LCP-dropout: Compression-based Multiple Subword Segmentation for Neural Machine Translation
arxiv(2022)
摘要
In this study, we propose a simple and effective preprocessing method for subword segmentation based on a data compression algorithm. Compression-based subword segmentation has recently attracted significant attention as a preprocessing method for training data in Neural Machine Translation. Among them, BPE/BPE-dropout is one of the fastest and most effective method compared to conventional approaches. However, compression-based approach has a drawback in that generating multiple segmentations is difficult due to the determinism. To overcome this difficulty, we focus on a probabilistic string algorithm, called locally-consistent parsing (LCP), that has been applied to achieve optimum compression. Employing the probabilistic mechanism of LCP, we propose LCP-dropout for multiple subword segmentation that improves BPE/BPE-dropout, and show that it outperforms various baselines in learning from especially small training data.
更多查看译文
关键词
byte-pair encoding, locally consistent parsing, vocabulary, word embedding
AI 理解论文
溯源树
样例
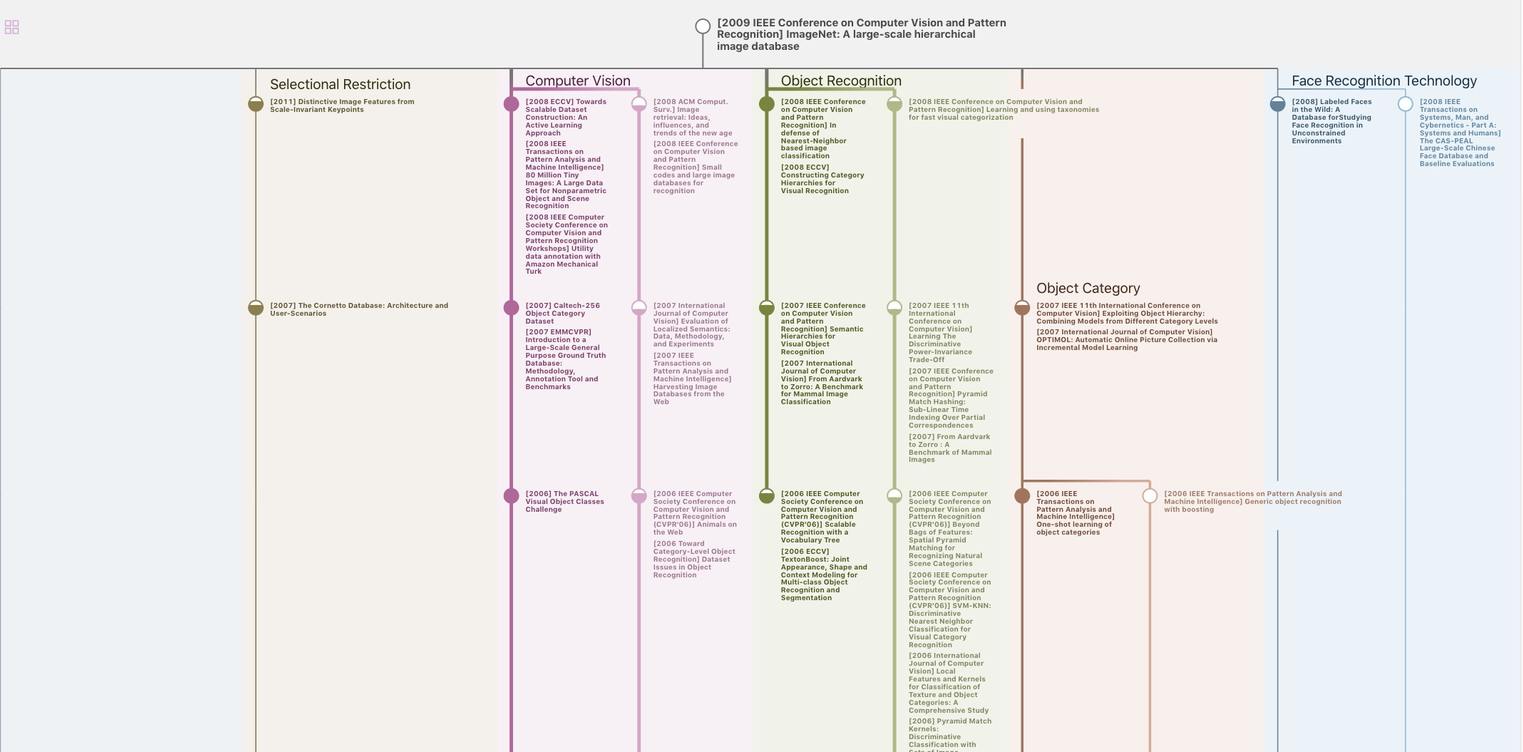
生成溯源树,研究论文发展脉络
Chat Paper
正在生成论文摘要