H.264 and H.265 video traffic modeling using neural networks
COMPUTER COMMUNICATIONS(2022)
摘要
As video has become the dominant type of traffic over wired and wireless networks, the efficient transmission of video streams is of paramount importance. Hence, especially for wireless networks, the optimum utilization of the available bandwidth while preserving the users' Quality of Service and Quality of Experience requirements is crucial. Towards this goal, the accurate prediction of upcoming video frame sizes can play a significant role. This work focuses on achieving such an accurate prediction for videos encoded with H.264 and H.265, which are the major state-of-the-art standards based on their current market share. Unlike previous studies, we use single-step and multi-step approaches to capture the long-range dependence and short-range dependence properties of variable bit rate video traces through neural networks-based modeling. We evaluate the accuracy of Long Short Term Memory, Convolutional Neural Networks and Sequence-to-Sequence models and compare them with existing approaches. Our models show significantly higher accuracy for a variety of videos. We also provide a case study on how our model can be used for traffic policing purposes.
更多查看译文
关键词
Video Streaming, Bandwidth prediction, Traffic modeling, Neural networks
AI 理解论文
溯源树
样例
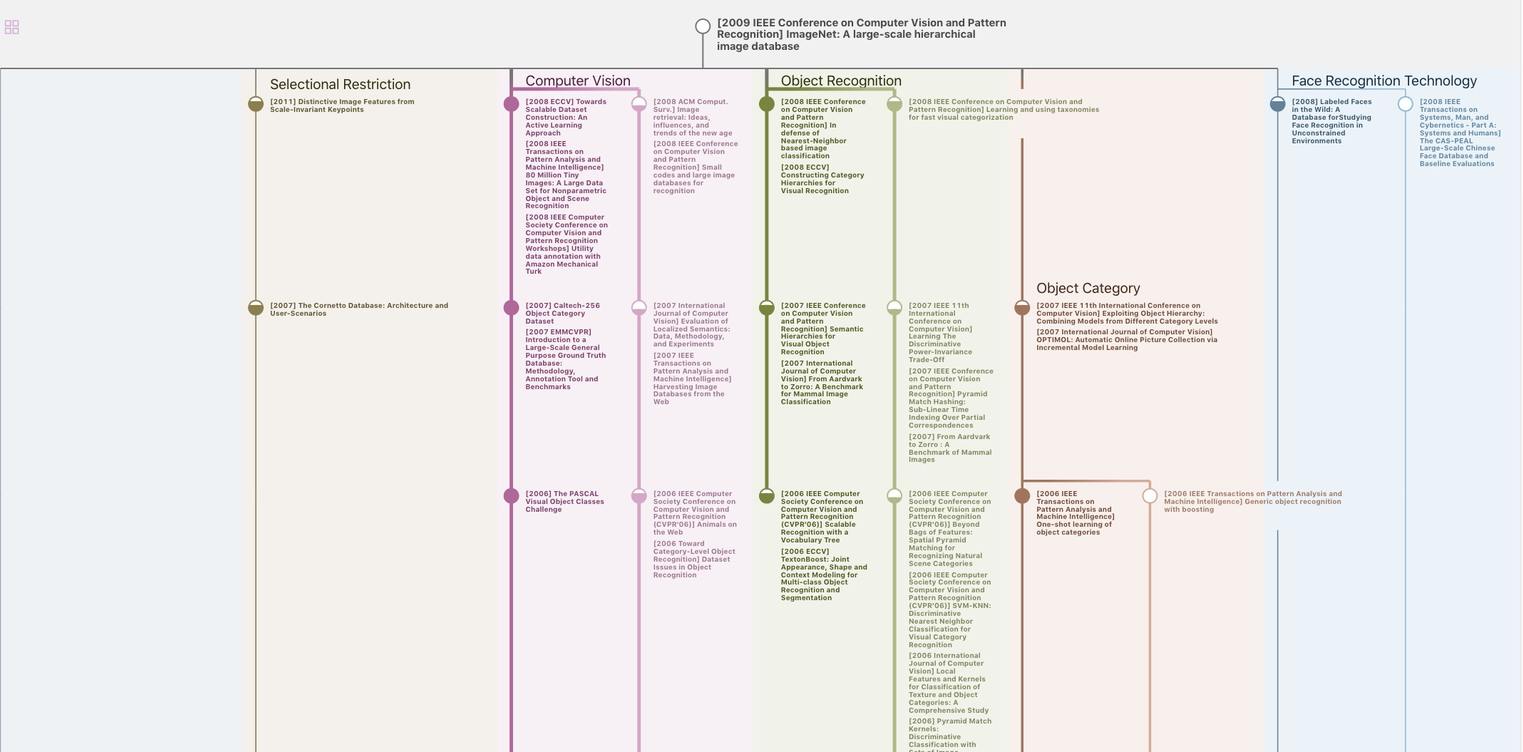
生成溯源树,研究论文发展脉络
Chat Paper
正在生成论文摘要