Deep Recurrent Neural Network DRNN Model for Real-Time Multistage Pumping Data
Day 3 Wed, November 07, 2018(2018)
摘要
Abstract A new real-time model was developed, based on a deep recurrent neural network (DRNN), to predict response variables, such as surface pressure response, during the hydraulic fracturing process. During the stimulation process stage, fluids are inserted at the top of the wellhead, and the flow is driven by the difference between the hydrostatic pressure and reservoir pressure. The major physics and engineering aspects in this process are very complex; quite often, the measured data includes a large amount of uncertainty related to the accuracy of the measured data, as well as intrinsic noise. Consequently, the best approach uses a machine learning-based technique that can resolve both temporal and spatial non-linear variations. The approach followed in this paper provides a long short-term memory (LSTM) network-based method to predict surface pressure in a fracturing job, considering all commonly known surface variables. The surface pumping data consists of real-time data captured within each stage, including surface treating pressure, fluid pumping rate, and proppant rate. The prediction of a response variable, such as the surface pressure response, is important because it provides the basis for decisions made in several oil and gas applications to ensure success, including hydraulic fracturing and matrix acidizing. Currently available modeling methods are limited in that the estimates are not high resolution and cannot address a high level of non-linearity in the treatment pressure time series relationship with other variables, such as flow rate and proppant rate. In addition, these methods cannot predict subsurface variable responses based only on surface variable measurements. The method described in this paper is extended to accommodate the prediction of diverter pressure response. The model presented in this paper uses a deep learning neural network model to predict the surface pressure based on flow rate and proppant rate. This work represents the first attempt to predict (in real time) a response variable, such as surface pressure, during a pumping stage using a memory-preserving recurrent neural network (RNN) variant (for example, LSTM and gated recurrent unit (GRU)). The results show that the LSTM is capable of modeling the surface pressure in a hydraulic fracturing process well. The surface pressure predictions obtained were within 10% of the actual values. The current effort to model surface pressure can be used to simulate response variables in real time, providing engineers with an accurate representation of the conditions in the wellbore and in the reservoir. The current method can overcome the handling of complex physics to provide a reliable, stable, and accurate numerical solution throughout the pumping stages.
更多查看译文
AI 理解论文
溯源树
样例
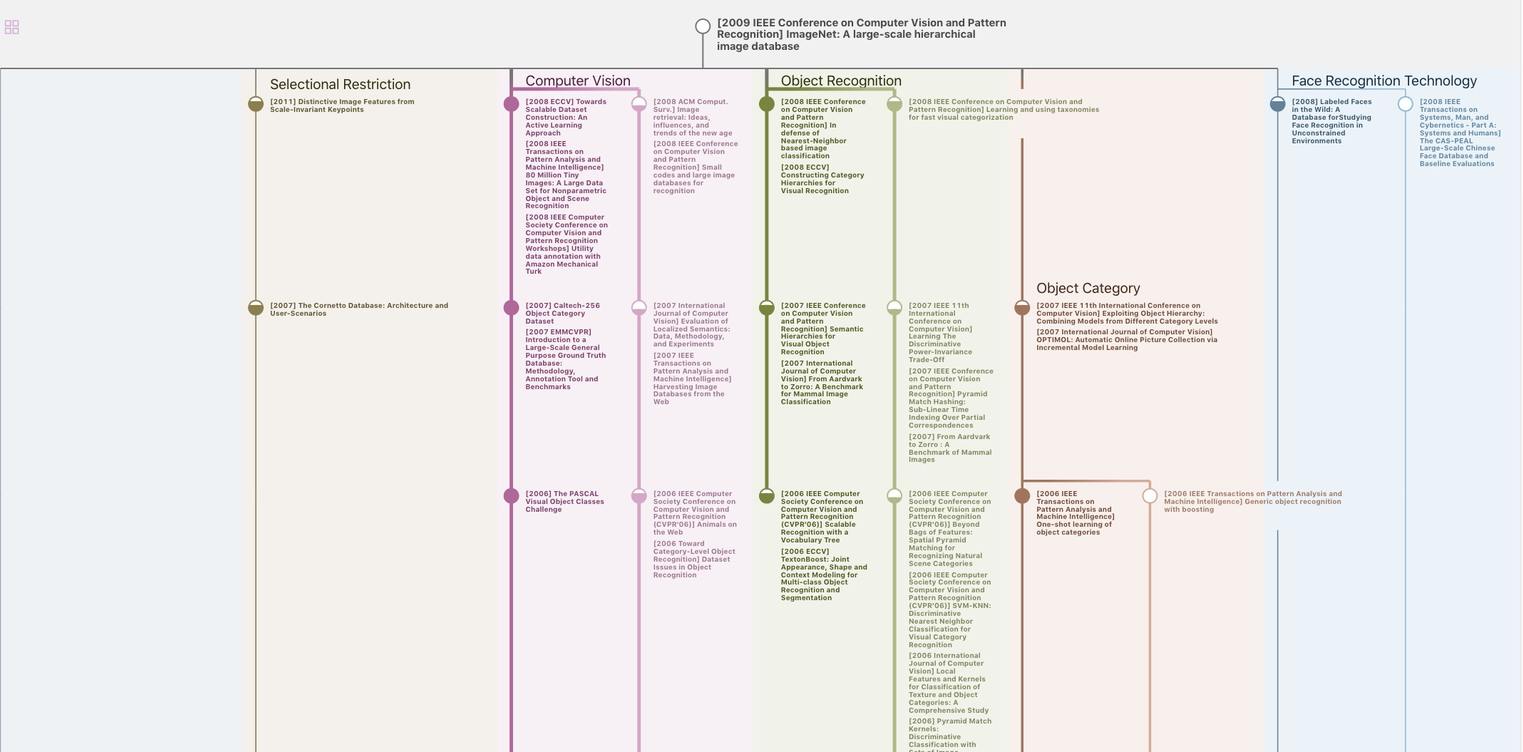
生成溯源树,研究论文发展脉络
Chat Paper
正在生成论文摘要