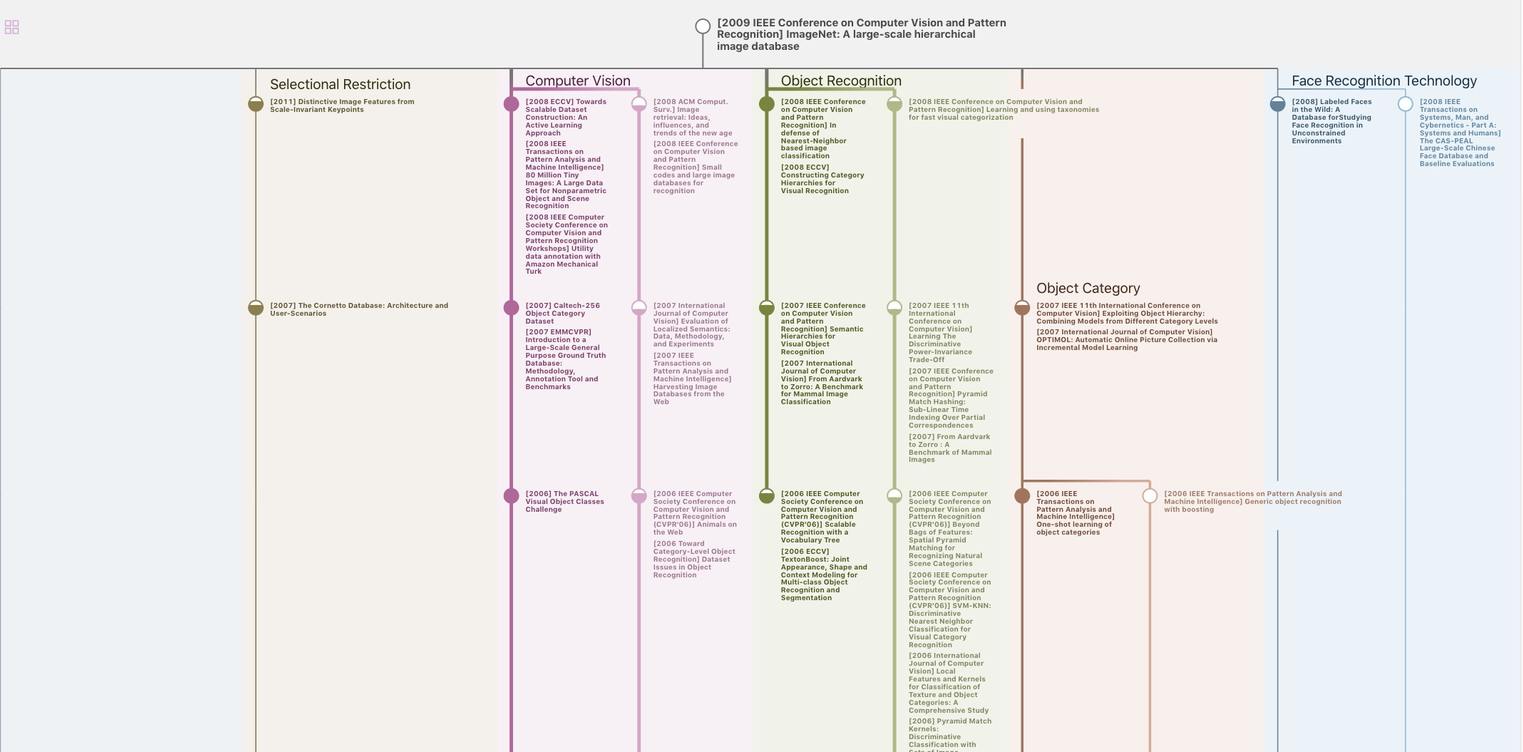
生成溯源树,研究论文发展脉络
Chat Paper
正在生成论文摘要