S-UNet: A Bridge-Style U-Net Framework With a Saliency Mechanism for Retinal Vessel Segmentation
IEEE Access(2019)
摘要
Deep learning methods have been successfully applied in medical image classification, segmentation and detection tasks. The U-Net architecture has been widely applied for these tasks. In this paper, we propose a U-Net variant for improved vessel segmentation in retinal fundus images. Firstly, we design a minimal U-Net (Mi-UNet) architecture, which drastically reduces the parameter count to 0.07M compared to 31.03M for the conventional U-Net. Moreover, based on Mi-UNet, we propose Salient U-Net (S-UNet), a bridge-style U-Net architecture with a saliency mechanism and with only 0.21M parameters. S-UNet uses a cascading technique that employs the foreground features of one net block as the foreground attention information of the next net block. This cascading leads to enhanced input images, inheritance of the learning experience of previous net blocks, and hence effective solution of the data imbalance problem. S-UNet was tested on two benchmark datasets, DRIVE and CHASE_DB1, with image sizes of $584\times 565$ and $960\times 999$ , respectively. S-UNet was tested on the TONGREN clinical dataset with image sizes of $1880\times 2816$ . The experimental results show superior performance in comparison to other state-of-the-art methods. Especially, for whole-image input from the DRIVE dataset, S-UNet achieved a Matthews correlation coefficient (MCC), an area under curve (AUC), and an F1 score of 0.8055, 0.9821, and 0.8303, respectively. The corresponding scores for the CHASE_DB1 dataset were 0.8065, 0.9867, and 0.8242, respectively. Moreover, our model shows an excellent performance on the TONGREN clinical dataset. In addition, S-UNet segments images of low, medium, and high resolutions in just 33ms, 91ms and 0.49s, respectively. This shows the real-time applicability of the proposed model.
更多查看译文
关键词
Deep learning,retinal fundus image,saliency mechanism,vessel segmentation
AI 理解论文
溯源树
样例
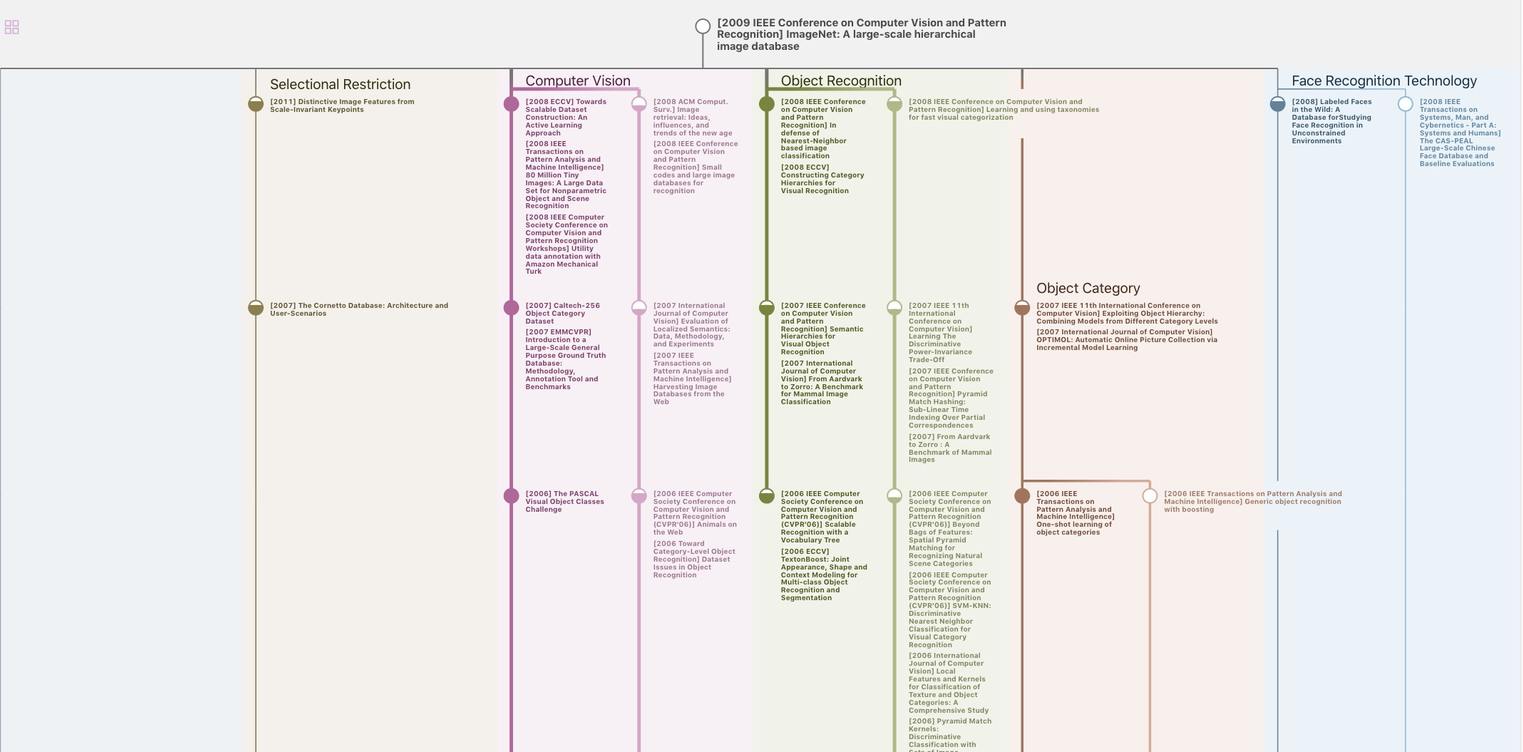
生成溯源树,研究论文发展脉络
Chat Paper
正在生成论文摘要