A two-phase rank-based algorithm for low-rank matrix completion
arxiv(2022)
摘要
Matrix completion aims to recover an unknown low-rank matrix from a small subset of its entries. In many applications, the rank of the unknown target matrix is known in advance and this information can be useful in the completion process. In this paper, first, we revisit a recently proposed rank-based heuristic for “known-rank” matrix completion and establish a condition under which the generated sequence is quasi-Fejér convergent to the solution set. Then, by including an acceleration mechanism similar to Nesterov’s acceleration, we obtain a new heuristic. Even though the convergence of this new heuristic cannot be granted in general, it turns out that it can be very useful as a warm-start phase (phase one), providing a suitable estimate for the regularization parameter and a good starting point to an accelerated proximal gradient algorithm (phase two) aimed to solve a nuclear-norm regularized problem. Numerical experiments with both synthetic and real data show that the resulting two-phase rank-based algorithm can recover low-rank matrices, with relatively high precision, faster than other well-established matrix completion algorithms.
更多查看译文
关键词
Matrix completion,Proximal gradient algorithm,Soft-thresholding
AI 理解论文
溯源树
样例
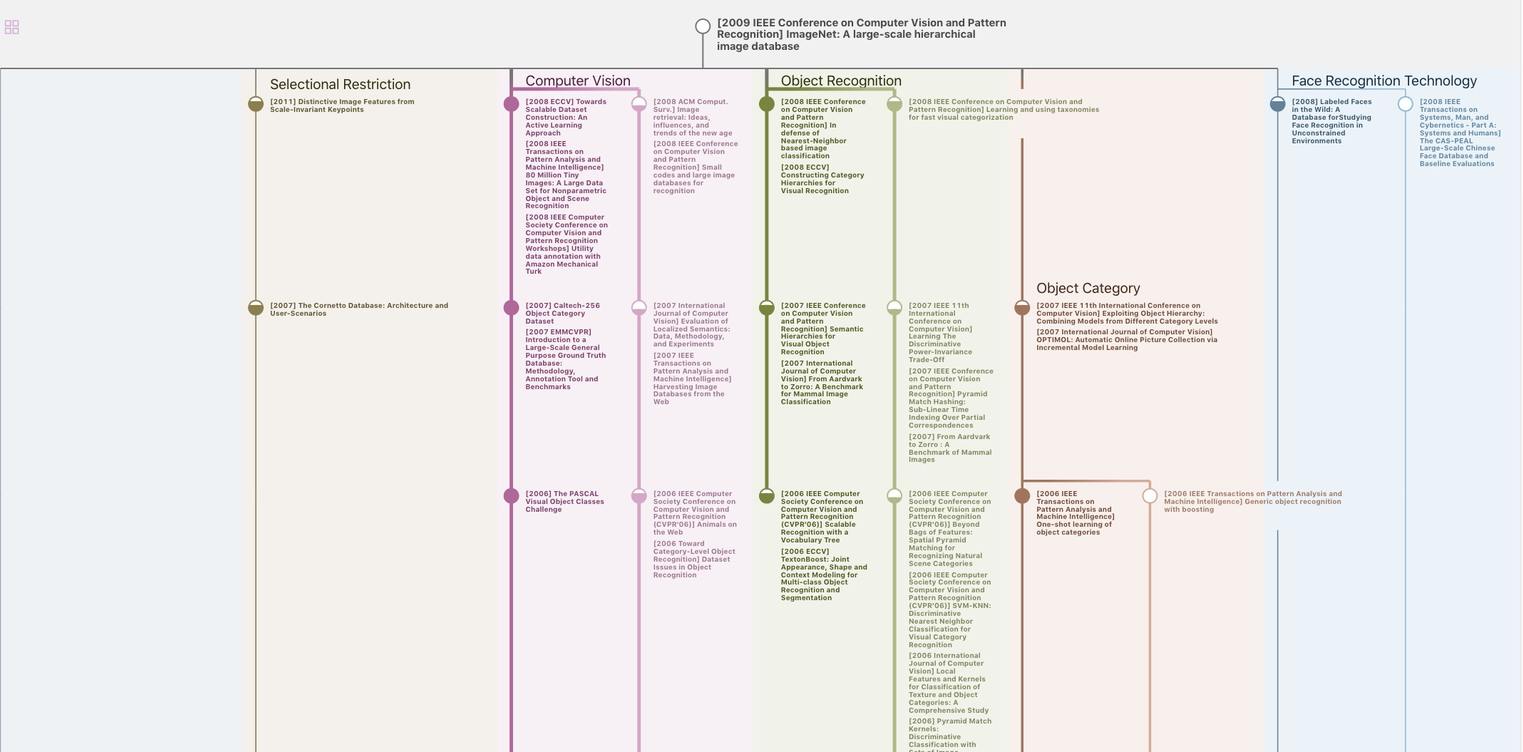
生成溯源树,研究论文发展脉络
Chat Paper
正在生成论文摘要