Decomposed-distance weighted optimal transport for unsupervised domain adaptation
Applied Intelligence(2022)
摘要
Unsupervised Domain Adaptation (UDA) aims to transfer knowledge from a label-rich source domain to an unlabeled target domain with a different but related distribution. Optimal Transport (OT) based Wasserstein distance has recently been used to measure and reduce the domain discrepancy in virtue of its robustness in distance measurement. However, the inaccurate estimation of the transport cost between samples is harmful to the fine-grained domain alignment. This paper proposes Decomposed-Distance Weighted Optimal Transport (DDW-OT) method for better adaptation. Technically, according to the clustering-based prototype generation (CPG), DDW-OT constructs a decomposed-distance reweighing matrix to revise the original inaccurate transport distance on sample-level, which conjoins the category uncertainty of the target samples and the correlation degree of category between domains. Besides, the dual-OT solver takes neural netw11 orks to parameterize the dual variables and alleviate the computation cost. DDW-OT also allocated an explicit class-conditional alignment strategy to enhance transfer performance. Extensive experiments on benchmarks demonstrate the effectiveness of the proposed method.
更多查看译文
关键词
Optimal transport, Unsupervised domain adaptation, Deep clustering, Wasserstein distance
AI 理解论文
溯源树
样例
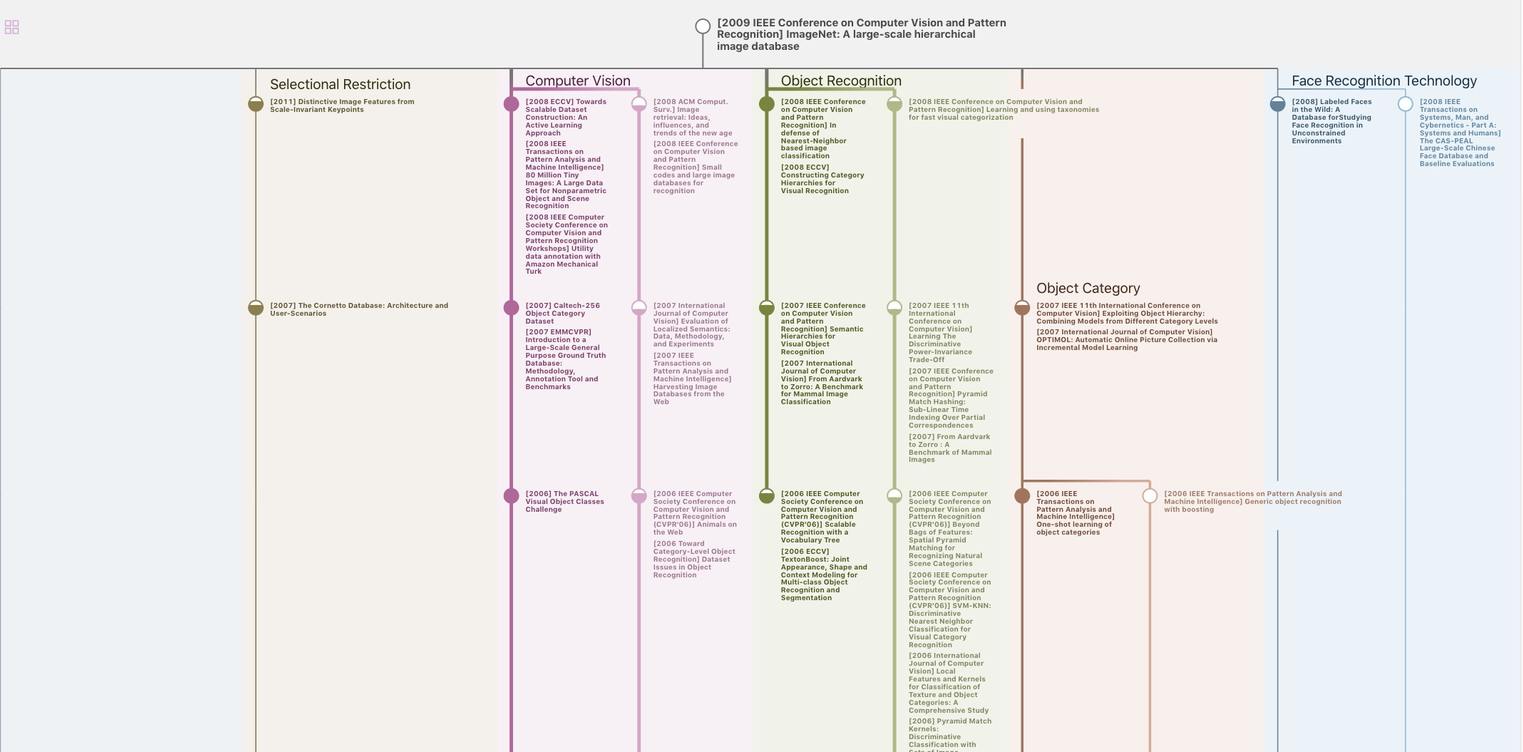
生成溯源树,研究论文发展脉络
Chat Paper
正在生成论文摘要