Remote Sensing Scene Classification with Multi-task Learning
springer
摘要
Remote sensing scene classification is a fundamental task for understanding the high-resolution remote sensing images and has achieved great progress thanks to the deep neural networks. However, the deep neural networks heavily depend on the large-scale training samples, while sufficient manually annotated data is often unavailable. In this paper, a Multi-Task Scene Classification Network (MTSCN) is proposed to address the label consumption dilemma by using annotated information from other related tasks. The MTSCN simultaneously conducts multiple tasks so that scene classification task can leverage information shared by other tasks. The MTSCN consists of a shared branch and multiple task-specific branches with each for one task. First, the shared branch extracts shared features for all tasks. Then, the task-specific branch adapts shared features into task-specific features to finish the classification task. Such architecture allows complementary information shared among tasks as well as avoids task interference by selecting specific information for a different task. The method is evaluated on two multi-task learning scenarios with respect to accuracy and the number of network parameters. The results show that the proposed method is efficient not only in terms of data but also in terms of network parameters.
更多查看译文
关键词
Remote sensing scene classification, Multi-task learning, Attention, Parameter efficient
AI 理解论文
溯源树
样例
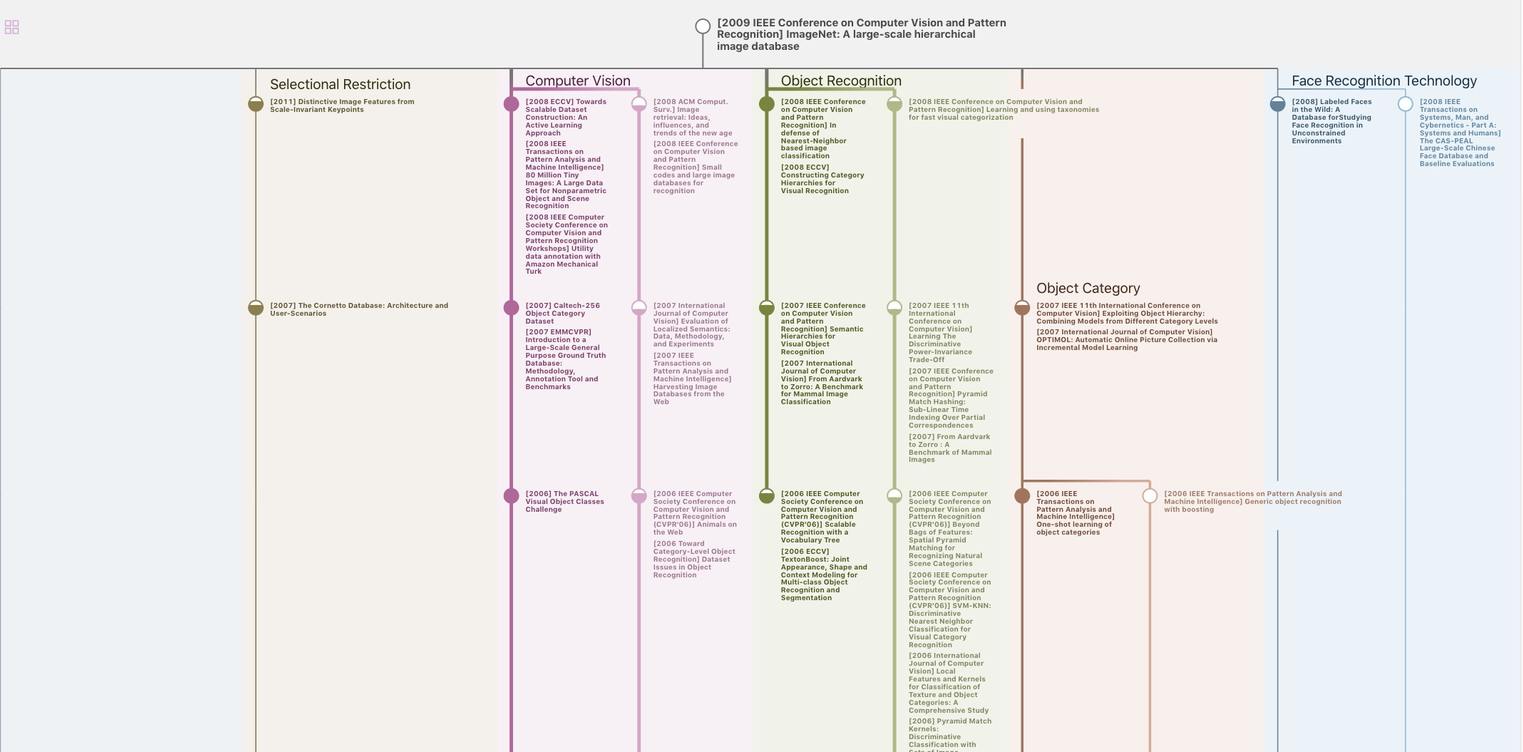
生成溯源树,研究论文发展脉络
Chat Paper
正在生成论文摘要