Neural Ordinary Differential Equations for Nonlinear System Identification.
ACC(2022)
摘要
Neural ordinary differential equations (NODE) have been recently proposed as a promising approach for nonlinear system identification tasks. In this work, we systematically compare their predictive performance with current state-of-the-art nonlinear and classical linear methods. In particular, we present a quantitative study comparing NODE's performance against neural state-space models and classical linear system identification methods. We evaluate the inference speed and prediction performance of each method on open-loop errors across eight different dynamical systems. The experiments show that NODEs can consistently improve the prediction accuracy by an order of magnitude compared to benchmark methods. Besides improved accuracy, we also observed that NODEs are less sensitive to hyperparameters compared to neural state-space models. On the other hand, these performance gains come with a slight increase of computation at the inference time.
更多查看译文
关键词
nonlinear system identification,differential equations
AI 理解论文
溯源树
样例
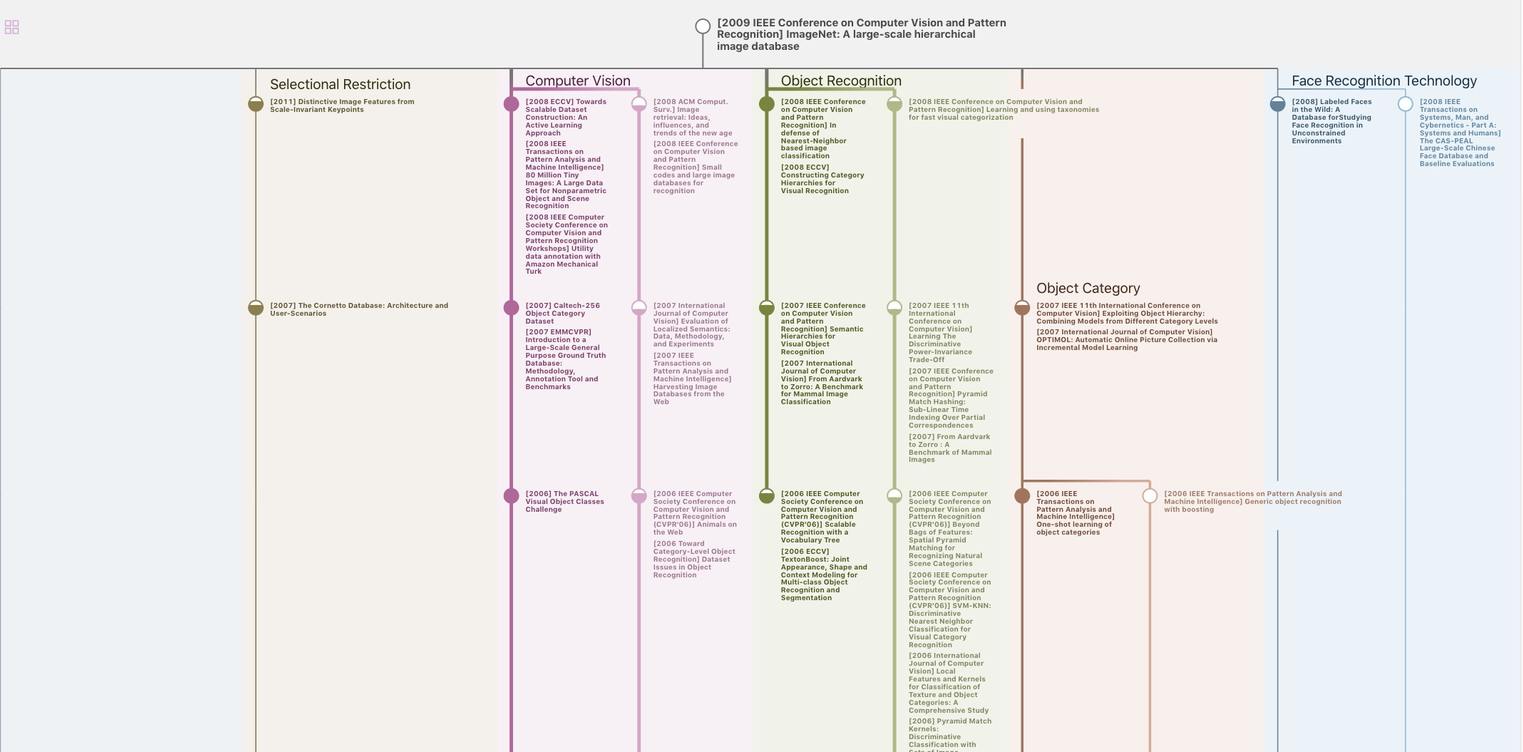
生成溯源树,研究论文发展脉络
Chat Paper
正在生成论文摘要