Machine Learning in Nested Simulations Under Actuarial Uncertainty
Springer eBooks(2021)
摘要
The Solvency II directive states that in order to be solvent the insurance undertakings must to hold eligible own funds covering the Solvency Capital Requirement (SCR), which is defined as the Value-at-Risk of the NAV probability distribution (PDF in the directive) at a confidence level of 99.5% over a one-year period. The estimation of the SCR requires the evaluation of the NAV (under risk-neutral probabilities) conditionally to the economic and actuarial scenarios estimated under real-world probabilities and involve nested Monte Carlo simulations. This approach usually presents unacceptable computational costs. In this paper we analyse the performance of Machine Learning techniques on some insurance portfolios considering a multivariate stochastic model for actuarial risks including mortality, lapse and expense risks. Experiments are aimed not only to analyse the performance of these techniques in a large-dimensional risk framework, but also to investigate variability and robustness of the obtained estimations.
更多查看译文
关键词
Solvency capital requirement, Life insurance, Support vector machines, Deep neural networks, Actuarial risks
AI 理解论文
溯源树
样例
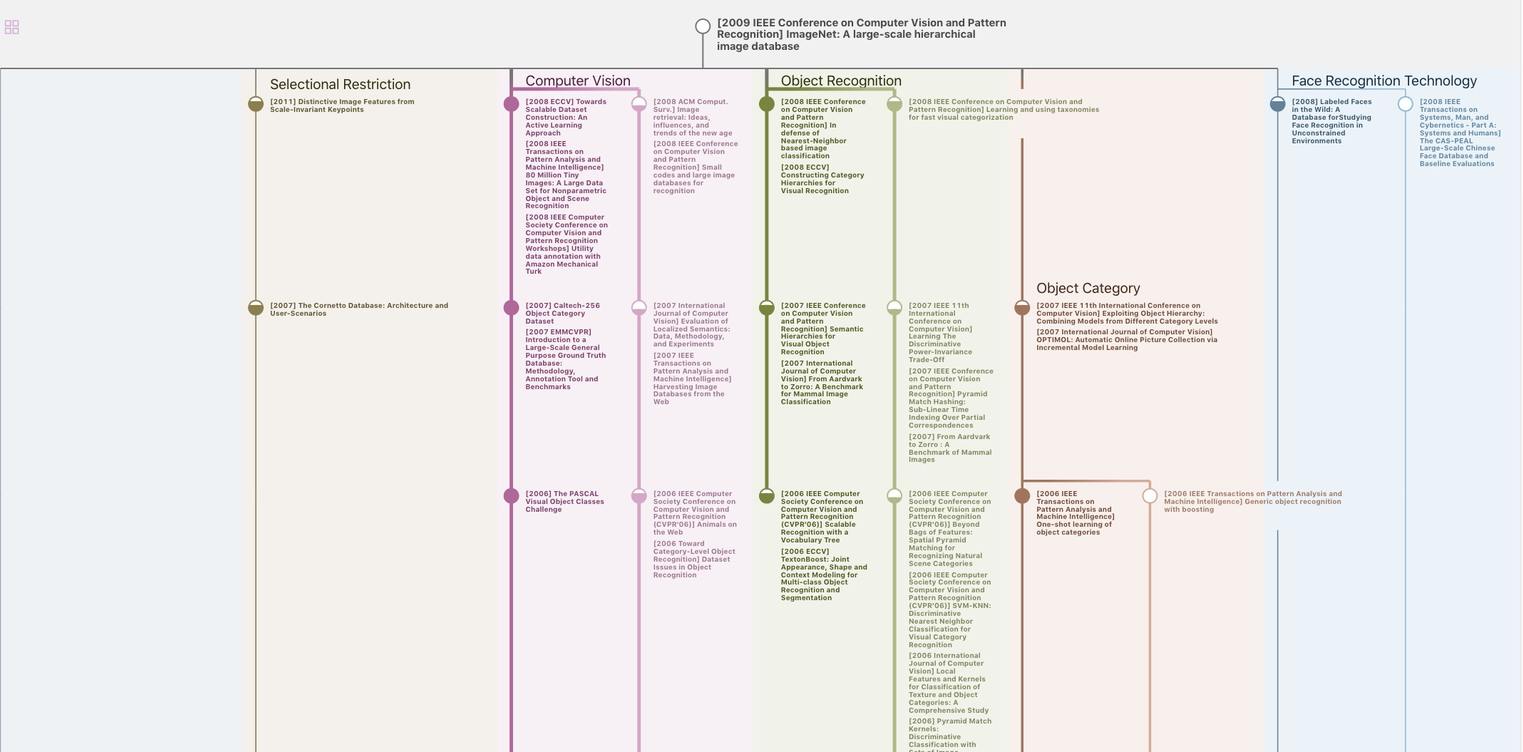
生成溯源树,研究论文发展脉络
Chat Paper
正在生成论文摘要