InsertionNet 2.0: Minimal Contact Multi-Step Insertion Using Multimodal Multiview Sensory Input
IEEE International Conference on Robotics and Automation(2022)
摘要
We address the problem of devising the means for a robot to rapidly and safely learn insertion skills with just a few human interventions and without hand-crafted rewards or demonstrations. Our InsertionNet version 2.0 provides an improved technique to robustly cope with a wide range of use-cases featuring different shapes, colors, initial poses, etc. In particular, we present a regression-based method based on multimodal input from stereo perception and force, augmented with contrastive learning for the efficient learning of valuable features. In addition, we introduce a one-shot learning technique for insertion, which relies on a relation network scheme to better exploit the collected data and to support multi-step insertion tasks. Our method improves on the results obtained with the original InsertionNet, achieving an almost perfect score (above 97.5% on 200 trials) in 16 real-life insertion tasks while minimizing the execution time and contact during insertion. We further demonstrate our method's ability to tackle a real-life 3-step insertion task and perfectly solve an unseen insertion task without learning.
更多查看译文
关键词
input,contact,multi-step
AI 理解论文
溯源树
样例
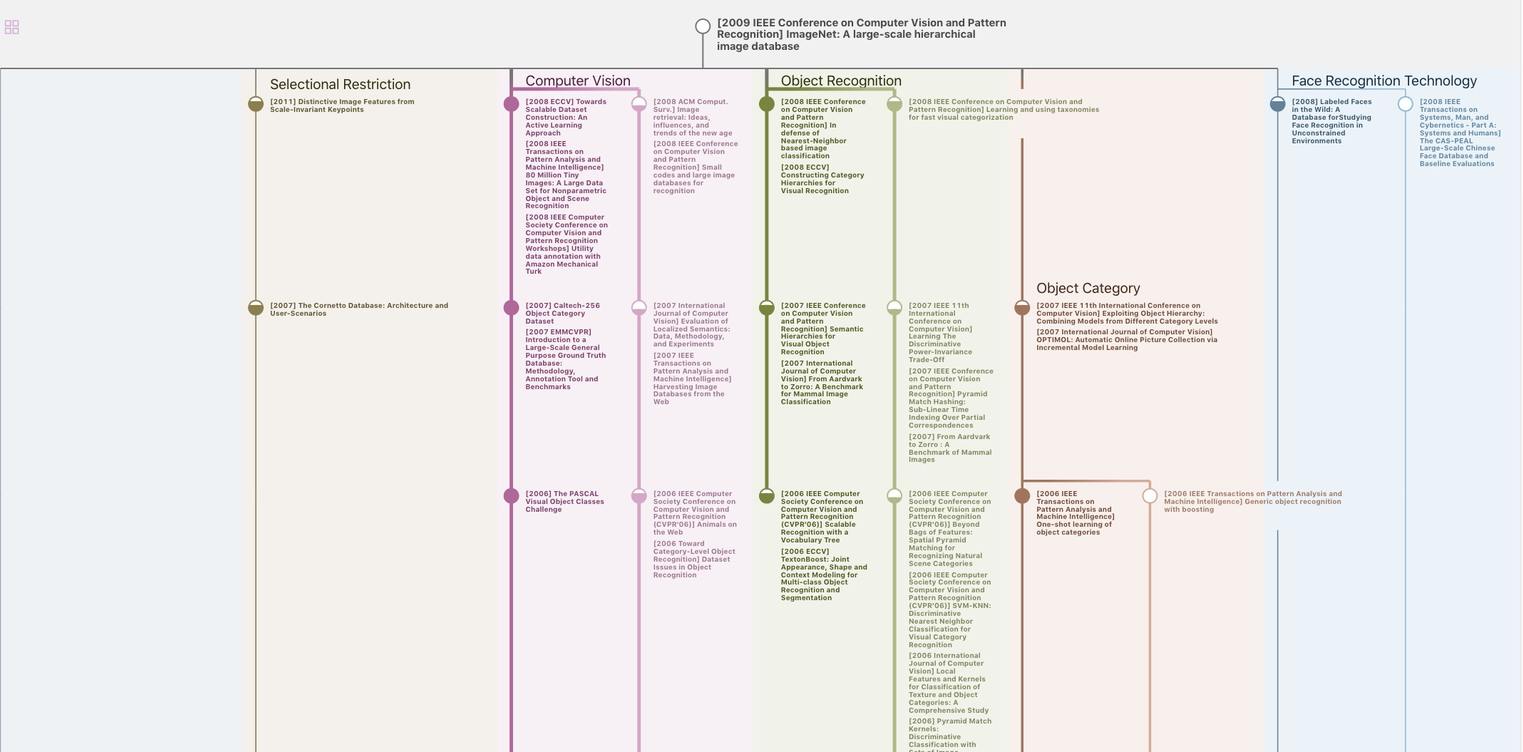
生成溯源树,研究论文发展脉络
Chat Paper
正在生成论文摘要