Long Short-Term Memory in Chemistry Dynamics Simulation
ADVANCES IN ARTIFICIAL INTELLIGENCE AND APPLIED COGNITIVE COMPUTING(2021)
摘要
Chemistry dynamics simulation is widely used in quantitative structure activity relationship QSAR, virtual screening, protein structure prediction, quantum chemistry, materials design, and property prediction, etc. This chapter explores the idea of integrating Long Short-Term Memory (LSTM) with chemistry dynamics simulations to enhance the performance of the simulation and improve its usability for research and education. The idea is successfully used to predict the location, energy, and Hessian of atoms in a H2O reaction system. The results demonstrate that the artificial neural network–based memory model successfully learns the desired features associated with the atomic trajectory and rapidly generates predictions that are in excellent agreement with the results from chemistry dynamics simulations. The accuracy of the prediction is better than expected.
更多查看译文
关键词
Long Short-Term Memory, Deep Learning, Chemistry Dynamics Simulation
AI 理解论文
溯源树
样例
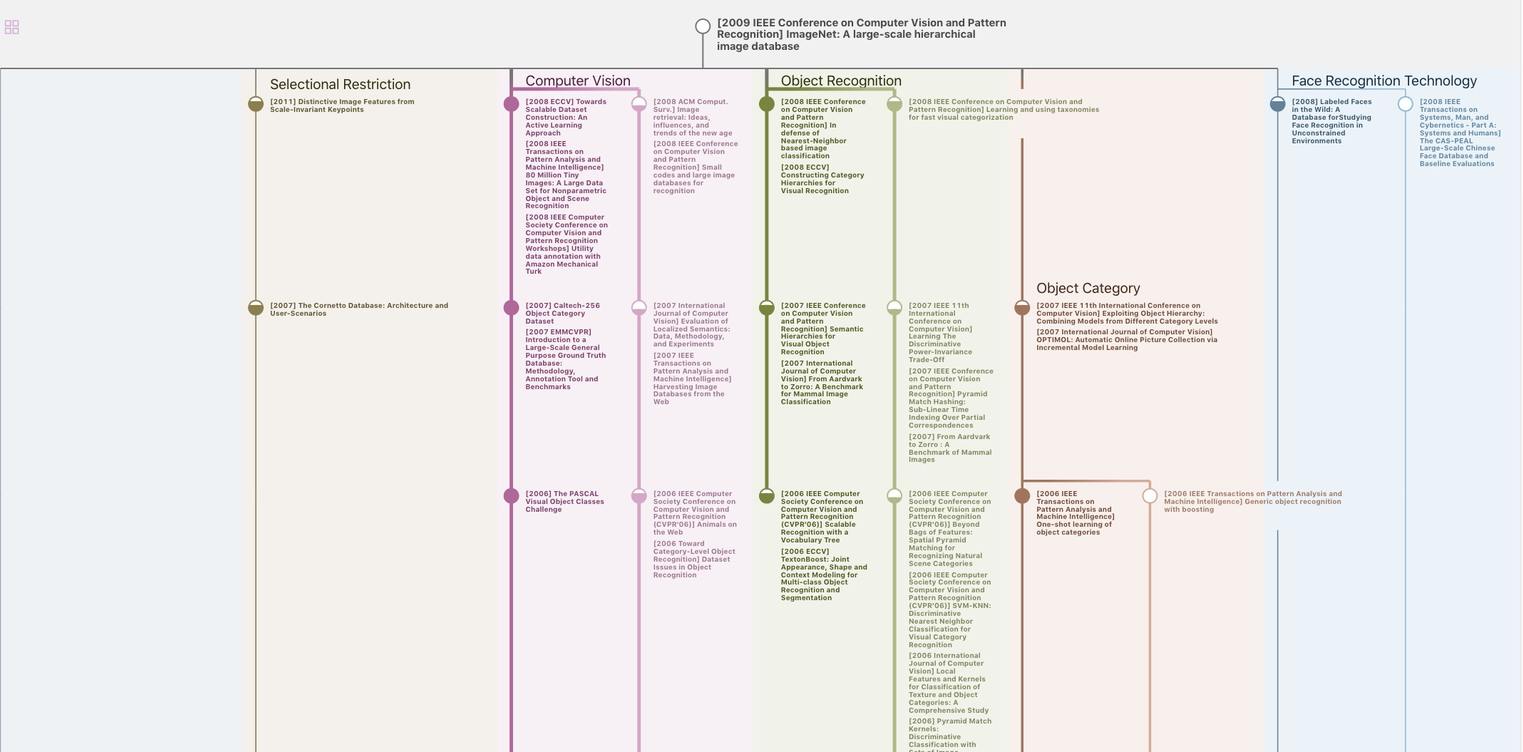
生成溯源树,研究论文发展脉络
Chat Paper
正在生成论文摘要