ctP2ISP: Protein–Protein Interaction Sites Prediction Using Convolution and Transformer With Data Augmentation
IEEE/ACM Transactions on Computational Biology and Bioinformatics(2023)
摘要
Protein–protein interactions are the basis of many cellular biological processes, such as cellular organization, signal transduction, and immune response. Identifying protein–protein interaction sites is essential for understanding the mechanisms of various biological processes, disease development, and drug design. However, it remains a challenging task to make accurate predictions, as the small amount of training data and severe imbalanced classification reduce the performance of computational methods. We design a deep learning method named ctP
2
ISP to improve the prediction of protein–protein interaction sites. ctP
2
ISP employs Convolution and Transformer to extract information and enhance information perception so that semantic features can be mined to identify protein–protein interaction sites. A weighting loss function with different sample weights is designed to suppress the preference of the model toward multi-category prediction. To efficiently reuse the information in the training set, a preprocessing of data augmentation with an improved sample-oriented sampling strategy is applied. The trained ctP
2
ISP was evaluated against current state-of-the-art methods on six public datasets. The results show that ctP
2
ISP outperforms all other competing methods on the balance metrics: F1, MCC, and AUPRC. In particular, our prediction on open tests related to viruses may also be consistent with biological insights. The source code and data can be obtained from
https://github.com/lennylv/ctP2ISP
.
更多查看译文
关键词
Protein–protein interaction sites,convolution,transformer,data augmentation
AI 理解论文
溯源树
样例
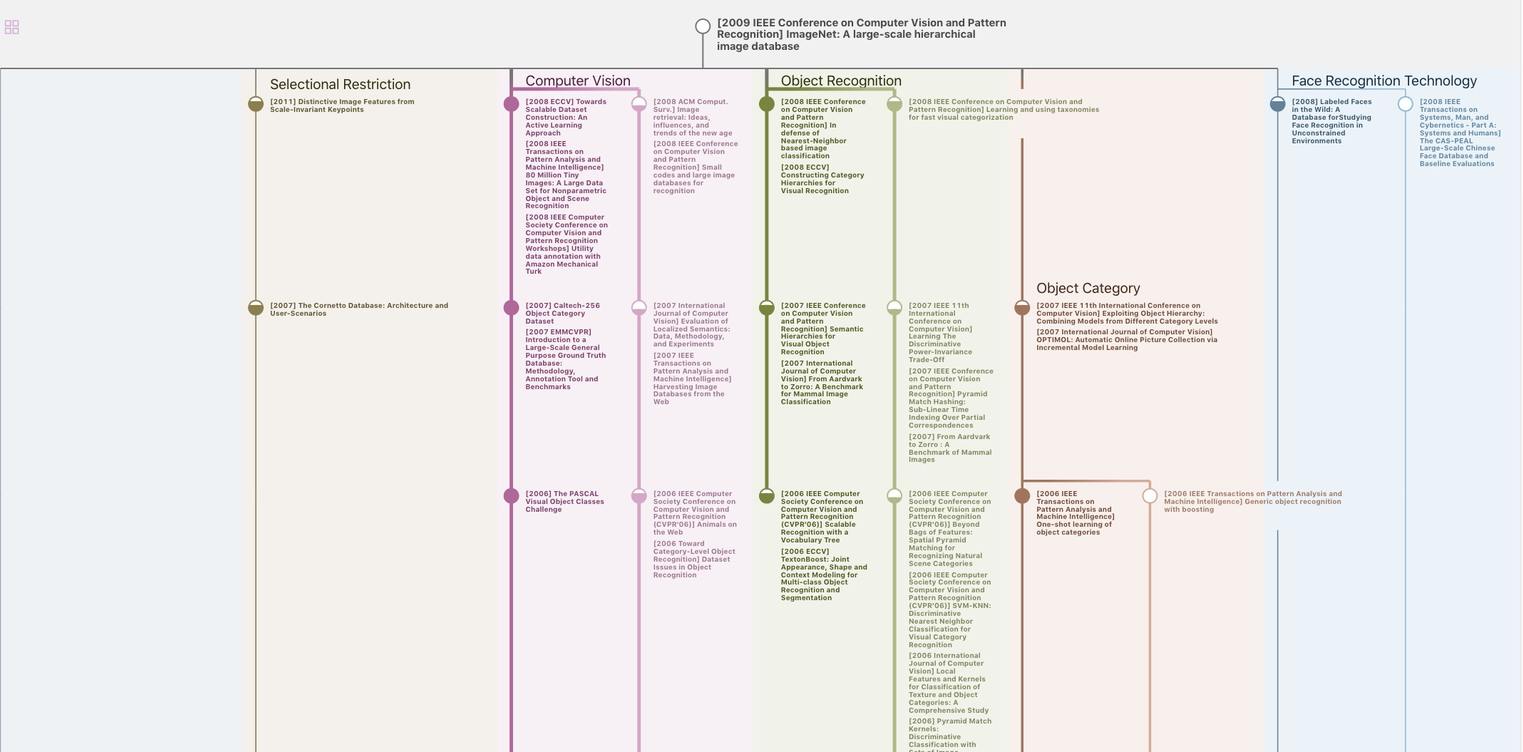
生成溯源树,研究论文发展脉络
Chat Paper
正在生成论文摘要