Seq3seq fingerprint
ACM SIGBioinformatics Record(2018)
摘要
Observing the recent progress in Deep Learning, the employment of AI is surging to accelerate drug discovery and cut R&D costs in the last few years. However, the success of deep learning is attributed to large-scale clean high-quality labeled data, which is generally unavailable in drug discovery practices. In this paper, we address this issue by proposing an end-to-end deep learning framework in a semi-supervised learning fashion. That is said, the proposed deep learning approach can utilize both labeled and unlabeled data. While labeled data is of very limited availability, the amount of available unlabeled data is generally huge. The proposed framework, named as seq3seq fingerprint , automatically learns a strong representation of each molecule in an unsupervised way from a huge training data pool containing a mixture of both unlabeled and labeled molecules. In the meantime, the representation is also adjusted to further help predictive tasks, e.g., acidity, alkalinity or solubility classification. The entire framework is trained end-to-end and simultaneously learn the representation and inference results. Extensive experiments support the superiority of the proposed framework.
更多查看译文
AI 理解论文
溯源树
样例
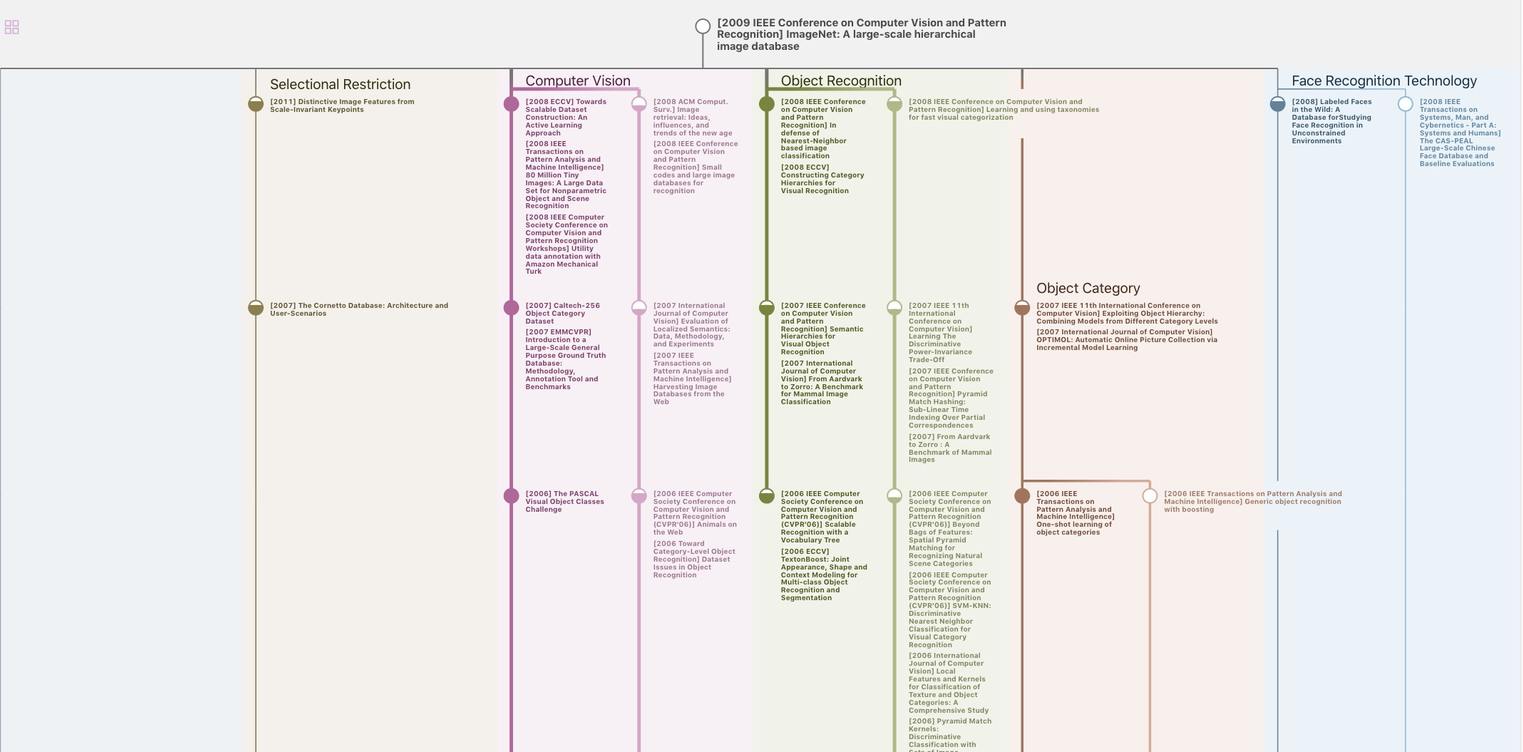
生成溯源树,研究论文发展脉络
Chat Paper
正在生成论文摘要