Split HE: Fast Secure Inference Combining Split Learning and Homomorphic Encryption
arxiv(2022)
摘要
This work presents a novel protocol for fast secure inference of neural networks applied to computer vision applications. It focuses on improving the overall performance of the online execution by deploying a subset of the model weights in plaintext on the client's machine, in the fashion of SplitNNs. We evaluate our protocol on benchmark neural networks trained on the CIFAR-10 dataset using SEAL via TenSEAL and discuss runtime and security performances. Empirical security evaluation using Membership Inference and Model Extraction attacks showed that the protocol was more resilient under the same attacks than a similar approach also based on SplitNN. When compared to related work, we demonstrate improvements of 2.5x-10x for the inference time and 14x-290x in communication costs.
更多查看译文
AI 理解论文
溯源树
样例
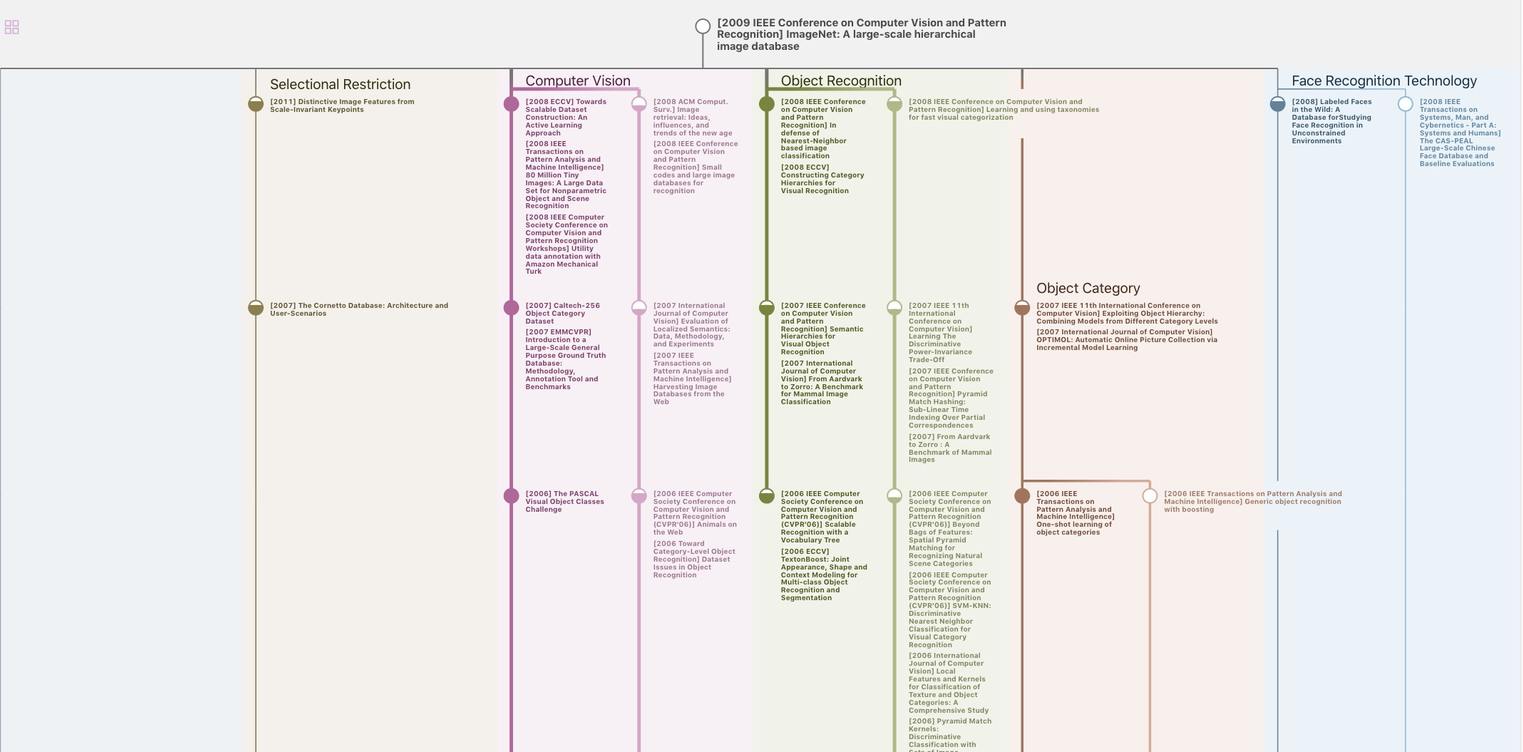
生成溯源树,研究论文发展脉络
Chat Paper
正在生成论文摘要