A Macaque Brain Extraction Model Based on U-Net Combined with Residual Structure
BRAIN SCIENCES(2022)
摘要
Accurately extracting brain tissue is a critical and primary step in brain neuroimaging research. Due to the differences in brain size and structure between humans and nonhuman primates, the performance of the existing tools for brain tissue extraction, working on macaque brain MRI, is constrained. A new transfer learning training strategy was utilized to address the limitations, such as insufficient training data and unsatisfactory model generalization ability, when deep neural networks processing the limited samples of macaque magnetic resonance imaging(MRI). First, the project combines two human brain MRI data modes to pre-train the neural network, in order to achieve faster training and more accurate brain extraction. Then, a residual network structure in the U-Net model was added, in order to propose a ResTLU-Net model that aims to improve the generalization ability of multiple research sites data. The results demonstrated that the ResTLU-Net, combined with the proposed transfer learning strategy, achieved comparable accuracy for the macaque brain MRI extraction tasks on different macaque brain MRI volumes that were produced by various medical centers. The mean Dice of the ResTLU-Net was 95.81% (no need for denoise and recorrect), and the method required only approximately 30-60 s for one extraction task on an NVIDIA 1660S GPU.
更多查看译文
关键词
brain extraction tool, macaque brain MRI, data fusion, residual structure, U-Net application
AI 理解论文
溯源树
样例
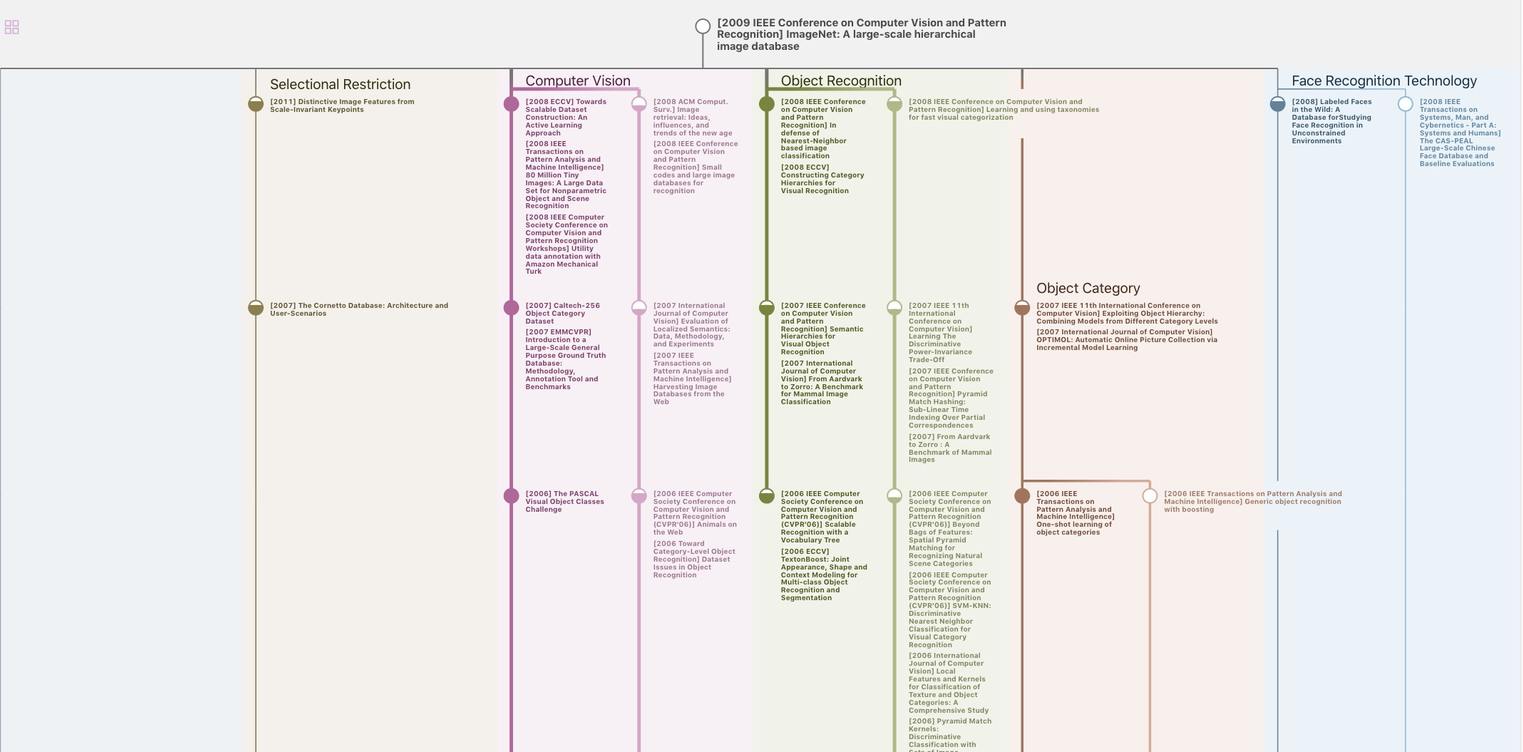
生成溯源树,研究论文发展脉络
Chat Paper
正在生成论文摘要