Unsupervised Domain Adaptation for Remote Sensing Images Using Metric Learning and Correlation Alignment
Communications in Computer and Information ScienceComputer Vision, Pattern Recognition, Image Processing, and Graphics(2020)
摘要
We address the problem of domain adaptation (DA) in the context of remote sensing (RS) image classification in this paper. By definition, the problem of unsupervised DA aims at classifying samples from a target domain which is strictly devoid of any label information while assuming that enough training data are available from a related yet non-identical (in terms of data distributions) source domain. A number of existing approaches in this regard are focused towards matching the underlying distributions of the data from both the domains in a shared latent space without explicitly considering: i) the discriminativeness of the embedding space, ii) the usefulness of a manifold distance is pulling the domains towards each other over the standard Euclidean measures. However, we argue the importance of both the aspects in learning the latent space, particularly for fine-grained classes. Our model jointly optimizes both the terms in an end-to-end fashion and the learned latent space is found to properly align the classes with high precision. Experimental results obtained on a hyper-spectral and a multi-spectral dataset confirm the superior performance of the approach over a number of techniques from the literature.
更多查看译文
关键词
Domain adaptation, Metric learning, Representation learning, Remote sensing.
AI 理解论文
溯源树
样例
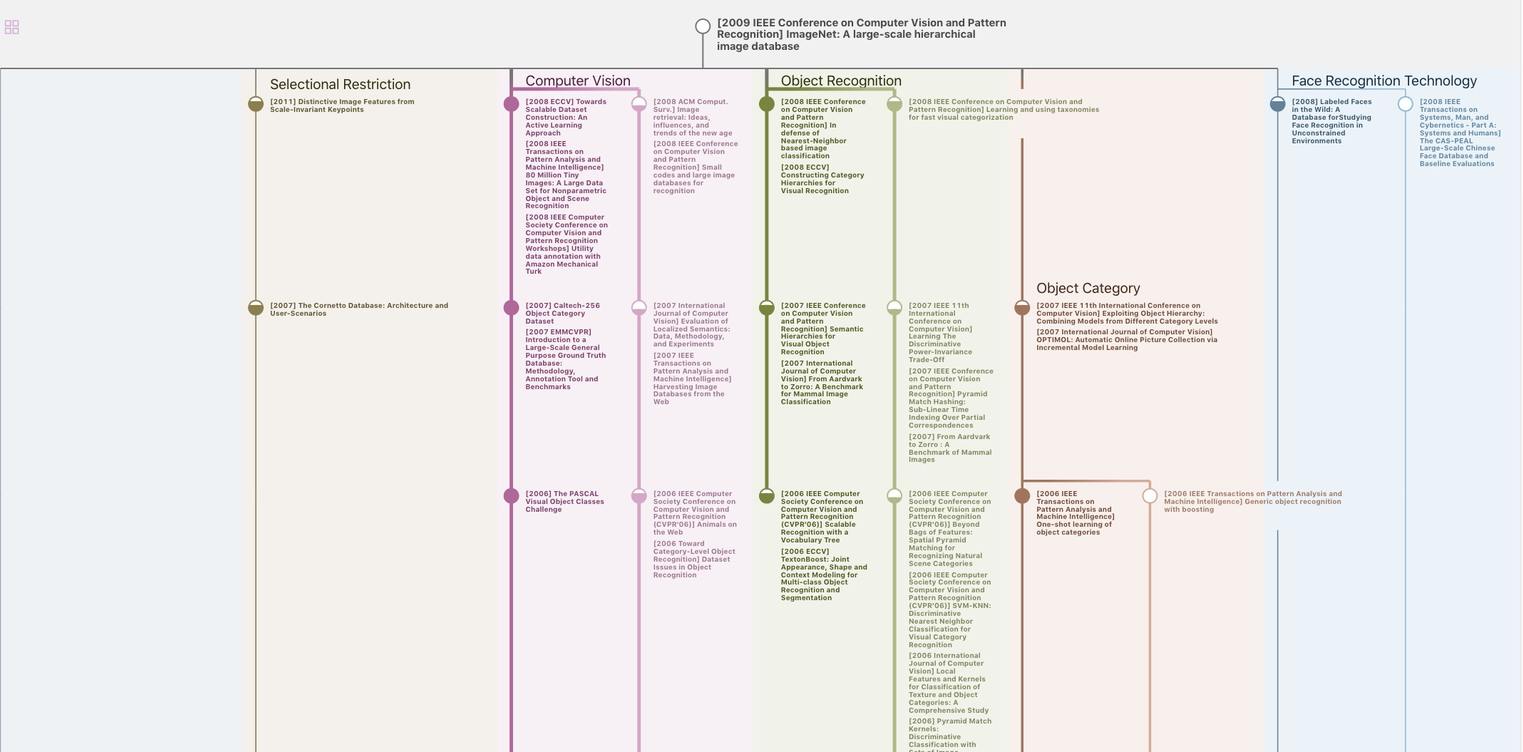
生成溯源树,研究论文发展脉络
Chat Paper
正在生成论文摘要