STRNN: End-to-end deep learning framework for video partial copy detection
Journal of Physics: Conference Series(2019)
摘要
Abstract The task of partial copy detection in videos aims at determine if one or more segments of the query video are already present in the data-set, while giving the information of similar portion time period. At present, most effective algorithms of partial copy detection in videos are designed as three steps: feature extraction, feature matching and time alignment. The separation of feature matching and time alignment module ignores the spatio-temporal information of partial copy to some extent. Therefore, satisfactory performance is not obtained. In order to reduce this loss, this article does not decompose it into two separate tasks, but using a single convolution neural network to solve these two aspects. First, we sample video frames and extract CNN features, calculate the spatio-temporal relationship matrix of the source video and the query video, and then graphically map the matrix and train the convolution neural network based on the object detection task of the RefineDet model. Finally, in the query phase, the time period of the partial copy is deduced based on the detection result. In this paper, we evaluate the performance of the algorithm on the real complex video copy detection data-set VCDB which is significantly improved compared with the state-of-the-art partial copy detection framework.
更多查看译文
AI 理解论文
溯源树
样例
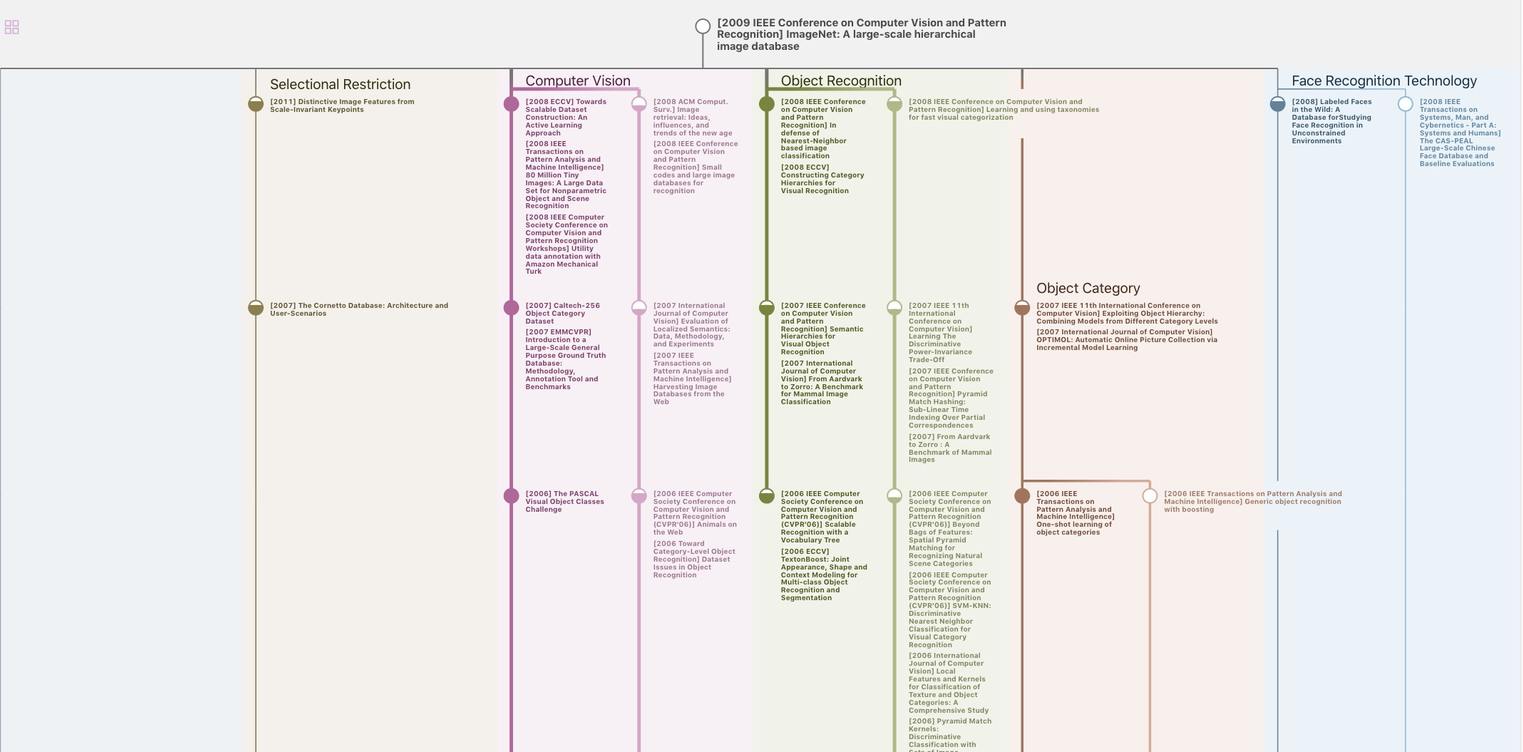
生成溯源树,研究论文发展脉络
Chat Paper
正在生成论文摘要