Indiscriminate Poisoning Attacks on Unsupervised Contrastive Learning.
ICLR 2023(2023)
摘要
Indiscriminate data poisoning attacks are quite effective against supervised learning. However, not much is known about their impact on unsupervised contrastive learning (CL). This paper is the first to consider indiscriminate poisoning attacks of contrastive learning. We propose contrastive poisoning, the first effective such attack on CL. We empirically show that contrastive poisoning, not only drastically reduces the performance of CL algorithms, but also attacks supervised learning models, making it the most generalizable indiscriminate poisoning attack. We also show that CL algorithms with a momentum encoder are more robust to indiscriminate poisoning, and propose a new countermeasure based on matrix completion.
更多查看译文
关键词
data poisoning,contrastive learning
AI 理解论文
溯源树
样例
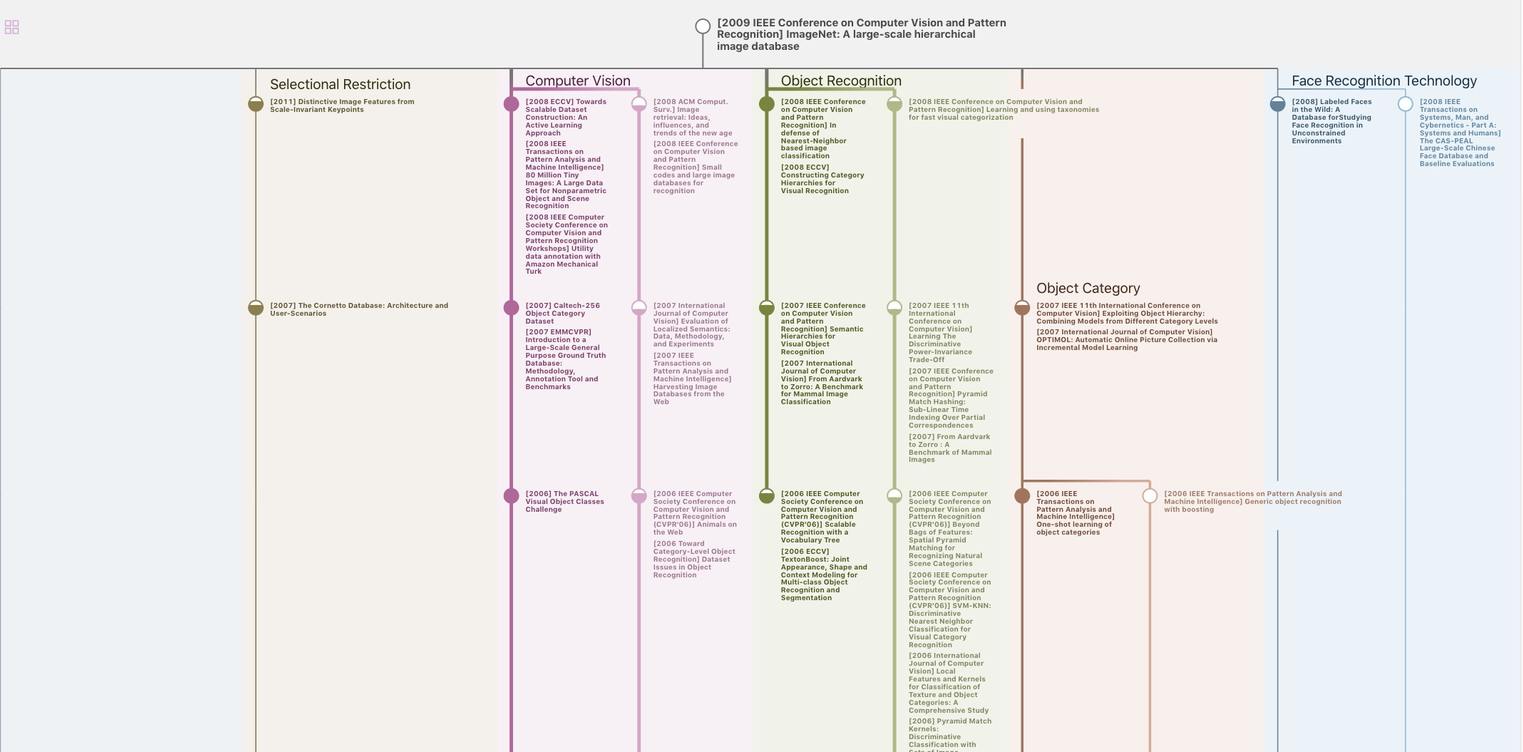
生成溯源树,研究论文发展脉络
Chat Paper
正在生成论文摘要