A directed graph convolutional neural network for edge-structured signals in link-fault detection
Pattern Recognition Letters(2022)
摘要
The growing interest in graph deep learning has led to a surge of research focusing on learning various characteristics of graph-structured data. Directed graphs have generally been treated as incidental to definitions on the more general class of undirected graphs. The implicit class imbalance in some graph problems also proves difficult to tackle. Moreover, a body of work has begun to grow that considers how to learn signals structured on the edges of graphs. In this paper, we propose the directed graph convolutional neural network (DGCNN), and describe a simple way to mitigate the inherent class imbalance in graphs. The model is applied to edge-structured signals from datacenter simulations using the structure of a directed linegraph to represent the second-order structure of its underlying graph. We demonstrate that the DGCNN's improves over undirected models and other directed models by applying our model to locating link-faults in a datacenter simulation. (c) 2021 Elsevier B.V. All rights reserved.
更多查看译文
关键词
Graph deep learning,Datacenter,Directed graph,Edge signals,Graph edge learning,Linegraphs,Directed linegraphs,Graph convolution
AI 理解论文
溯源树
样例
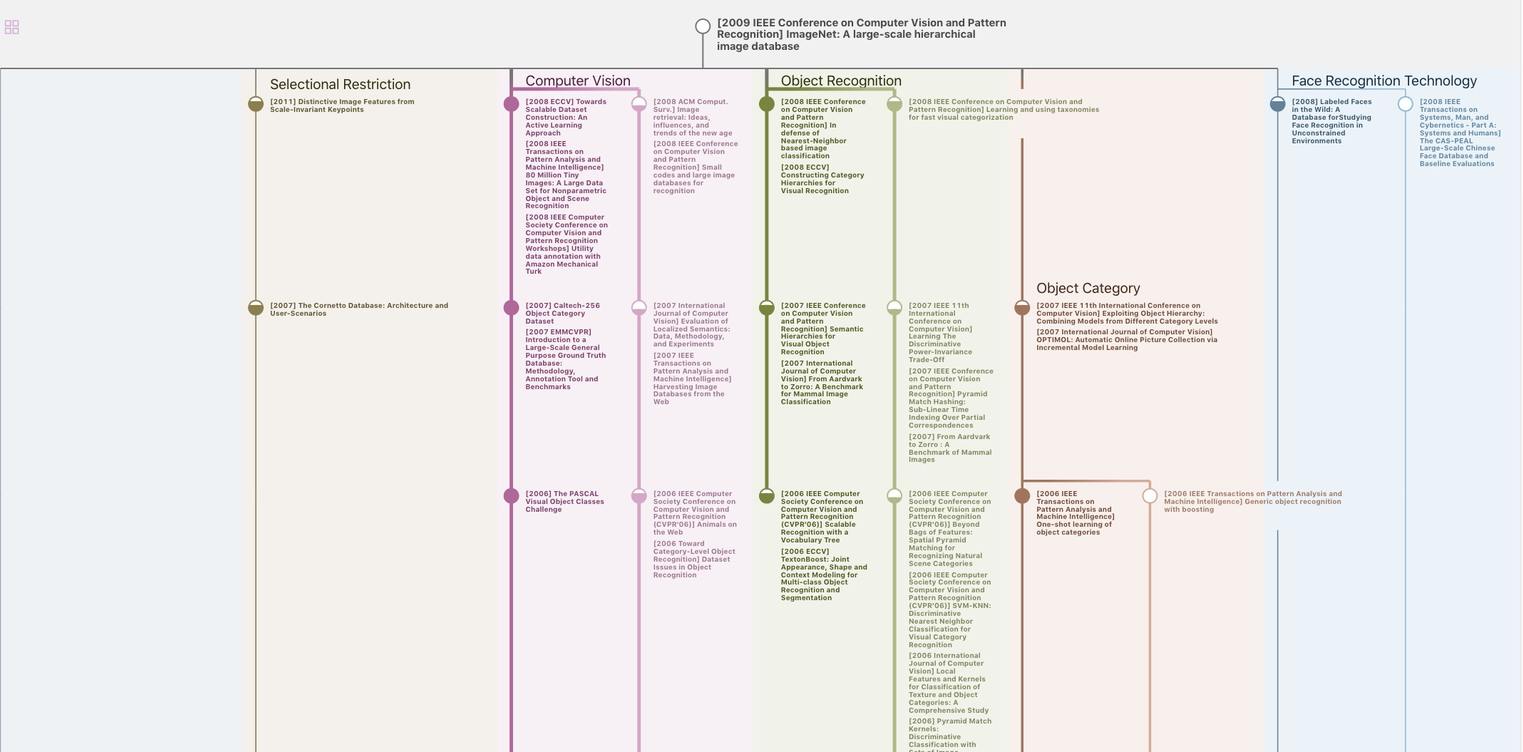
生成溯源树,研究论文发展脉络
Chat Paper
正在生成论文摘要