UAMNer: uncertainty-aware multimodal named entity recognition in social media posts
Applied Intelligence(2021)
摘要
Named Entity Recognition (NER) on social media is a challenging task, as social media posts are usually short and noisy. Recently, some work explores different ways to incorporate the visual information from the image to improve NER on social media and achieves great success. However, existing methods ignore a common scenario on social media—the image sometimes does not match the posted text. Thus, the irrelevant images may introduce noisy information in existing models. In this paper, a novel uncertainty-aware framework for multimodal NER (UAMNer) on social media is put forward, which combines visual features with text when the text information is insufficient, thus suppressing noisy information from the irrelevant images. Specifically, we propose a two-stage label refinement framework for multimodal NER in social media posts. Given a multimodal post, we first use a bayesian neural network to produce candidate labels from the text. If the candidate labels have high uncertainty, we then use a multimodal transformer to refine the label with textual and visual features. We experiment on two public datasets, namely Twitter-2015 and Twitter-2017. The proposed method achieves better performance compared with the state-of-the-art methods.
更多查看译文
关键词
Multimodal named entity recognition, Multimodal social media, Bayesian neural network, Multimodal transformer
AI 理解论文
溯源树
样例
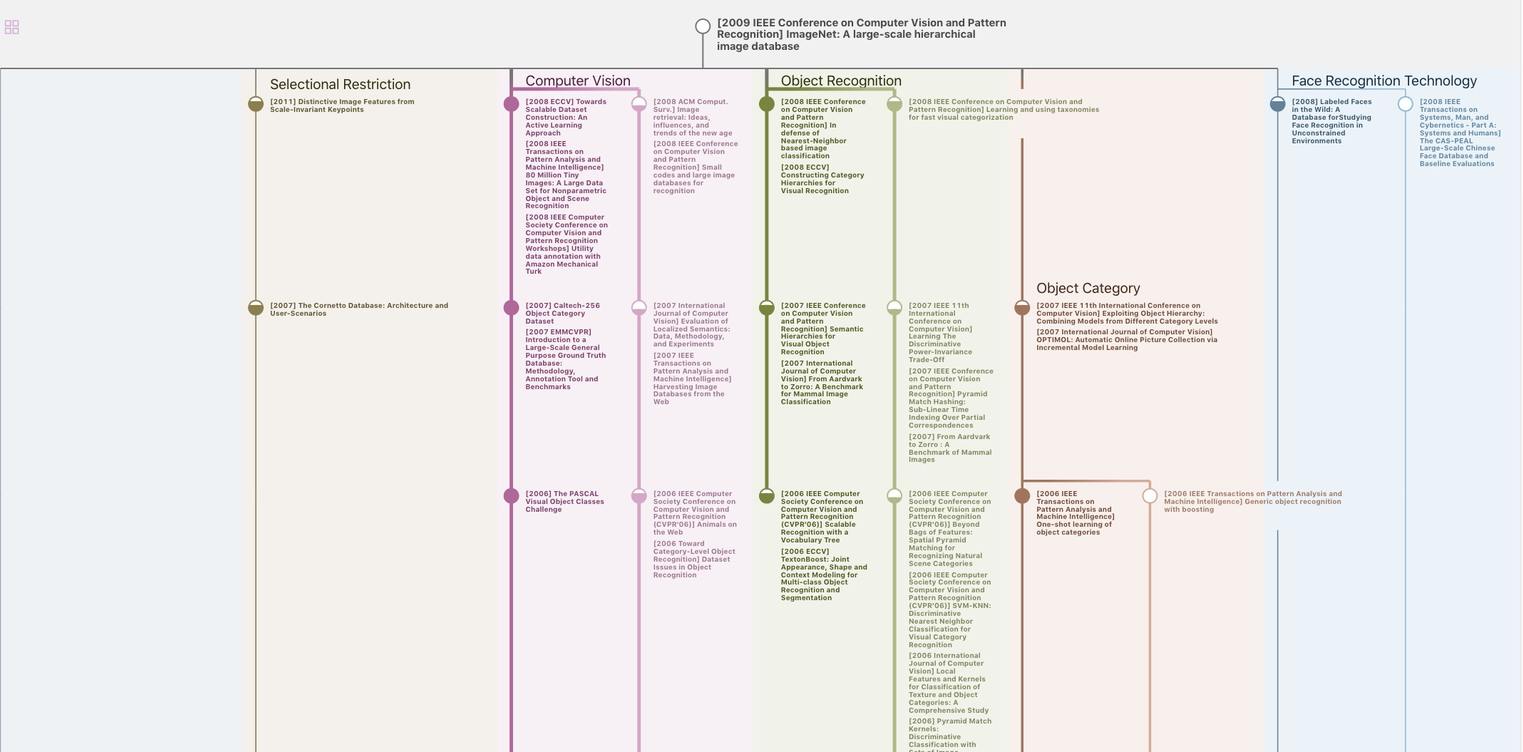
生成溯源树,研究论文发展脉络
Chat Paper
正在生成论文摘要