Towards automating systematic reviews on immunization using an advanced natural language processing–based extraction system
Canada Communicable Disease Report(2020)
摘要
Evidence-informed decision making is based on the premise that the entirety of information on a topic is collected and analyzed. Systematic reviews allow for data from different studies to be rigorously assessed according to PICO principles (population, intervention, control, outcomes). However, conducting a systematic review is generally a slow process that is a significant drain on resources. The fundamental problem is that the current approach to creating a systematic review cannot scale to meet the challenges resulting from the massive body of unstructured evidence. For this reason, the Public Health Agency of Canada has been examining the automation of different stages of evidence synthesis to increase efficiencies. In this article, we present an overview of an initial version of a novel machine learning–based system that is powered by recent advances in natural language processing (NLP), such as BioBERT, with further optimizations completed using a new immunization-specific document database. The resulting optimized NLP model at the core of this system is able to identify and extract PICO-related fields from publications on immunization with an average accuracy of 88% across five classes of text. Functionality is provided through a straightforward web interface.
更多查看译文
关键词
automation,natural language processing,nlp,data extraction,systematic reviews,machine learning
AI 理解论文
溯源树
样例
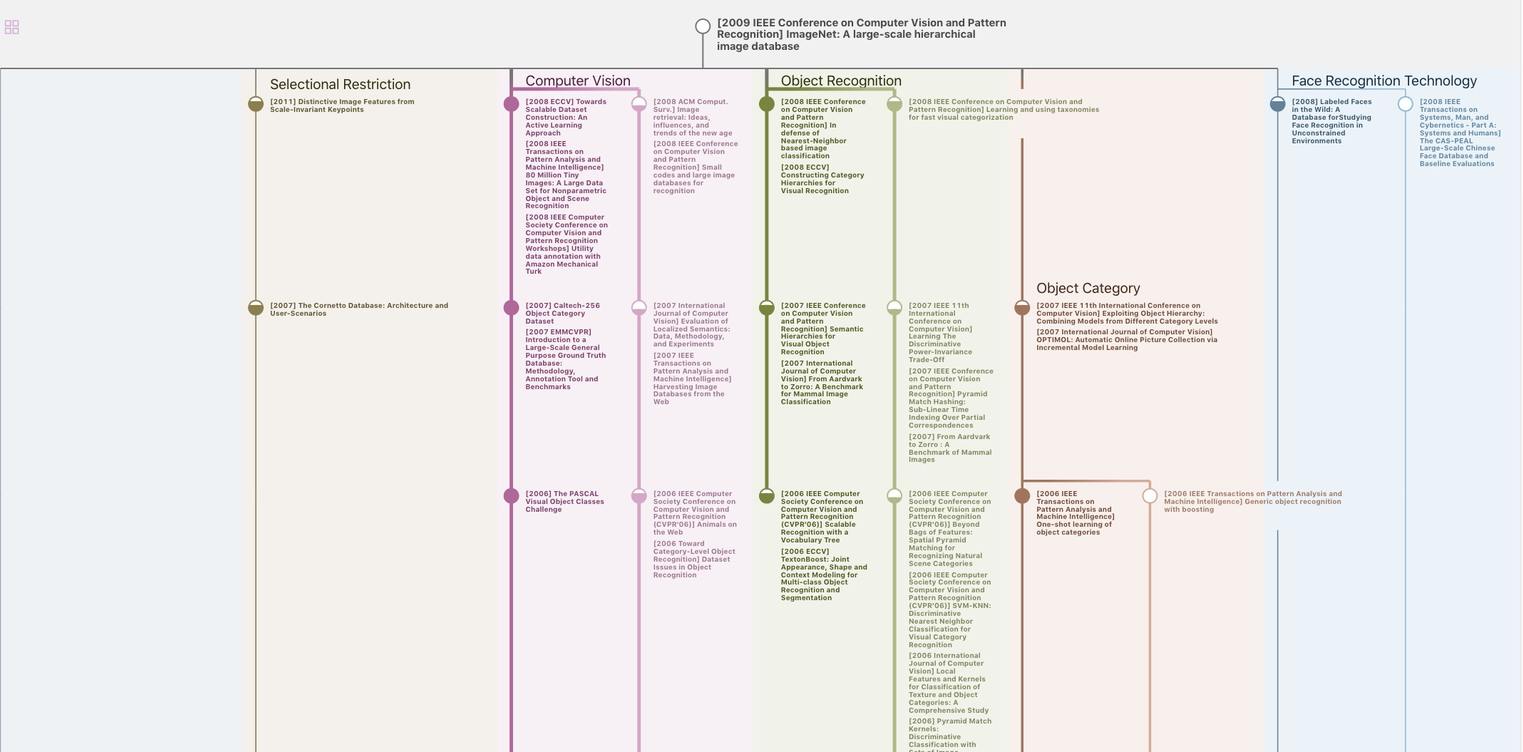
生成溯源树,研究论文发展脉络
Chat Paper
正在生成论文摘要