TASR: Adversarial learning of topic-agnostic stylometric representations for informed crisis response through social media
Information Processing & Management(2022)
摘要
The impact of crisis events can be devastating in a multitude of ways, many of which are unpredictable due to the suddenness in which they occur. The evolution of social media (for example Twitter) has given directly affected individuals or those with valuable information a platform to effectively share their stories to the masses. As a result, these platforms have become vast repositories of helpful information for emergency organizations. However, different crisis events often contain event-specific keywords, which results in the difficult extraction of useful information with a single model. In this paper, we put forward TASR, which stands for Topic-Agnostic Stylometric Representations, a novice deep learning architecture that uses stylometric and adversarial learning to remove topical bias to better manage the unknown surrounding unseen events. As an alternative to domain adaptive approaches requiring data from the unseen event, it reduces the work for those responding to the onset of a crisis. Overall, we conduct a comprehensive study of the situational properties of TASR, the benefits of its architecture including its topic-agnostic and explainable properties, and how it improves upon comparable models in past research. From two experiments, on average, TASR is able to outperform state-of-the-art methods such as transfer learning and domain adoption by 11% in AUC. The ablation study illustrates how different architecture choices of TASR impact the results and that TASR has been optimized for this task. Finally, we conduct a case study to show that explainable results from our model can be used to help guide human analysts through crisis information extraction.
更多查看译文
关键词
Crisis informatics,Adversarial learning,Stylometric representation,Explainability
AI 理解论文
溯源树
样例
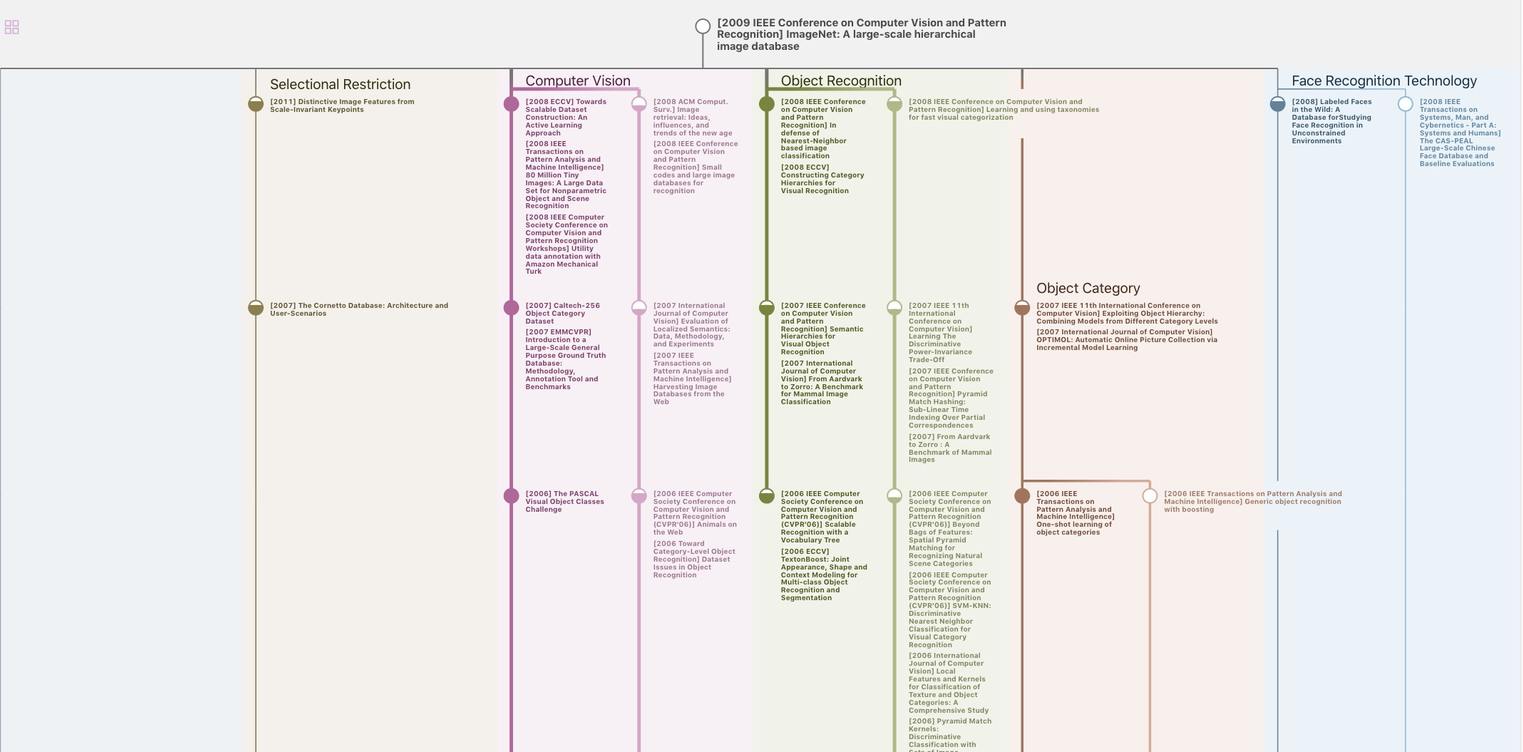
生成溯源树,研究论文发展脉络
Chat Paper
正在生成论文摘要