Rethinking Pretraining as a Bridge from ANNs to SNNs
arxiv(2022)
摘要
Spiking neural networks (SNNs) are known as a typical kind of brain-inspired models with their unique features of rich neuronal dynamics, diverse coding schemes and low power consumption properties. How to obtain a high-accuracy model has always been the main challenge in the field of SNN. Currently, there are two mainstream methods, i.e., obtaining a converted SNN through converting a well-trained Artificial Neural Network (ANN) to its SNN counterpart or training an SNN directly. However, the inference time of a converted SNN is too long, while SNN training is generally very costly and inefficient. In this work, a new SNN training paradigm is proposed by combining the concepts of the two different training methods with the help of the pretrain technique and BP-based deep SNN training mechanism. We believe that the proposed paradigm is a more efficient pipeline for training SNNs. The pipeline includes pipeS for static data transfer tasks and pipeD for dynamic data transfer tasks. SOTA results are obtained in a large-scale event-driven dataset ES-ImageNet. For training acceleration, we achieve the same (or higher) best accuracy as similar LIF-SNNs using 1/10 training time on ImageNet-1K and 2/5 training time on ES-ImageNet and also provide a time-accuracy benchmark for a new dataset ES-UCF101. These experimental results reveal the similarity of the functions of parameters between ANNs and SNNs and also demonstrate the various potential applications of this SNN training pipeline.
更多查看译文
关键词
Training,Task analysis,Neurons,Pipelines,Artificial neural networks,Feature extraction,Transfer learning,Event-driven dataset,neural network (NN) analysis,pretraining technique,spiking NN (SNN),transfer learning
AI 理解论文
溯源树
样例
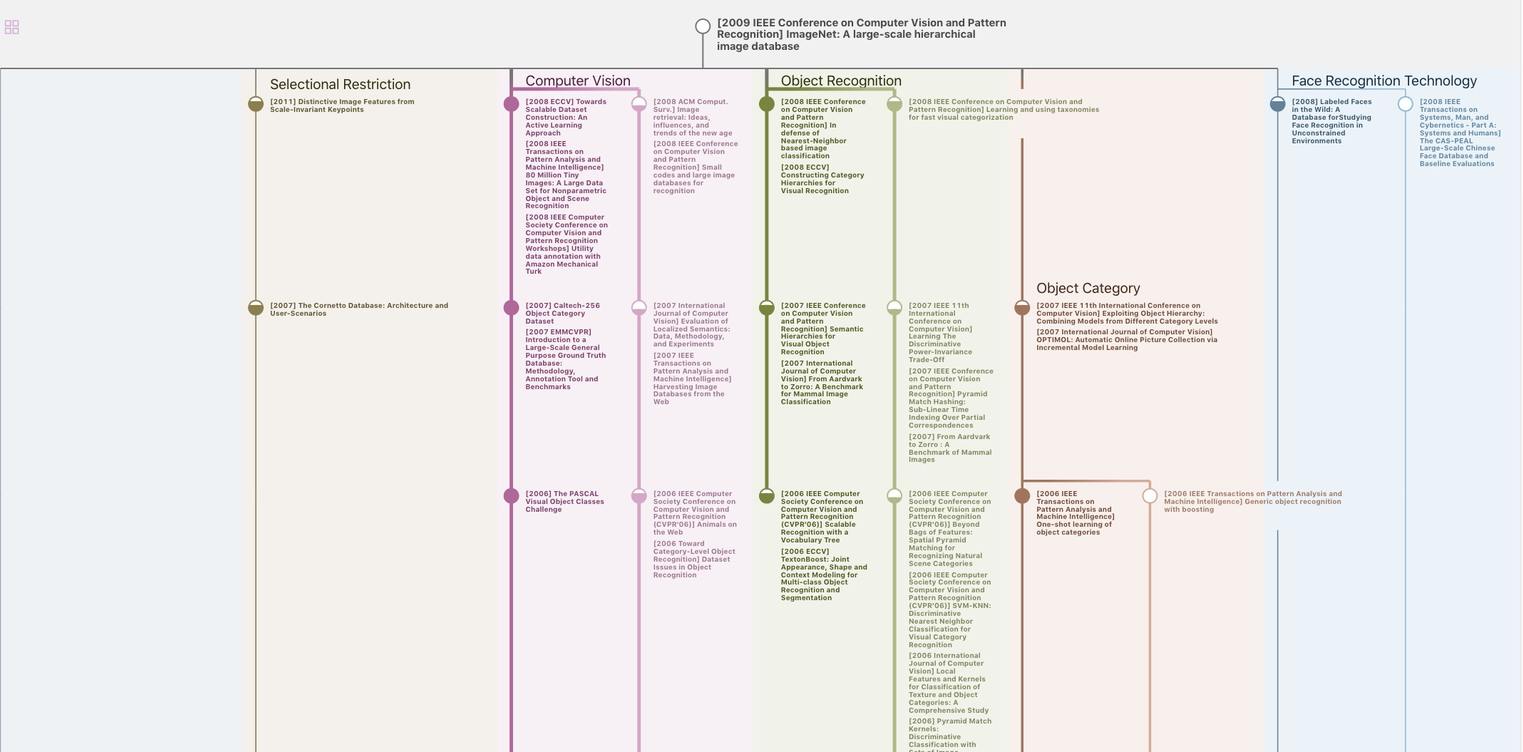
生成溯源树,研究论文发展脉络
Chat Paper
正在生成论文摘要