NeuroView-RNN: It's About Time.
ACM Conference on Fairness, Accountability and Transparency (FAccT)(2022)
摘要
Recurrent Neural Networks (RNNs) are important tools for processing sequential data such as time-series or video. Interpretability is defined as the ability to be understood by a person and is different from explainability, which is the ability to be explained in a mathematical formulation. A key interpretability issue with RNNs is that it is not clear how each hidden state per time step contributes to the decision-making process in a quantitative manner. We propose NeuroView-RNN as a family of new RNN architectures that explains how all the time steps are used for the decision-making process. Each member of the family is derived from a standard RNN architecture by concatenation of the hidden steps into a global linear classifier. The global linear classifier has all the hidden states as the input, so the weights of the classifier have a linear mapping to the hidden states. Hence, from the weights, NeuroView-RNN can quantify how important each time step is to a particular decision. As a bonus, NeuroView-RNN also offers higher accuracy in many cases compared to the RNNs and their variants. We showcase the benefits of NeuroView-RNN by evaluating on a multitude of diverse time-series datasets.
更多查看译文
关键词
neuroview-rnn
AI 理解论文
溯源树
样例
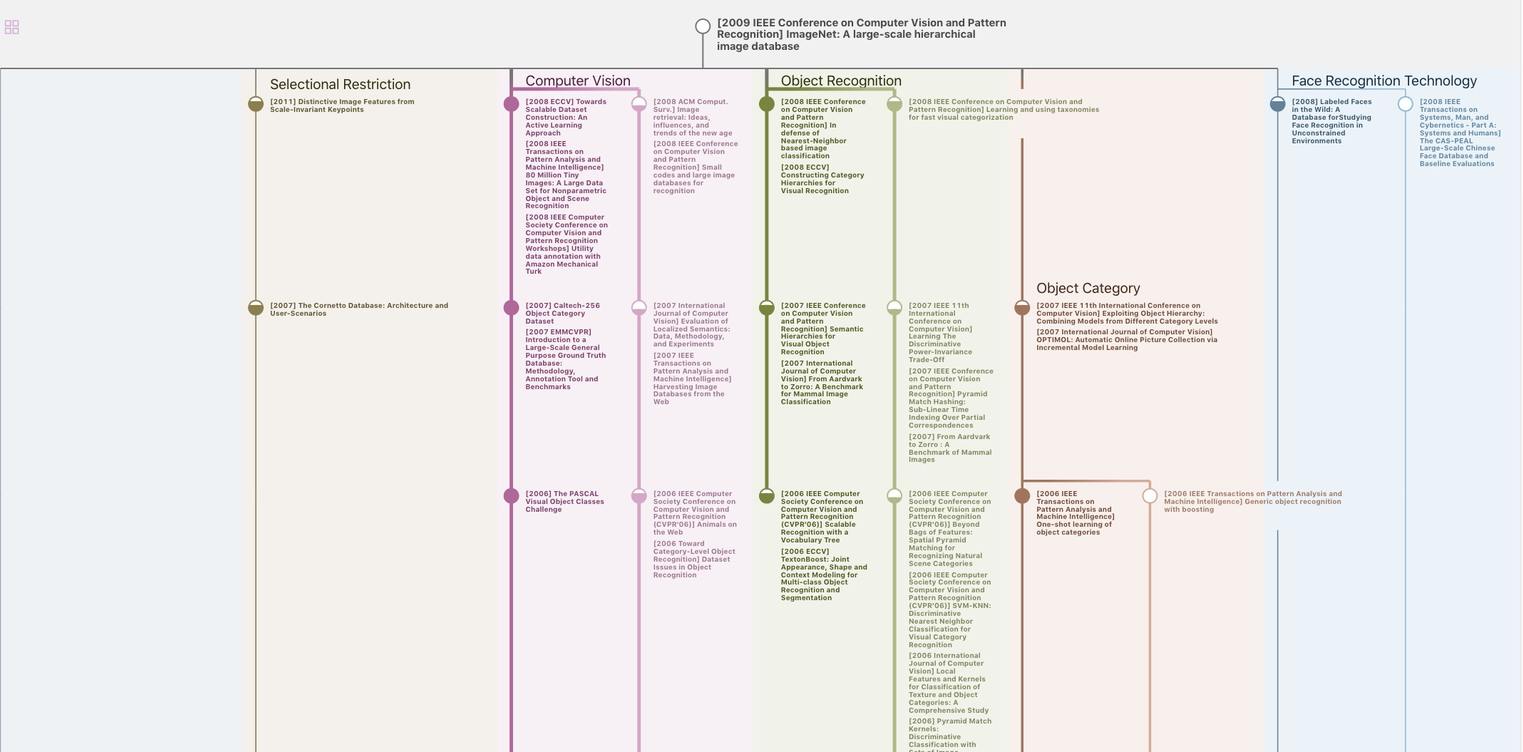
生成溯源树,研究论文发展脉络
Chat Paper
正在生成论文摘要