On Optimal Early Stopping: Over-informative versus Under-informative Parametrization
arxiv(2022)
摘要
Early stopping is a simple and widely used method to prevent over-training neural networks. We develop theoretical results to reveal the relationship between the optimal early stopping time and model dimension as well as sample size of the dataset for certain linear models. Our results demonstrate two very different behaviors when the model dimension exceeds the number of features versus the opposite scenario. While most previous works on linear models focus on the latter setting, we observe that the dimension of the model often exceeds the number of features arising from data in common deep learning tasks and propose a model to study this setting. We demonstrate experimentally that our theoretical results on optimal early stopping time corresponds to the training process of deep neural networks.
更多查看译文
关键词
early stopping,optimal,parametrization,over-informative,under-informative
AI 理解论文
溯源树
样例
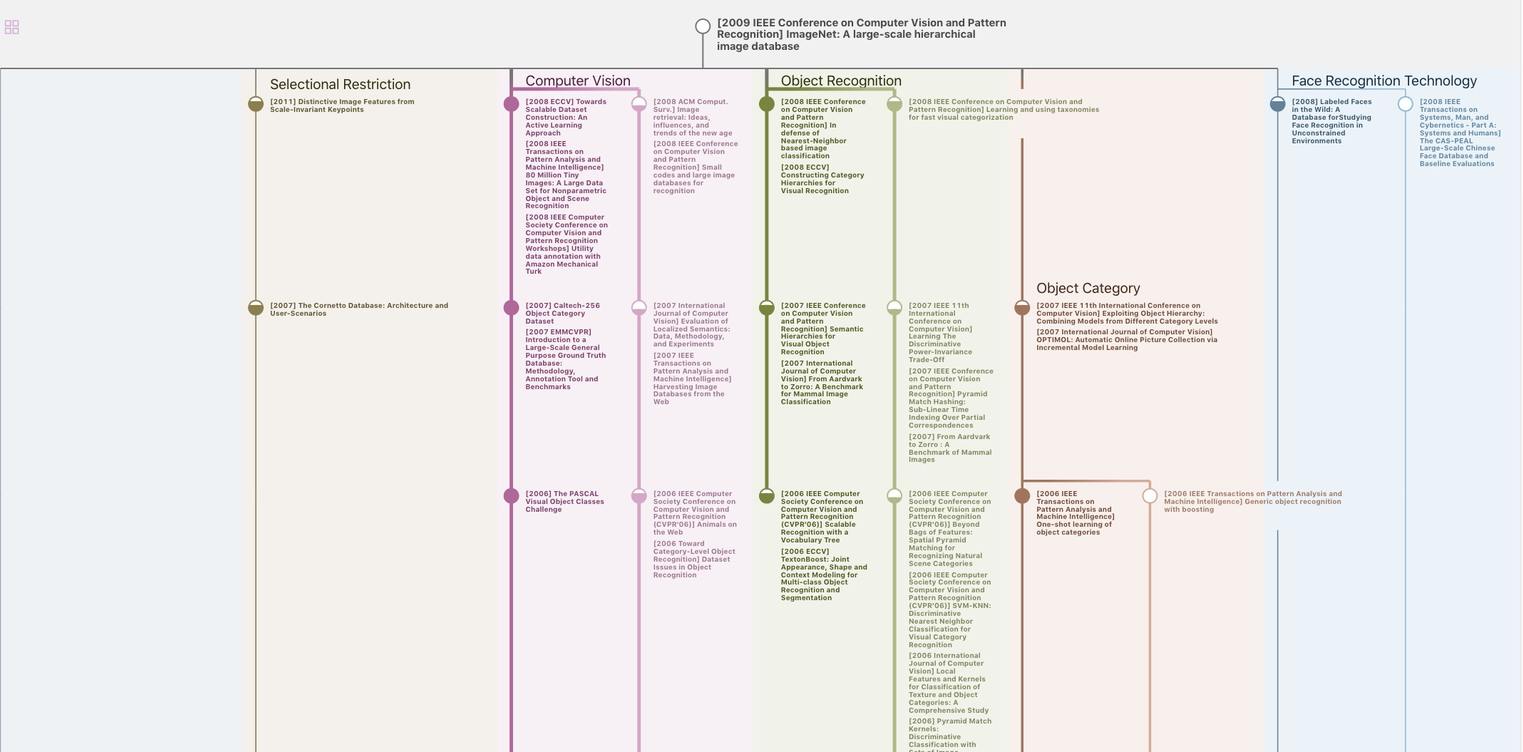
生成溯源树,研究论文发展脉络
Chat Paper
正在生成论文摘要