$\mathcal{Y}$-Tuning: An Efficient Tuning Paradigm for Large-Scale Pre-Trained Models via Label Representation Learning
CoRR(2022)
摘要
With the success of large-scale pre-trained models (PTMs), how efficiently adapting PTMs to downstream tasks has attracted tremendous attention, especially for PTMs with billions of parameters. Although some parameter-efficient tuning paradigms have been proposed to address this problem, they still require large resources to compute the gradients in the training phase. In this paper, we propose $\mathcal{Y}$-Tuning, an efficient yet effective paradigm to adapt frozen large-scale PTMs to specific downstream tasks. $\mathcal{Y}$-tuning learns dense representations for labels $\mathcal{Y}$ defined in a given task and aligns them to fixed feature representation. Without tuning the features of input text and model parameters, $\mathcal{Y}$-tuning is both parameter-efficient and training-efficient. For $\text{DeBERTa}_\text{XXL}$ with 1.6 billion parameters, $\mathcal{Y}$-tuning achieves performance more than $96\%$ of full fine-tuning on GLUE Benchmark with only $2\%$ tunable parameters and much fewer training costs.
更多查看译文
AI 理解论文
溯源树
样例
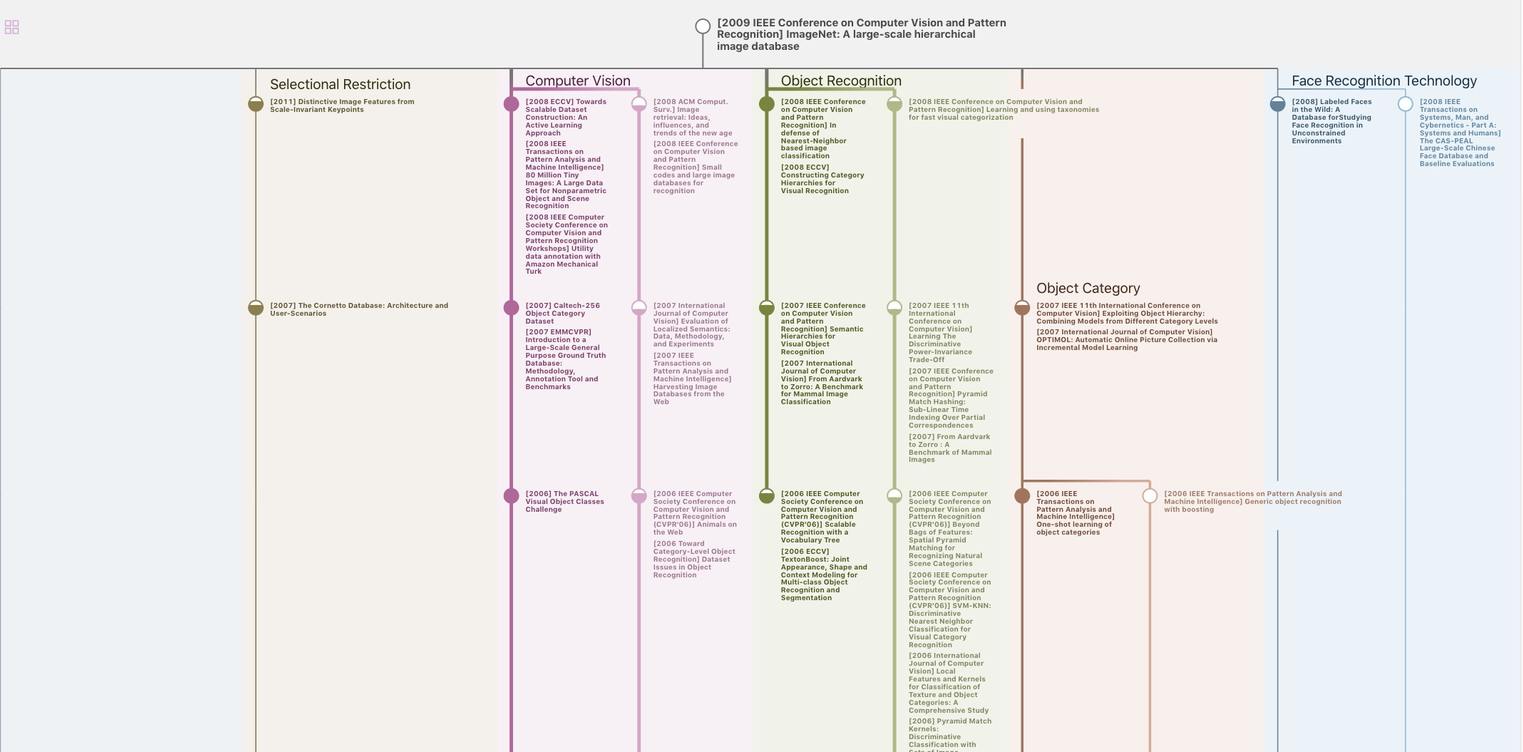
生成溯源树,研究论文发展脉络
Chat Paper
正在生成论文摘要