Recovery of Missing Sensor Data by Reconstructing Time-varying Graph Signals.
European Signal Processing Conference (EUSIPCO)(2022)
摘要
Wireless sensor networks are among the most promising technologies of the current era because of their small size, lower cost, and ease of deployment. With the increasing number of wireless sensors, the probability of generating missing data also rises. This incomplete data could lead to disastrous consequences if used for decision-making. There is rich literature dealing with this problem. However, most approaches show performance degradation when a sizable amount of data is lost. Inspired by the emerging field of graph signal processing, this paper performs a new study of a Sobolev reconstruction algorithm in wireless sensor networks. Experimental comparisons on several publicly available datasets demonstrate that the algorithm surpasses multiple state-of-the-art techniques by a maximum margin of 54%. We further show that this algorithm consistently retrieves the missing data even during massive data loss situations.
更多查看译文
关键词
missing sensor data,time-varying
AI 理解论文
溯源树
样例
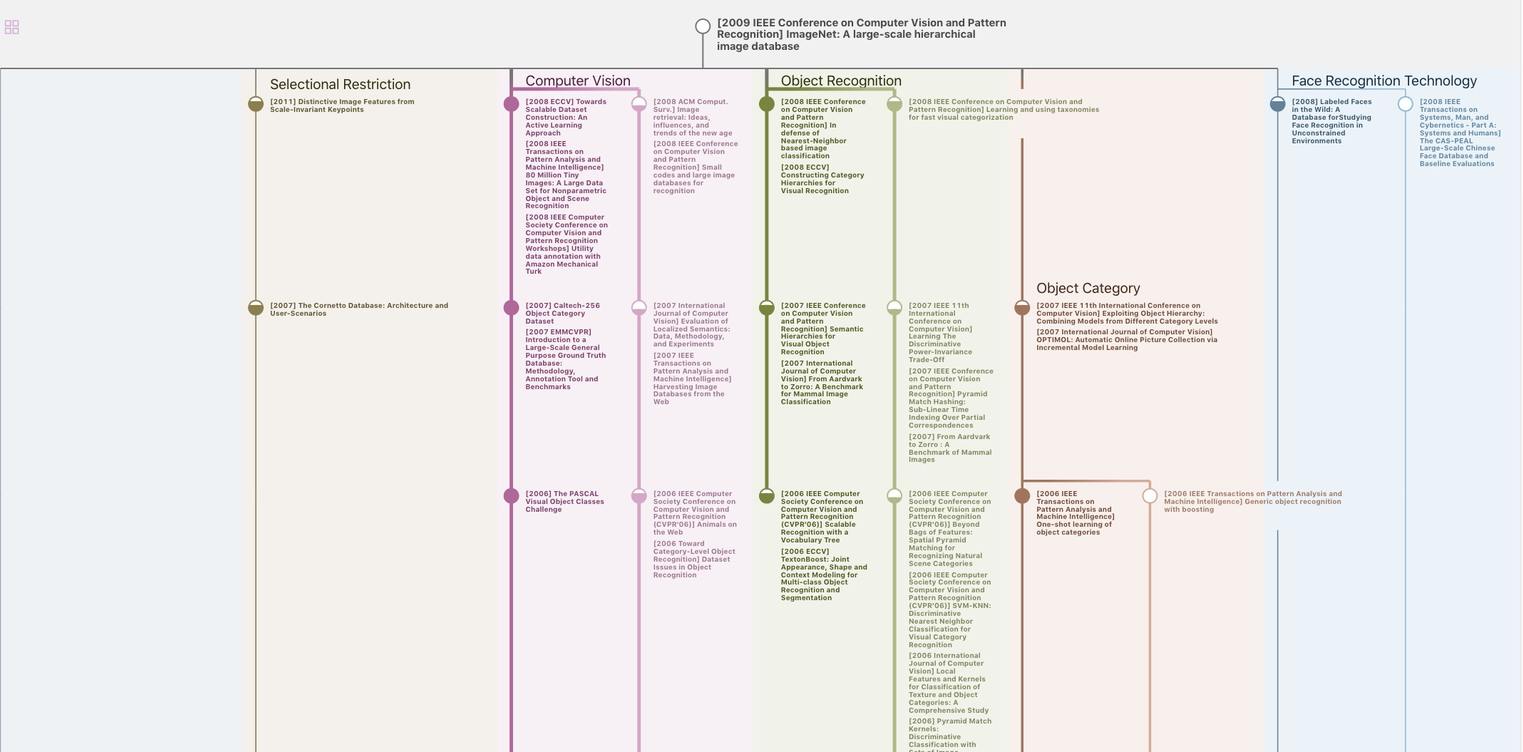
生成溯源树,研究论文发展脉络
Chat Paper
正在生成论文摘要