An adaptive learning and control framework based on dynamic movement primitives with application to human–robot handovers
Robotics and Autonomous Systems(2022)
摘要
Object handover is a fundamental skill needed in many human–robot collaboration tasks ranging from industrial manipulation to daily service. It remains challenging for robots to perform a handover as flexibly and fluently as a human. This article proposes a framework based on Dynamic Movement Primitives (DMP) that enables robot to learn from human demonstrations and transfer the skill into human–robot handovers. In particular, we focus on the problem of dealing with time varying handover locations. Compared to the conventional DMP formalism, the proposed method contains the following extensions: (1) uncertainty-aware learning with Gaussian Process, (2) a weighting function to control the transition of the shape and goal attraction terms, (3) an orientation-based spatial scaling, (4) online parameter adaption with human feedback. Moreover, inspired by the principle of cooperative DMPs, we present an equivalent model to study the interactive dynamics in human–robot handovers. The proposed framework has been validated in experiments and evaluated by both subjective and objective metrics. Results show an enhancement of success rate, fluency and human comfort.
更多查看译文
关键词
Dynamic movement primitives,Human–robot handovers,Adaptive control,Interaction model
AI 理解论文
溯源树
样例
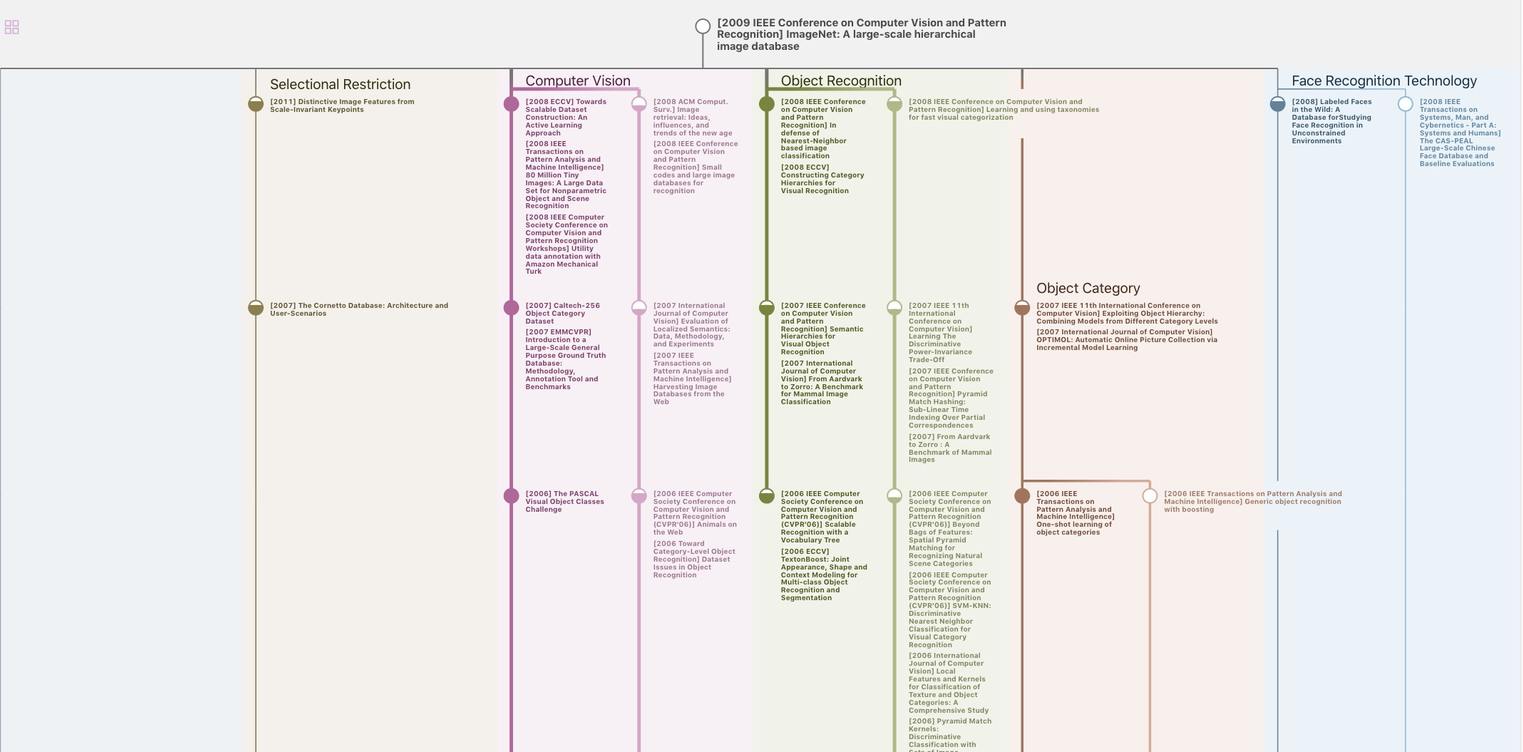
生成溯源树,研究论文发展脉络
Chat Paper
正在生成论文摘要