Hyperspectral Image Classification Using Semi-supervised Random Forest
Proceedings of the International Conference on ISMAC in Computational Vision and Bio-Engineering 2018 (ISMAC-CVB)Lecture Notes in Computational Vision and Biomechanics(2019)
摘要
In this paper, a hyperspectral image classification technique is proposed using semi-supervised random forest (SSRF). Robust node splitting in the random forest requires enormous training data, which is scarce in remote sensing applications. In order to overcome this drawback, we propose utilizing unlabeled data in conjunction with labeled data to assist the splitting process. Moreover, in order to tackle the curse of dimensionality associated with a hyperspectral image, we explore nonnegative matrix factorization (NMF) to remove redundant information. Experimental results confirm the efficacy of the proposed method.
更多查看译文
关键词
Hyperspectral imaging, Semi-supervised learning, Random forest, Nonnegative matrix factorization
AI 理解论文
溯源树
样例
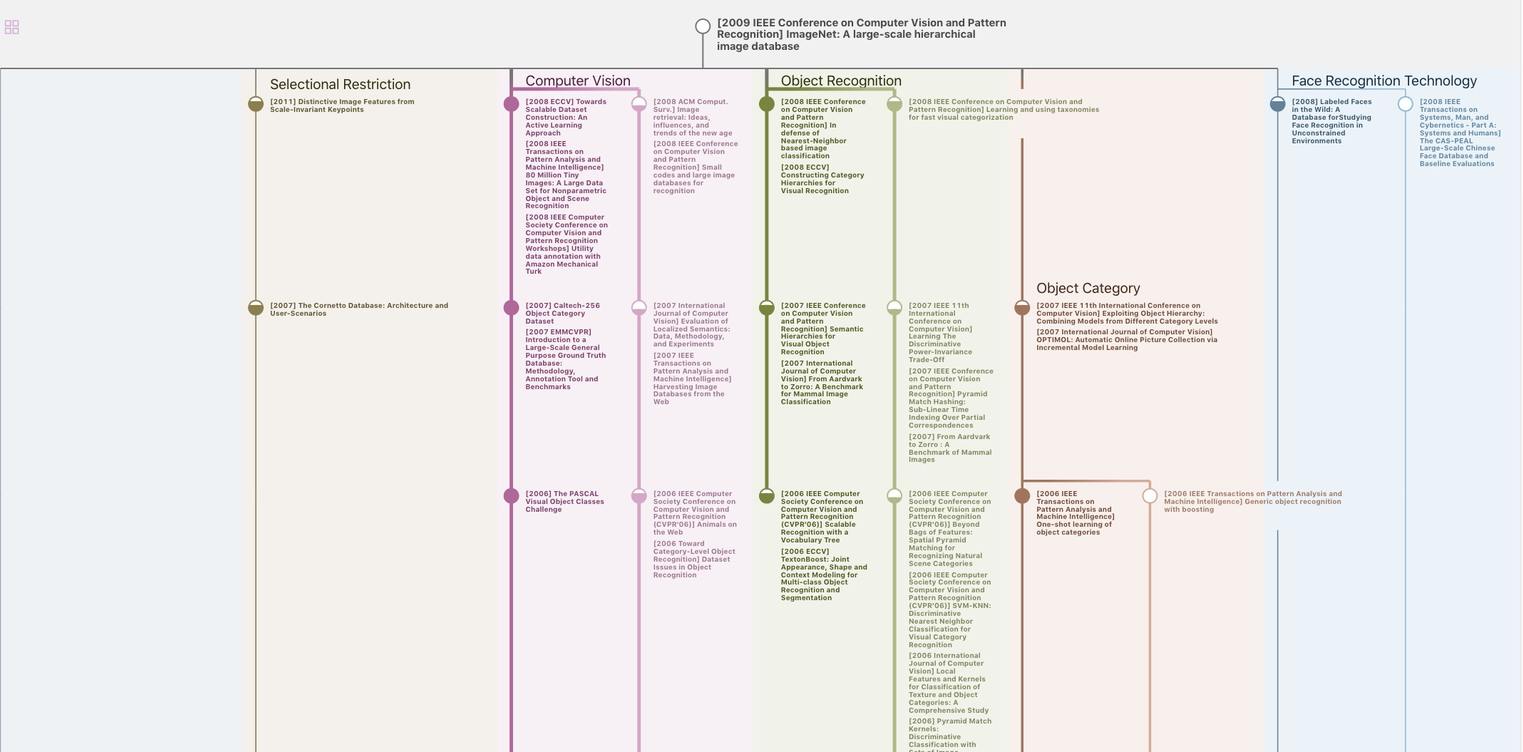
生成溯源树,研究论文发展脉络
Chat Paper
正在生成论文摘要