Clinical-BERT: Vision-Language Pre-training for Radiograph Diagnosis and Reports Generation.
AAAI Conference on Artificial Intelligence(2022)
摘要
In this paper, we propose a vision-language pre-training model, Clinical-BERT, for the medical domain, and devise three domain-specific tasks: Clinical Diagnosis (CD), Masked MeSH Modeling (MMM), Image-MeSH Matching (IMM), together with one general pre-training task: Masked Language Modeling (MLM), to pre-train the model. The CD task helps the model to learn medical domain knowledge by predicting disease from radiographs. Medical Subject Headings (MeSH) words are important semantic components in radiograph reports, and the MMM task helps the model focus on the prediction of MeSH words. The IMM task helps the model learn the alignment of MeSH words with radiographs by matching scores obtained by a two-level sparse attention: region sparse attention and word sparse attention. Region sparse attention generates corresponding visual features for each word, and word sparse attention enhances the contribution of images-MeSH matching to the matching scores. To the best of our knowledge, this is the first attempt to learn domain knowledge during pre-training for the medical domain. We evaluate the pre-training model on Radiograph Diagnosis and Reports Generation tasks across four challenging datasets: MIMIC-CXR, IU X-Ray, COV-CTR, and NIH, and achieve state-of-the-art results for all the tasks, which demonstrates the effectiveness of our pre-training model.
更多查看译文
关键词
Computer Vision (CV)
AI 理解论文
溯源树
样例
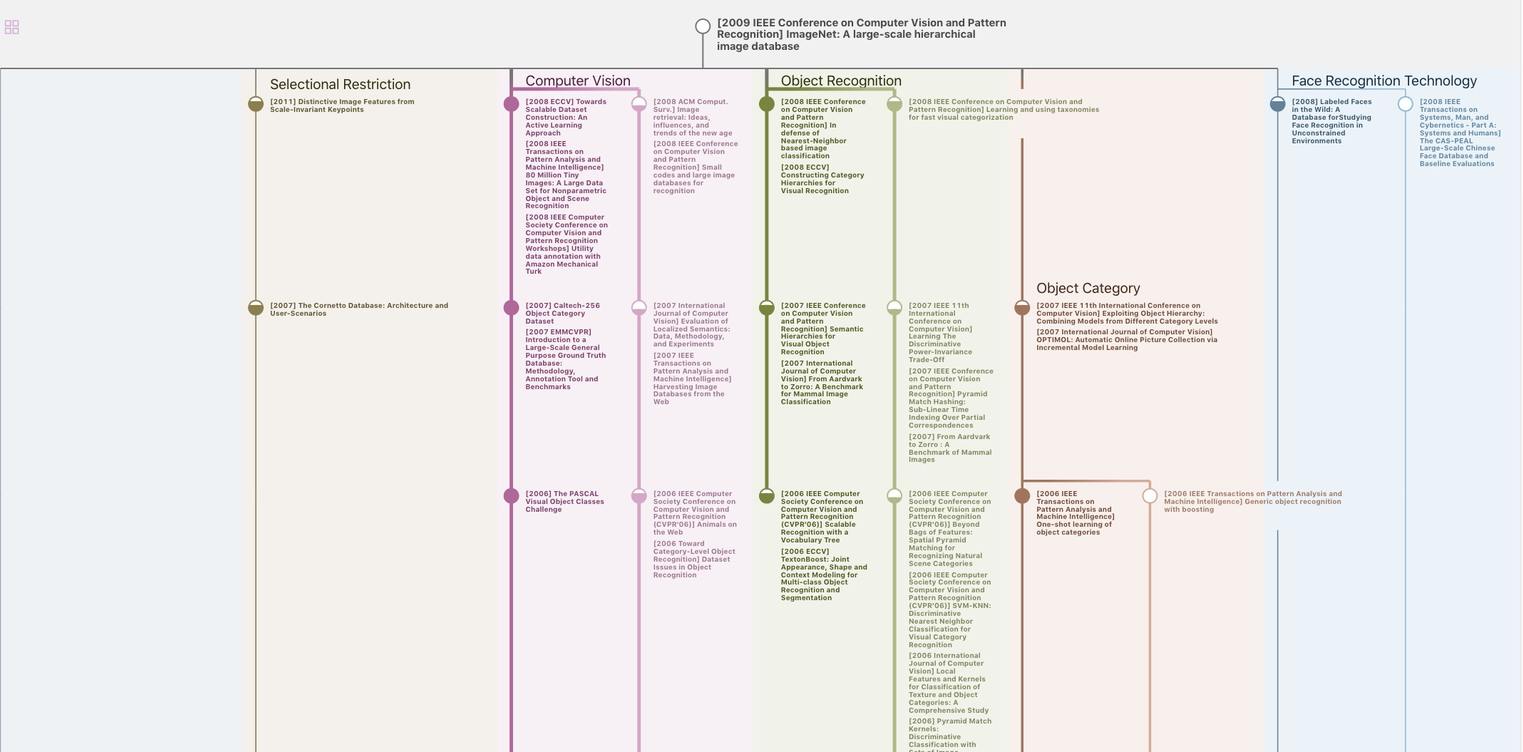
生成溯源树,研究论文发展脉络
Chat Paper
正在生成论文摘要