3D Common Corruptions and Data Augmentation
IEEE Conference on Computer Vision and Pattern Recognition(2022)
摘要
We introduce a set of image transformations that can be used as corruptions to evaluate the robustness of models as well as data augmentation mechanisms for training neural networks. The primary distinction of the proposed transformations is that, unlike existing approaches such as Common Corruptions [27], the geometry of the scene is incorporated in the transformations - thus leading to corruptions that are more likely to occur in the real world. We also introduce a set of semantic corruptions (e.g. natural object occlusions. See Fig. 1). We show these transformations are ‘efficient’ (can be computed on-the-fly), ‘extendable’ (can be applied on most image datasets), expose vulnerability of existing models, and can effectively make models more robust when employed as ‘3D data augmentation’ mechanisms. The evaluations on several tasks and datasets suggest incorporating 3D information into benchmarking and training opens up a promising direction for robustness research.
更多查看译文
关键词
Datasets and evaluation, 3D from single images, Adversarial attack and defense, Scene analysis and understanding
AI 理解论文
溯源树
样例
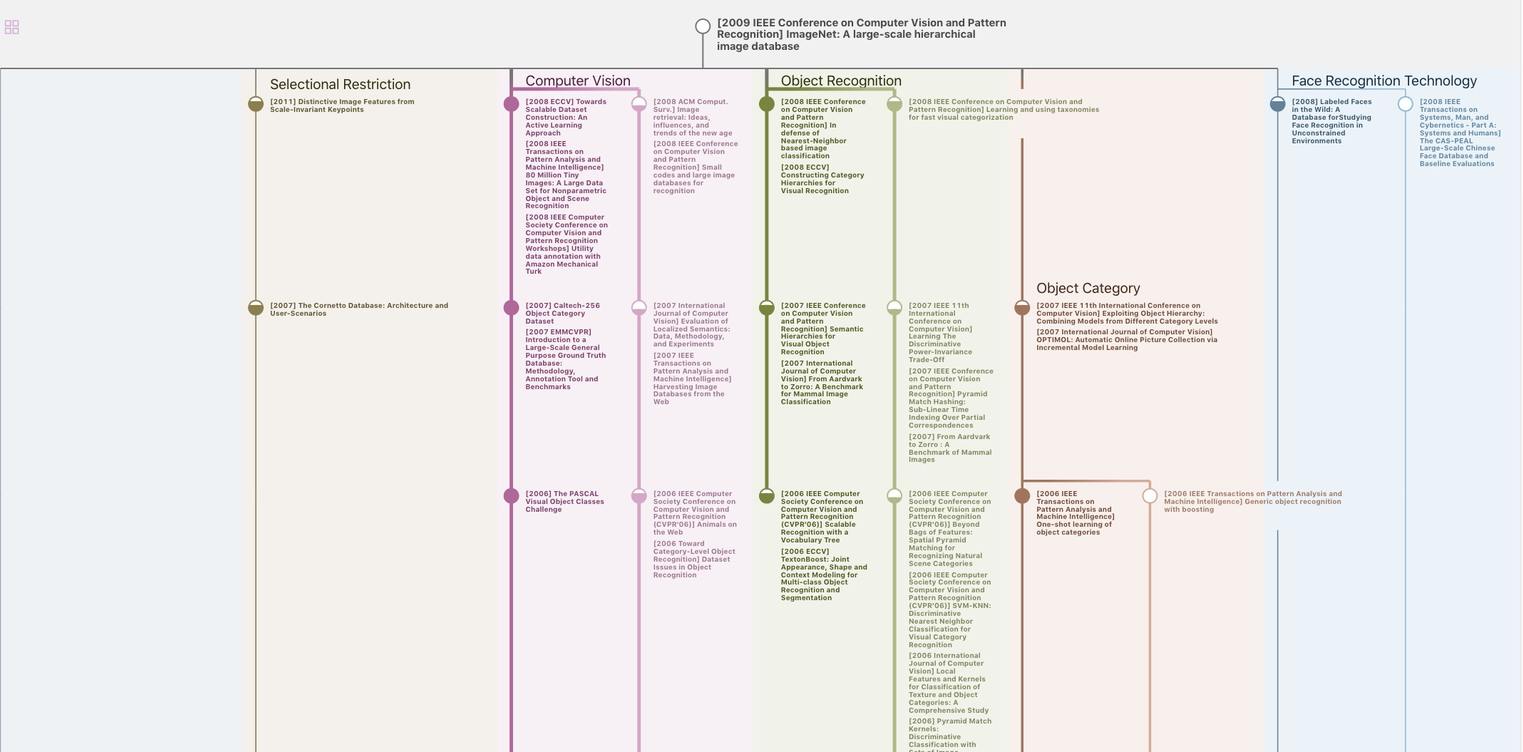
生成溯源树,研究论文发展脉络
Chat Paper
正在生成论文摘要