On Uncertainty Estimation by Tree-based Surrogate Models in Sequential Model-based Optimization
INTERNATIONAL CONFERENCE ON ARTIFICIAL INTELLIGENCE AND STATISTICS, VOL 151(2022)
摘要
Sequential model-based optimization sequentially selects a candidate point by constructing a surrogate model with the history of evaluations, to solve a black-box optimization problem. Gaussian process (GP) regression is a popular choice as a surrogate model, because of its capability of calculating prediction uncertainty analytically. On the other hand, an ensemble of randomized trees is another option and has practical merits over GPs due to its scalability and easiness of handling continuous/discrete mixed variables. In this paper we revisit various ensembles of randomized trees to investigate their behavior in the perspective of prediction uncertainty estimation. Then, we propose a new way of constructing an ensemble of randomized trees, referred to as BwO forest, where bagging with oversampling is employed to construct boot-strapped samples that are used to build randomized trees with random splitting. Experimental results demonstrate the validity and good performance of BwO forest over existing tree-based models in various circumstances.
更多查看译文
关键词
uncertainty estimation,surrogate models,optimization,tree-based,model-based
AI 理解论文
溯源树
样例
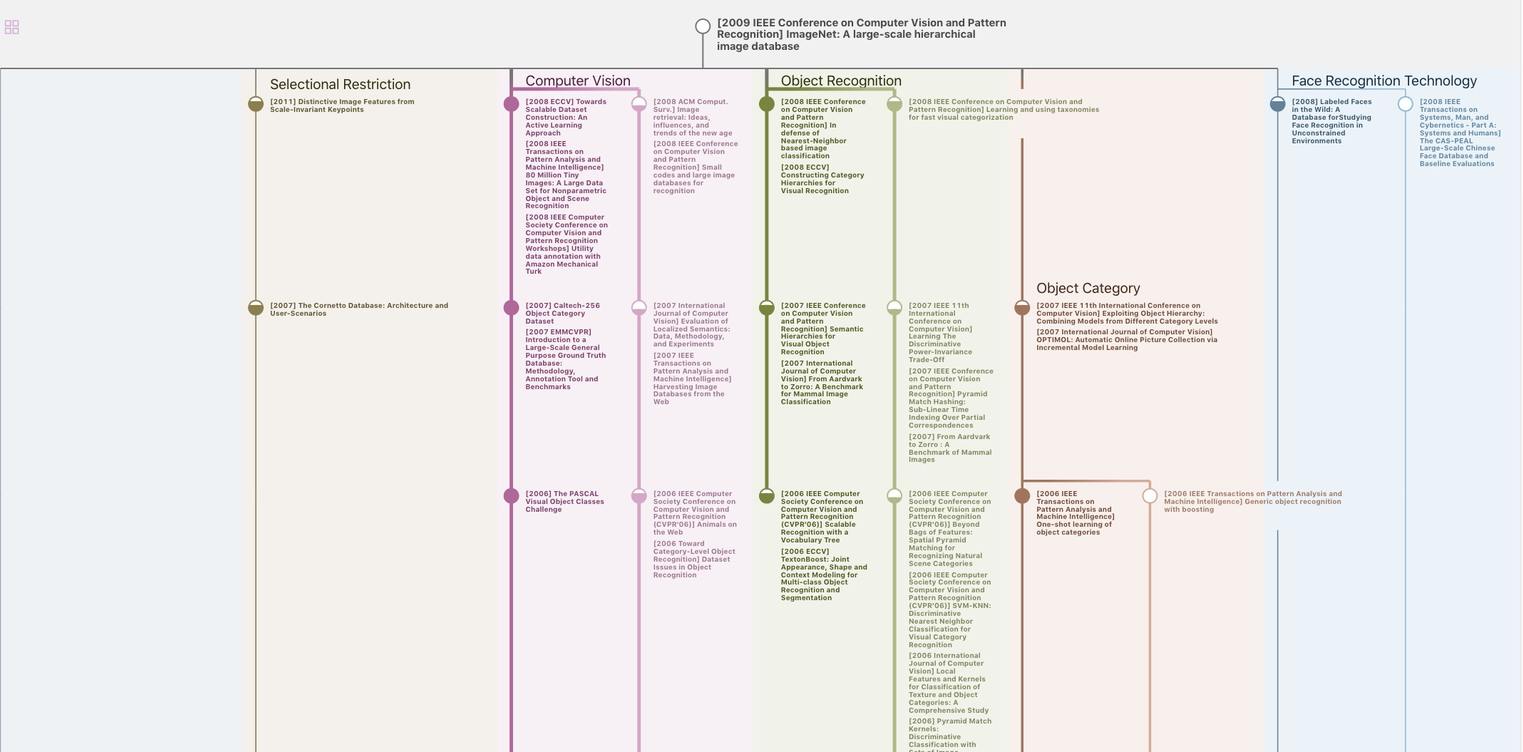
生成溯源树,研究论文发展脉络
Chat Paper
正在生成论文摘要