Predicting deformation mechanisms in architected metamaterials using GNN
arxiv(2022)
摘要
The present paradigm in design and modelling of lattice architected mechanical metamaterials is mostly limited to traditional numerical methods like finite element analysis. Recently, the use of machine learning and artificial intelligence techniques have become popular and here we extend these ideas to architected metamaterials. We show that truss based lattices have a natural resemblance to computational graphs which serve as an input for the rapidly emerging field of graph neural networks (GNNs). A dataset comprising thousands of such extremely complex lattices is trained using a GNN to predict the underlying dominant deformation mechanism viz. stretching and bending. The trained GNN achieves > 90% accuracy on a previously unseen complex lattice dataset. Such graph-based learning of metamaterials has the capability to predict a range of properties, from elastic moduli to fracture toughness and promises AI driven discovery of emergent metamaterials possessing superlative properties.
更多查看译文
关键词
metamaterials,deformation mechanisms,gnn
AI 理解论文
溯源树
样例
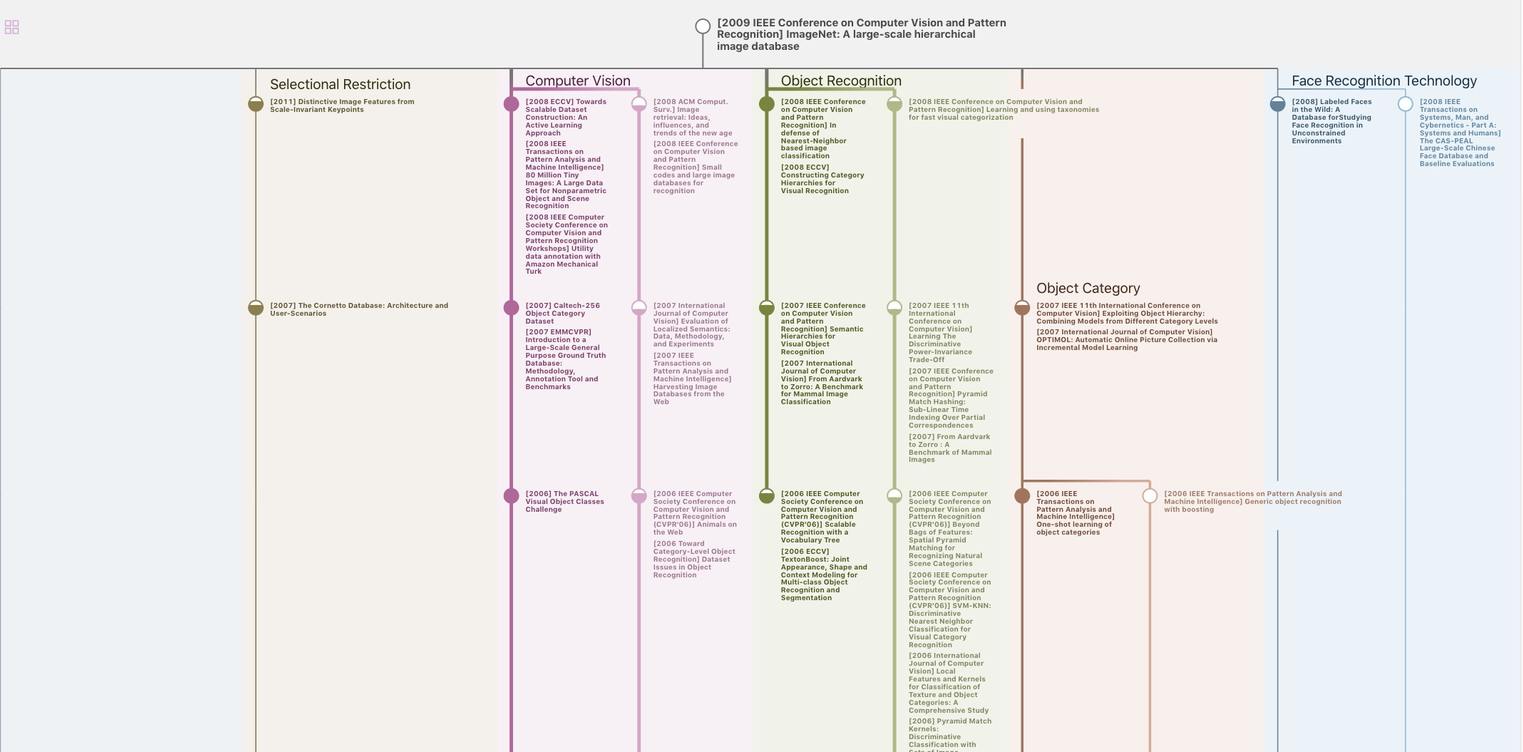
生成溯源树,研究论文发展脉络
Chat Paper
正在生成论文摘要