Rice Mapping in Training Sample Shortage Regions Using a Deep Semantic Segmentation Model Trained on Pseudo-Labels
REMOTE SENSING(2022)
摘要
A deep semantic segmentation model-based method can achieve state-of-the-art accuracy and high computational efficiency in large-scale crop mapping. However, the model cannot be widely used in actual large-scale crop mapping applications, mainly because the annotation of ground truth data for deep semantic segmentation model training is time-consuming. At the operational level, it is extremely difficult to obtain a large amount of ground reference data by photointerpretation for the model training. Consequently, in order to solve this problem, this study introduces a workflow that aims to extract rice distribution information in training sample shortage regions, using a deep semantic segmentation model (i.e., U-Net) trained on pseudo-labels. Based on the time series Sentinel-1 images, Cropland Data Layer (CDL) and U-Net model, the optimal multi-temporal datasets for rice mapping were summarized, using the global search method. Then, based on the optimal multi-temporal datasets, the proposed workflow (a combination of K-Means and random forest) was directly used to extract the rice-distribution information of Jiangsu (i.e., the K-RF pseudo-labels). For comparison, the optimal well-trained U-Net model acquired from Arkansas (i.e., the transfer model) was also transferred to Jiangsu to extract local rice-distribution information (i.e., the TF pseudo-labels). Finally, the pseudo-labels with high confidences generated from the two methods were further used to retrain the U-Net models, which were suitable for rice mapping in Jiangsu. For different rice planting pattern regions of Jiangsu, the final results showed that, compared with the U-Net model trained on the TF pseudo-labels, the rice area extraction errors of pseudo-labels could be further reduced by using the U-Net model trained on the K-RF pseudo-labels. In addition, compared with the existing rule-based rice mapping methods, he U-Net model trained on the K-RF pseudo-labels could robustly extract the spatial distribution information of rice. Generally, this study could provide new options for applying a deep semantic segmentation model to training sample shortage regions.
更多查看译文
关键词
Sentinel-1, rice mapping, K-Means algorithm, random forest classifier, pseudo-label, U-Net
AI 理解论文
溯源树
样例
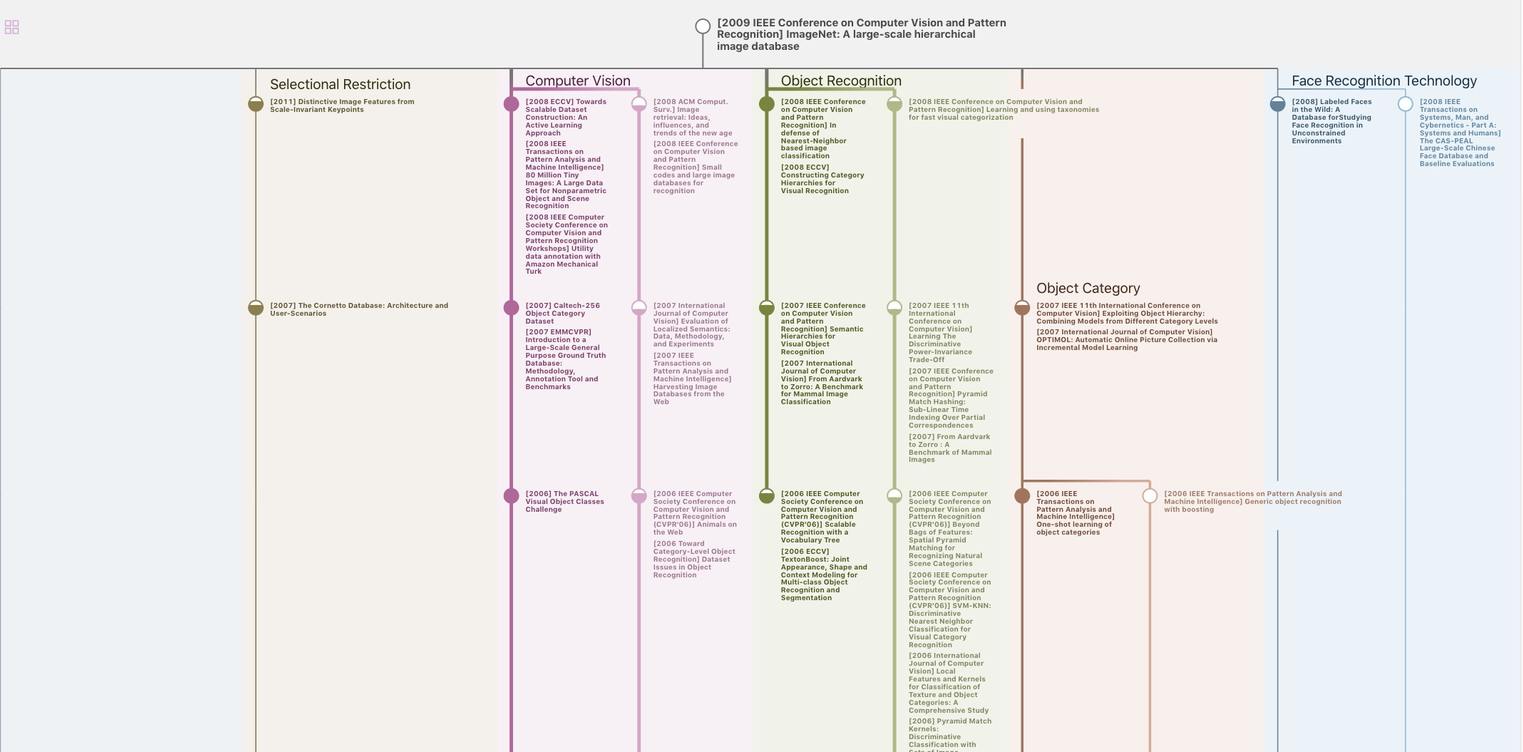
生成溯源树,研究论文发展脉络
Chat Paper
正在生成论文摘要