When Hashes Met Wedges
Proceedings of the 26th International Conference on World Wide Web(2017)
摘要
Finding similar user pairs is a fundamental task in social networks, with numerous applications in ranking and personalization tasks such as link prediction and tie strength detection. A common manifestation of user similarity is based upon network structure: each user is represented by a vector that represents the user's network connections, where pairwise cosine similarity among these vectors defines user similarity. The predominant task for user similarity applications is to discover all similar pairs that have a pairwise cosine similarity value larger than a given threshold $\tau$. In contrast to previous work where $\tau$ is assumed to be quite close to 1, we focus on recommendation applications where $\tau$ is small, but still meaningful. The all pairs cosine similarity problem is computationally challenging on networks with billions of edges, and especially so for settings with small $\tau$. To the best of our knowledge, there is no practical solution for computing all user pairs with, say $\tau = 0.2$ on large social networks, even using the power of distributed algorithms. Our work directly addresses this challenge by introducing a new algorithm --- WHIMP --- that solves this problem efficiently in the MapReduce model. The key insight in WHIMP is to combine the "wedge-sampling" approach of Cohen-Lewis for approximate matrix multiplication with the SimHash random projection techniques of Charikar. We provide a theoretical analysis of WHIMP, proving that it has near optimal communication costs while maintaining computation cost comparable with the state of the art. We also empirically demonstrate WHIMP's scalability by computing all highly similar pairs on four massive data sets, and show that it accurately finds high similarity pairs. In particular, we note that WHIMP successfully processes the entire Twitter network, which has tens of billions of edges.
更多查看译文
AI 理解论文
溯源树
样例
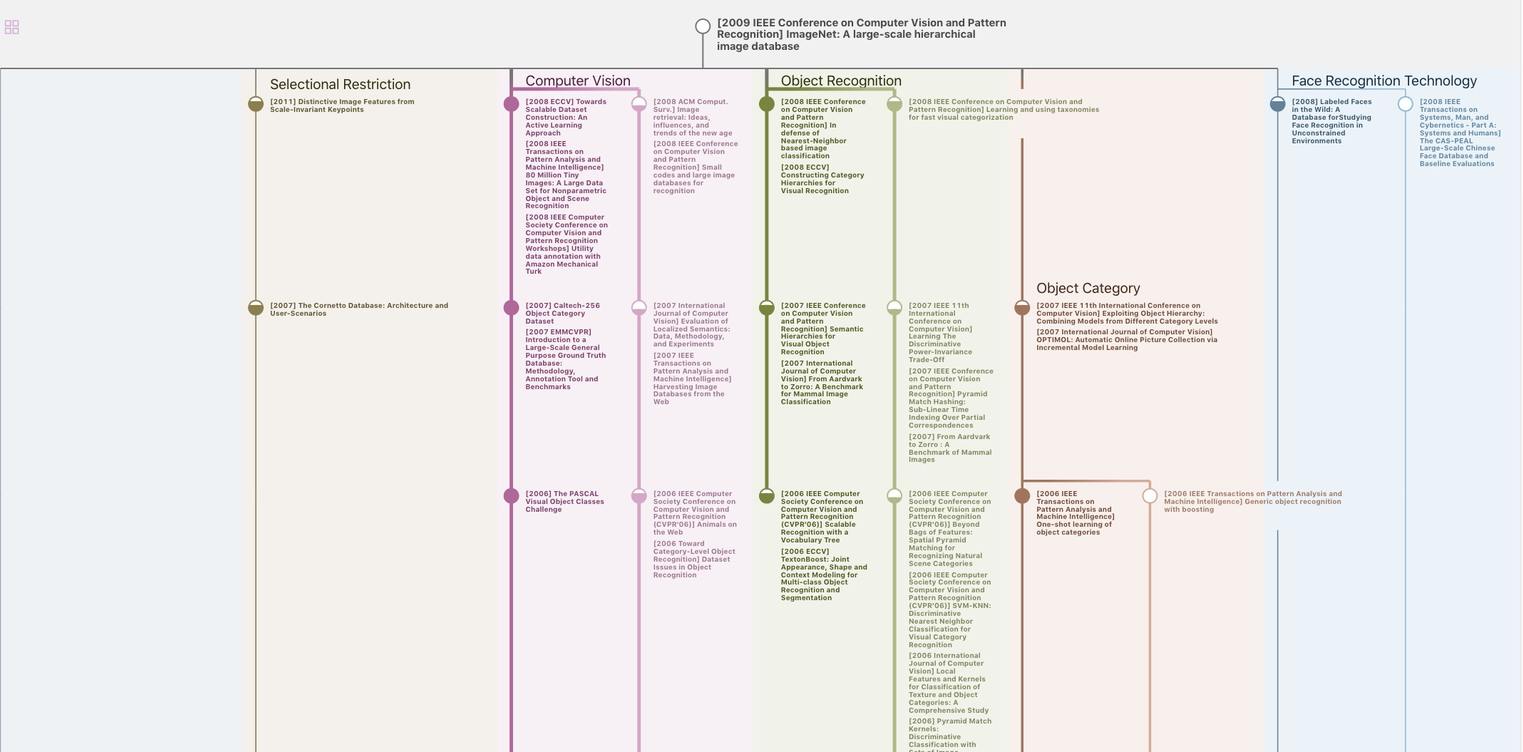
生成溯源树,研究论文发展脉络
Chat Paper
正在生成论文摘要